A Framework Using Contrastive Learning For Classification With Noisy Labels
DATA(2021)
摘要
We propose a framework using contrastive learning as a pre-training task to perform image classification in the presence of noisy labels. Recent strategies, such as pseudo-labeling, sample selection with Gaussian Mixture models, and weighted supervised contrastive learning have, been combined into a fine-tuning phase following the pre-training. In this paper, we provide an extensive empirical study showing that a preliminary contrastive learning step brings a significant gain in performance when using different loss functions: non robust, robust, and early-learning regularized. Our experiments performed on standard benchmarks and real-world datasets demonstrate that: (i) the contrastive pre-training increases the robustness of any loss function to noisy labels and (ii) the additional fine-tuning phase can further improve accuracy, but at the cost of additional complexity.
更多查看译文
关键词
noisy labels, image classification, contrastive learning, robust loss
AI 理解论文
溯源树
样例
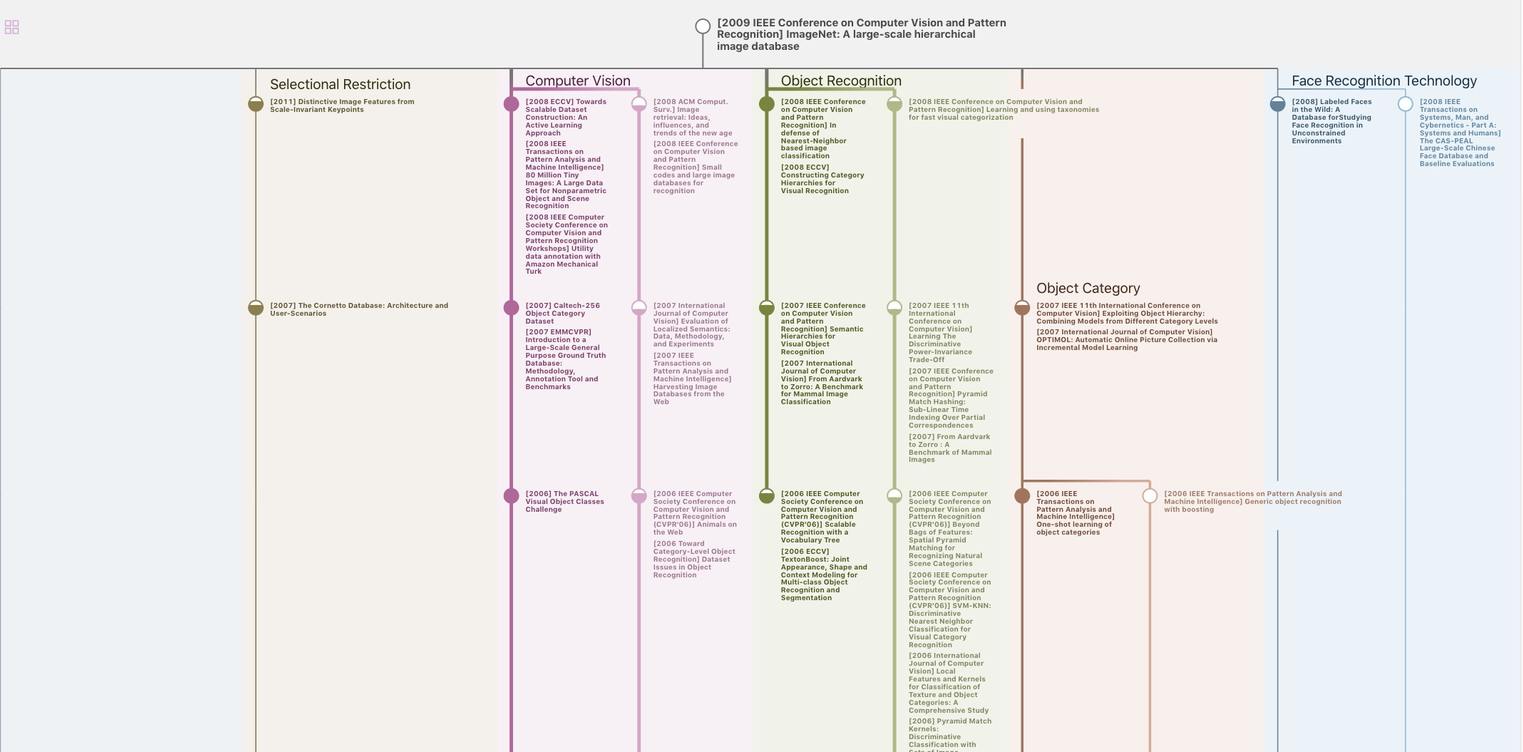
生成溯源树,研究论文发展脉络
Chat Paper
正在生成论文摘要