DeepHunter: A Graph Neural Network Based Approach for Robust Cyber Threat Hunting
SECURITY AND PRIVACY IN COMMUNICATION NETWORKS, SECURECOMM 2021, PT I(2021)
摘要
Cyber Threat hunting is a proactive search for known attack behaviors in the organizational information system. It is an important component to mitigate advanced persistent threats (APTs). However, the attack behaviors recorded in provenance data may not be completely consistent with the known attack behaviors. In this paper, we propose DeepHunter, a graph neural network (GNN) based graph pattern matching approach that can match provenance data against known attack behaviors in a robust way. Specifically, we design a graph neural network architecture with two novel networks: attribute embedding networks that could incorporate Indicators of Compromise (IOCs) information, and graph embedding networks that could capture the relationships between IOCs. To evaluate DeepHunter, we choose five real and synthetic APT attack scenarios. Results show that DeepHunter can hunt all attack behaviors, and the accuracy and robustness of DeepHunter outperform the state-of-the-art method, Poirot.
更多查看译文
关键词
Cyber threat hunting,Robustness,Provenance analysis,Graph neural network,Graph pattern matching
AI 理解论文
溯源树
样例
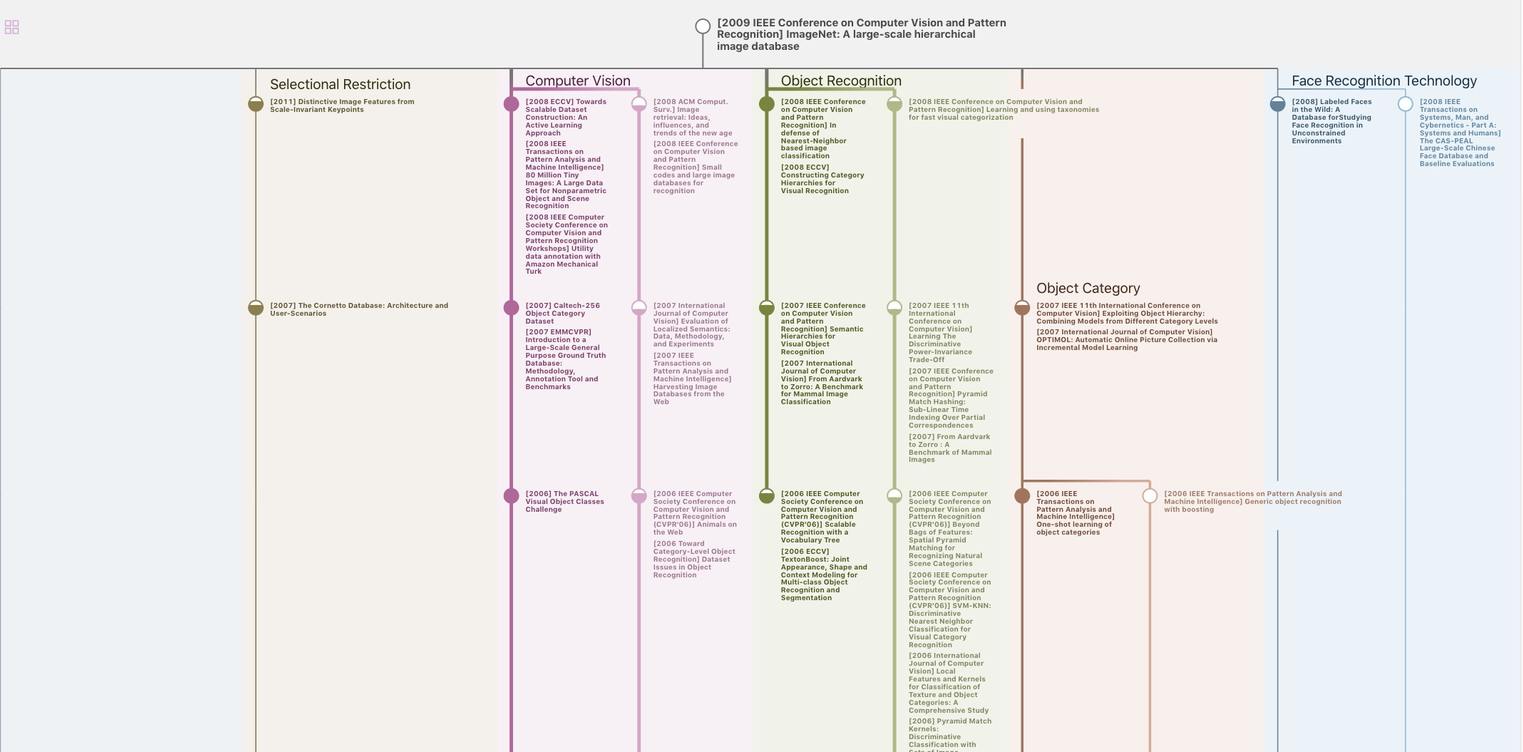
生成溯源树,研究论文发展脉络
Chat Paper
正在生成论文摘要