Active And Sparse Methods In Smoothed Model Checking
QUANTITATIVE EVALUATION OF SYSTEMS (QEST 2021)(2021)
摘要
Smoothed model checking based on Gaussian process classification provides a powerful approach for statistical model checking of parametric continuous time Markov chain models. The method constructs a model for the functional dependence of satisfaction probability on the Markov chain parameters. This is done via Gaussian process inference methods from a limited number of observations for different parameter combinations. In this work we incorporate sparse variational methods and active learning into the smoothed model checking setting. We use these methods to improve the scalability of smoothed model checking. In particular, we see that active learning-based ideas for iteratively querying the simulation model for observations can be used to steer the model-checking to more informative areas of the parameter space and thus improve sample efficiency. We demonstrate that online extensions of sparse variational Gaussian process inference algorithms provide a scalable method for implementing active learning approaches for smoothed model checking.
更多查看译文
关键词
sparse methods,model checking
AI 理解论文
溯源树
样例
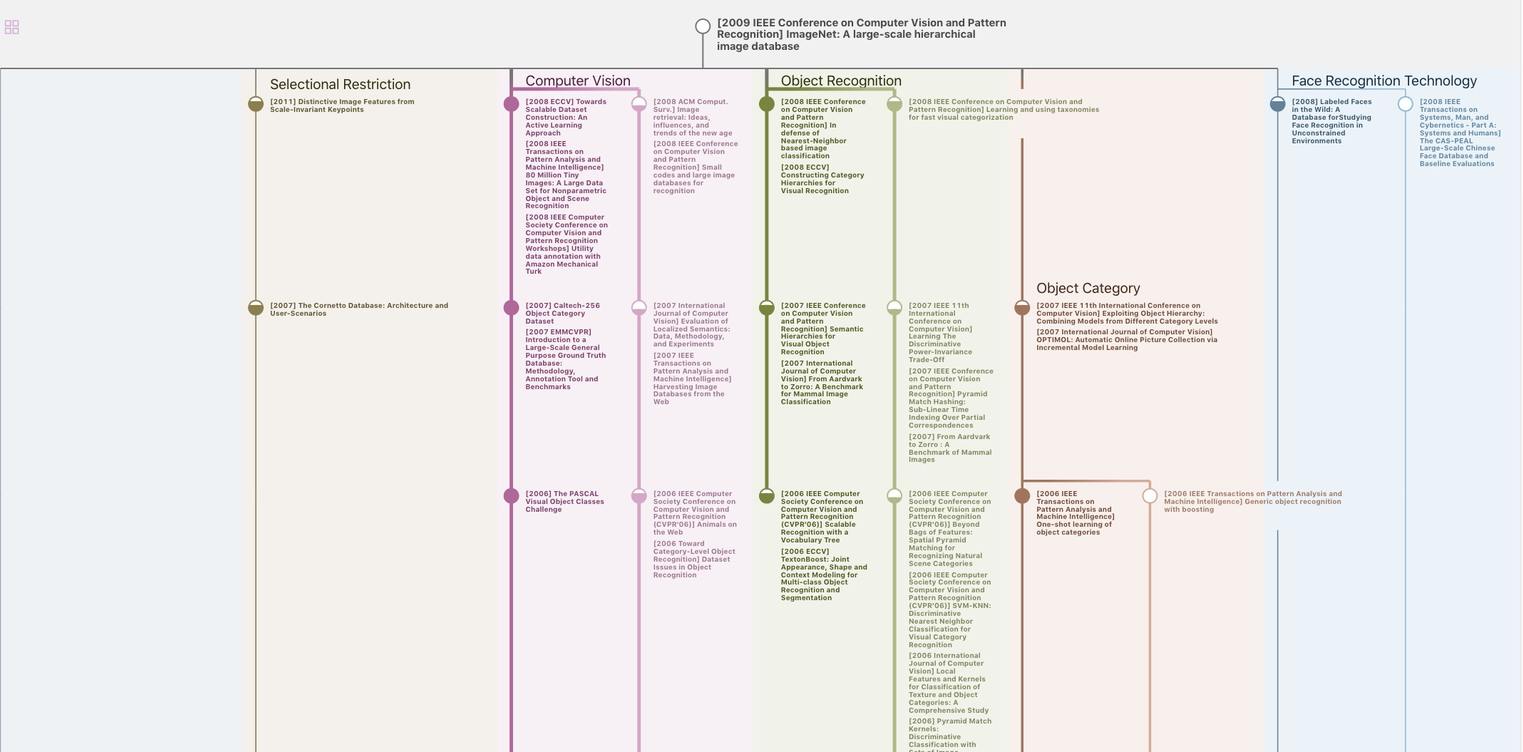
生成溯源树,研究论文发展脉络
Chat Paper
正在生成论文摘要