X-METRA-ADA: Cross-lingual Meta-Transfer Learning Adaptation to Natural Language Understanding and Question Answering
NAACL-HLT(2021)
摘要
Multilingual models, such as M-BERT and XLM-R, have gained increasing popularity, due to their zero-shot cross-lingual transfer learning capabilities. However, their generalization ability is still inconsistent for typologically diverse languages and across different benchmarks. Recently, meta-learning has garnered attention as a promising technique for enhancing transfer learning under low-resource scenarios: particularly for cross-lingual transfer in Natural Language Understanding (NLU). In this work, we propose X-METRA-ADA, a cross-lingual MEta-TRAnsfer learning ADAptation approach for NLU. Our approach adapts MAML, an optimization-based meta-learning approach, to learn to adapt to new languages. We extensively evaluate our framework on two challenging cross-lingual NLU tasks: multilingual task-oriented dialog and typologically diverse question answering. We show that our approach outperforms naive fine-tuning, reaching competitive performance on both tasks for most languages. Our analysis reveals that X-METRA-ADA can leverage limited data for faster adaptation.
更多查看译文
关键词
adaptation,natural language understanding,learning,x-metra-ada,cross-lingual,meta-transfer
AI 理解论文
溯源树
样例
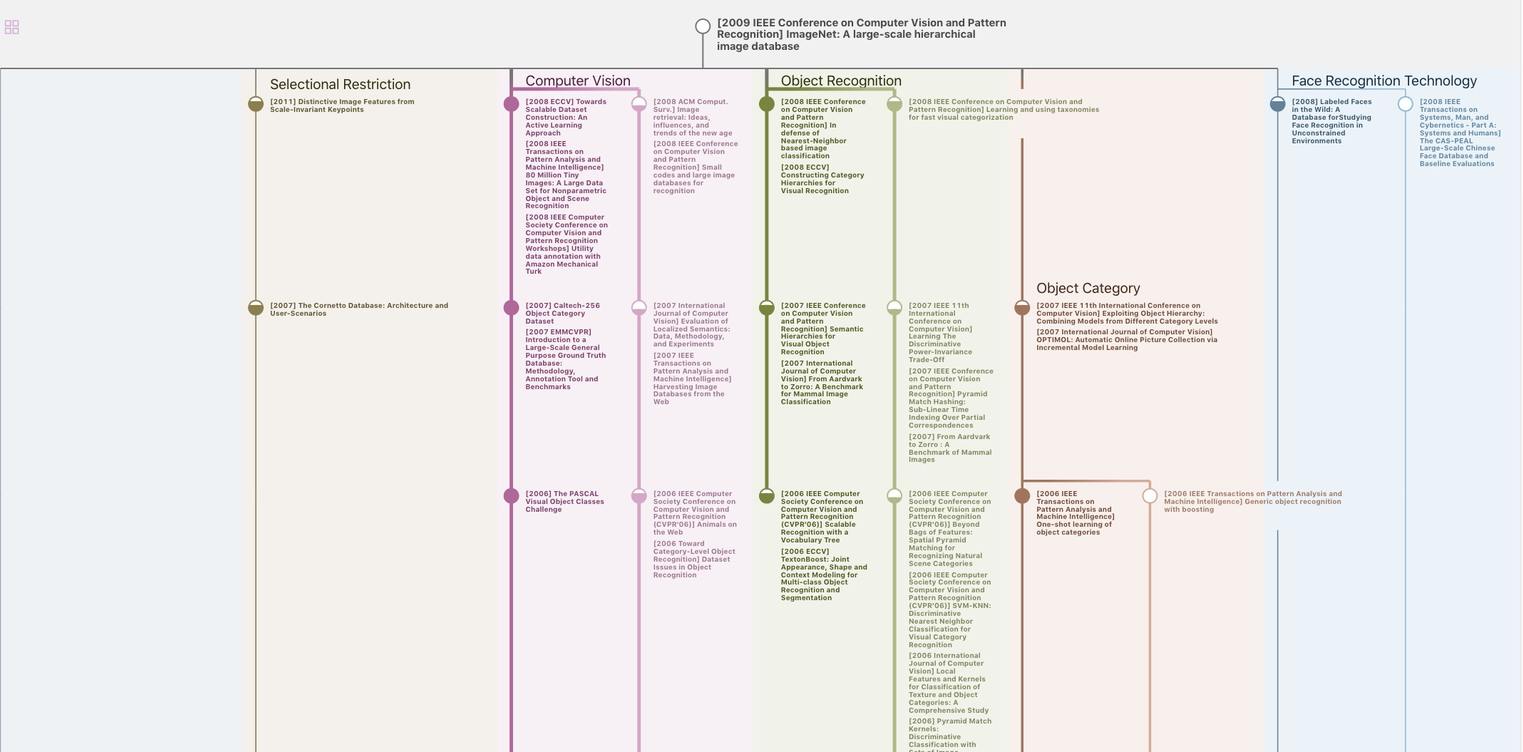
生成溯源树,研究论文发展脉络
Chat Paper
正在生成论文摘要