Robust Rank-Constrained Sparse Learning: A Graph-Based Framework for Single View and Multiview Clustering.
IEEE Transactions on Cybernetics(2021)
Abstract
Graph-based clustering aims to partition the data according to a similarity graph, which has shown impressive performance on various kinds of tasks. The quality of similarity graph largely determines the clustering results, but it is difficult to produce a high-quality one, especially when data contain noises and outliers. To solve this problem, we propose a robust rank constrained sparse learning (RRCSL) method in this article. The L2,1 -norm is adopted into the objective function of sparse representation to learn the optimal graph with robustness. To preserve the data structure, we construct an initial graph and search the graph within its neighborhood. By incorporating a rank constraint, the learned graph can be directly used as the cluster indicator, and the final results are obtained without additional postprocessing. In addition, the proposed method cannot only be applied to single-view clustering but also extended to multiview clustering. Plenty of experiments on synthetic and real-world datasets have demonstrated the superiority and robustness of the proposed framework.
MoreTranslated text
Key words
Optimization,Linear programming,Clustering algorithms,Laplace equations,Sparse matrices,Robustness,Data structures,Graph clustering,graph learning,machine learning,multiview clustering,sparse representation
AI Read Science
Must-Reading Tree
Example
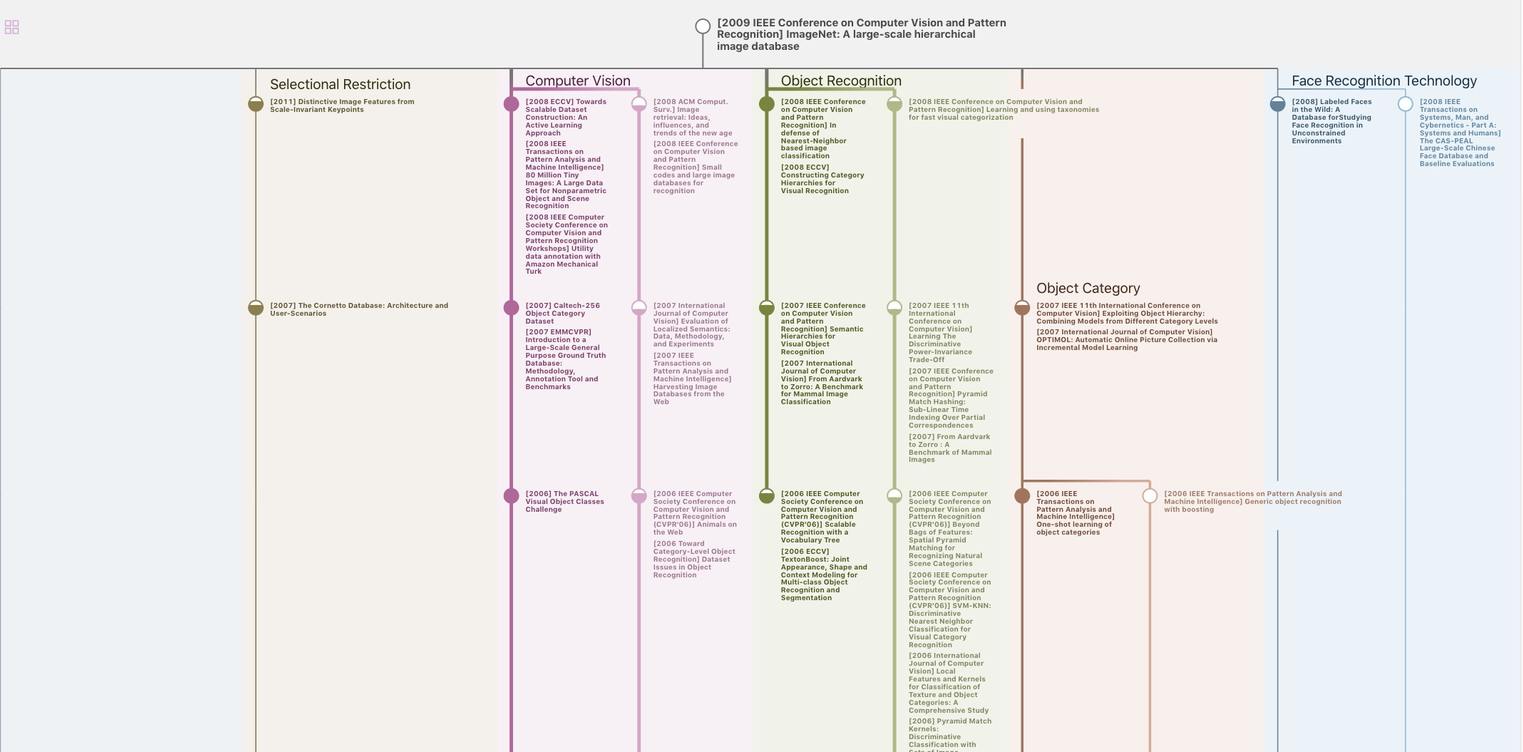
Generate MRT to find the research sequence of this paper
Chat Paper
Summary is being generated by the instructions you defined