Estimating the False Positive Prediction Rate in Automated Volumetric Measurements of Malignant Pleural Mesothelioma.
BIOSTEC(2020)
Abstract
Malignant Pleural Mesothelioma (MPM) is a rare cancer associated with exposure to asbestos fibres. It grows in the pleural space surrounding the lungs, exhibiting an irregular shape with high surface-to-volume ratio. Reliable measurements are important to assessing treatment efficacy, however these tumour characteristics make manual measurements time consuming, and prone to intra- and inter-observer variation. Previously we described a fully automatic Convolutional Neural Network (CNN) for volumetric measurement of MPM in CT images, trained and evaluated by seven-fold cross validation on 123 CT datasets with expert manual annotations. The mean difference between the manual and automatic volume measurements was not significantly different from zero (27.2 cm\(^3\); \(p = 0.225\)), the 95% limits of agreement were between −417 and +363 cm\(^3\), and the mean Dice coefficient was 0.64. Previous studies have focused on images with known MPM, sometimes even focusing on the lung with known MPM. In this paper, we investigate the false positive detection rate in a large image set with no known MPM. For this, a cohort of 14,965 subjects from the National Lung Screening Trial (NLST) were analysed. The mean volume of “MPM” found in these images by the automated detector was 3.6 cm\(^3\) (compared with 547.2 cm\(^3\) for MPM positive subjects). A qualitative examination of the one hundred subjects with the largest probable false detection volumes found that none of them were normal: the majority contain hyperdense pathology, large regions of pleural effusion, or evidence of pleural thickening. One false positive was caused by liver masses. The next step will be to evaluate the automated measurement accuracy on an independent, unseen, multi-centre data set.
MoreTranslated text
Key words
Malignant Pleural Mesothelioma (MPM),Deep learning (DL),Convolutional Neural Network (CNN),Computed tomography (CT),Image segmentation
AI Read Science
Must-Reading Tree
Example
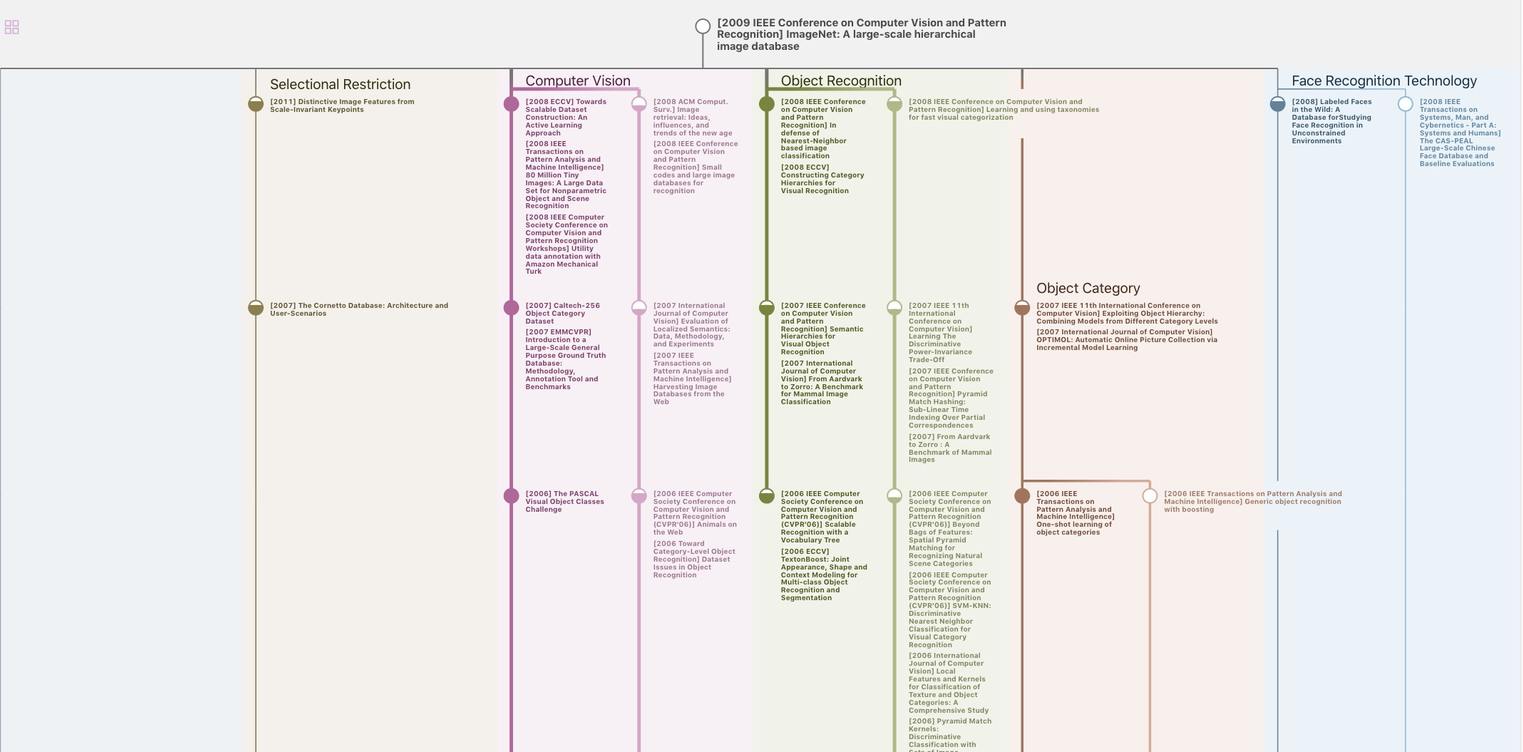
Generate MRT to find the research sequence of this paper
Chat Paper
Summary is being generated by the instructions you defined