Virtual-Fixture Based Drilling Control for Robot-Assisted Craniotomy: Learning From Demonstration
IEEE ROBOTICS AND AUTOMATION LETTERS(2021)
摘要
One of the promising solutions for drilling craniotomy is robot-assisted surgery with human guidance. The present study deals with a piecewise collaborative drilling task assisted by a robot while containing aligning and drilling. It can enable surgeons to complete the operation more efficiently and accurately. The switched virtual fixture (VF) between drilling and aligning can be addressed using intention recognition, which learns from demon-strating human-guided force during the collaborative drilling. The training of the switching condition is derived in terms of Gaussian mixture models (GMMs) and the intention recognition is achieved using the Kullback-Leibler (KL) divergence between the GMMs and human-guided real-time sampled forces. To evaluate the performance of aligning and drilling, two experiments are conducted corresponding to the steps of drilling tasks. The compliance, accuracy, and costing time are demonstrated in the experiments. The results indicate that the proposed method has better performance (0.78 +/- 0.50 mm in collaborative drilling tasks for positioning accuracy and 38.65 +/- 5.00 s for time-consuming) than the conventional method(2.96 +/- 1.90 mm for positioning accuracy and 55.41 +/- 13.70 s for time-consuming).
更多查看译文
关键词
Physical human-robot interaction,robot-assisted craniotomy,dynamic virtual fixture,imitation Learning,gaussian mixture model
AI 理解论文
溯源树
样例
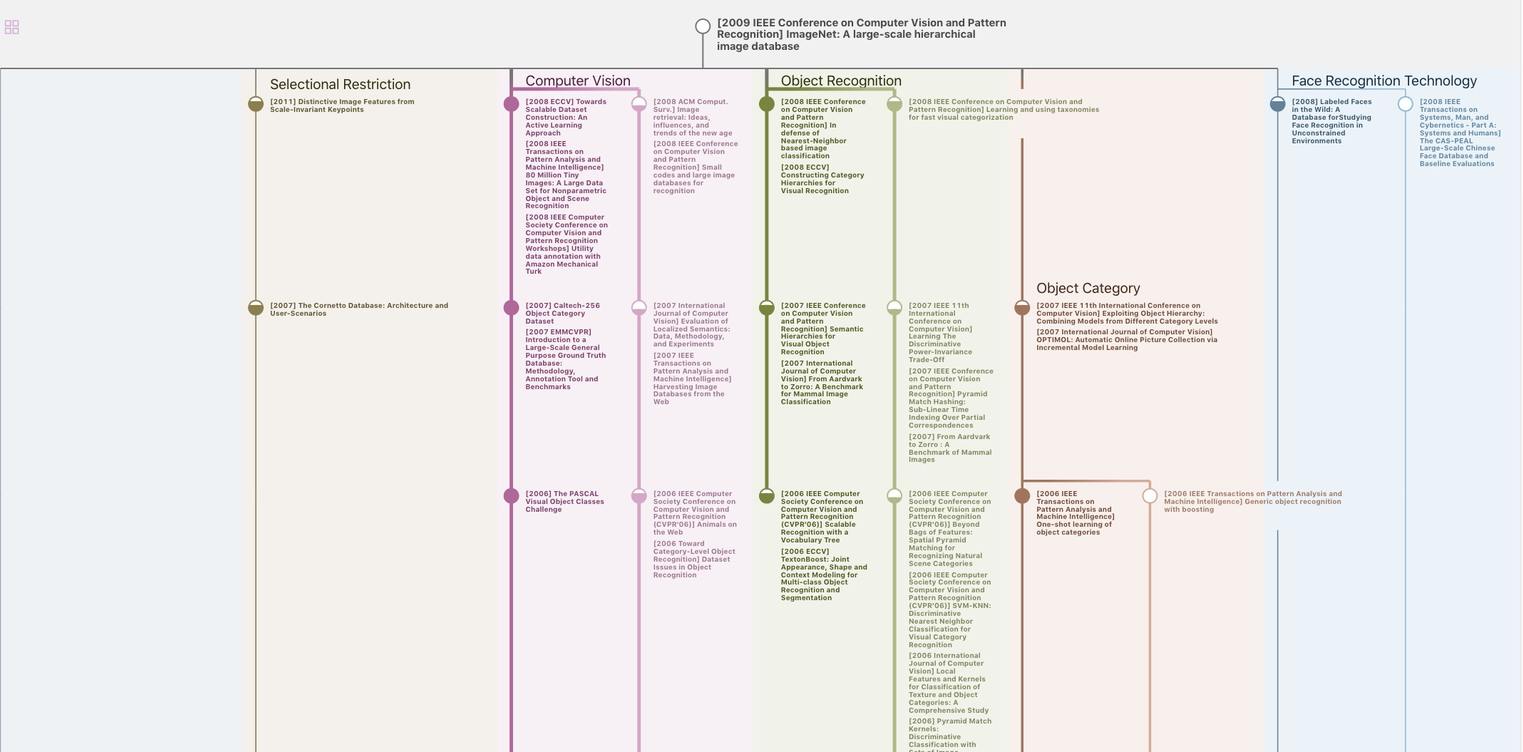
生成溯源树,研究论文发展脉络
Chat Paper
正在生成论文摘要