BayesSpace enables the robust characterization of spatial gene expression architecture in tissue sections at increased resolution
user-5f8cf9244c775ec6fa691c99(2020)
摘要
Abstract Recently developed spatial gene expression technologies such as the Spatial Transcriptomics and Visium platforms allow for comprehensive measurement of transcriptomic profiles while retaining spatial context. However, existing methods for analyzing spatial gene expression data often do not efficiently leverage the spatial information and fail to address the limited resolution of the technology. Here, we introduce BayesSpace, a fully Bayesian statistical method for clustering analysis and resolution enhancement of spatial transcriptomics data that seamlessly integrates into current transcriptomics analysis workflows. We show that BayesSpace improves the identification of transcriptionally distinct tissues from spatial transcriptomics samples of the brain, of melanoma, and of squamous cell carcinoma. In particular, BayesSpace’s improved resolution allows the identification of tissue structure that is not detectable at the original resolution and thus not recovered by other methods. Using an in silico dataset constructed from scRNA-seq, we demonstrate that BayesSpace can spatially resolve expression patterns to near single-cell resolution without the need for external single-cell sequencing data. In all, our results illustrate the utility BayesSpace has in facilitating the discovery of biological insights from a variety of spatial transcriptomics datasets.
更多查看译文
关键词
Spatial analysis,Cluster analysis,Spatial contextual awareness,In silico,Computational biology,Bayesian probability,Gene expression,Computer science,Sequencing data,Tissue sections
AI 理解论文
溯源树
样例
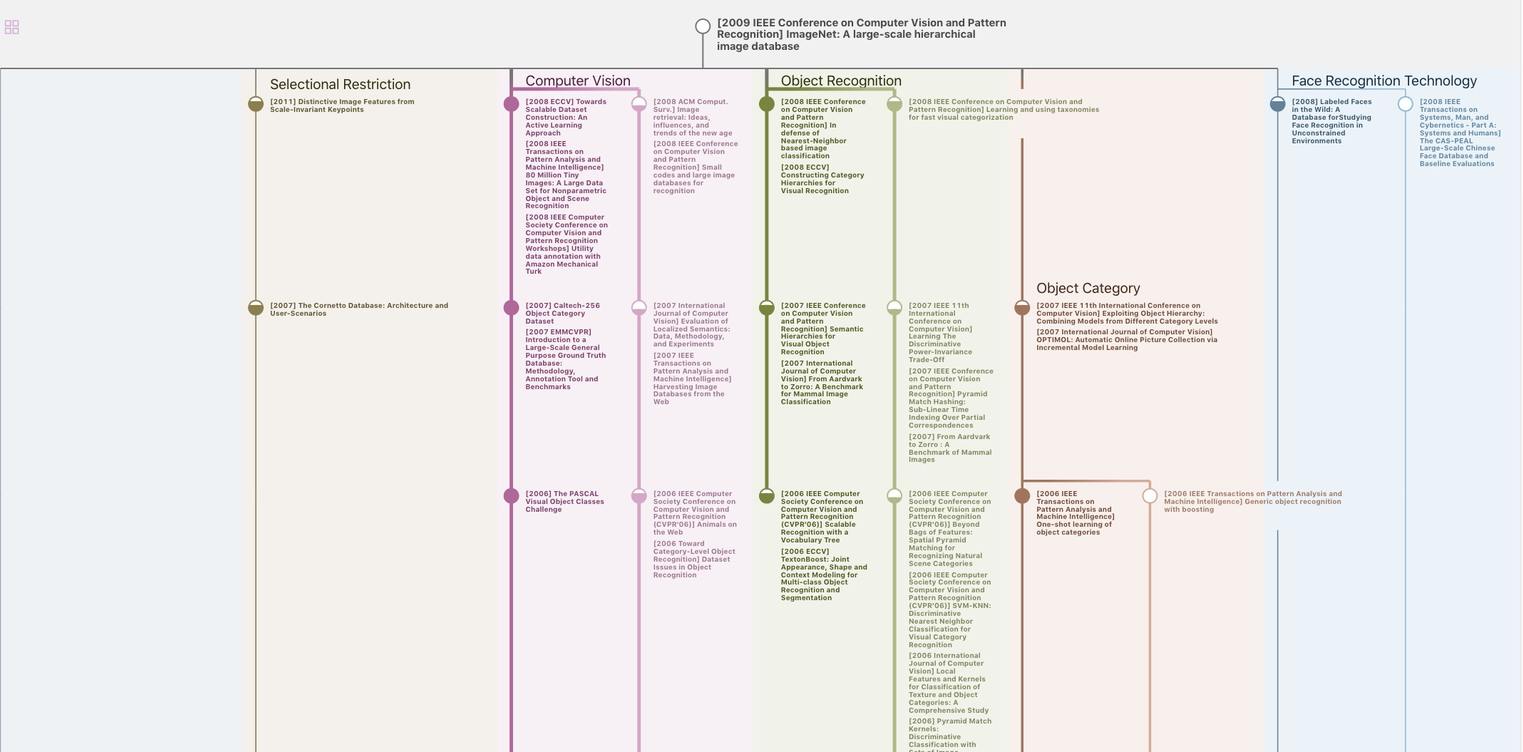
生成溯源树,研究论文发展脉络
Chat Paper
正在生成论文摘要