SuperMeshing: A New Deep Learning Architecture for Increasing the Mesh Density of Metal Forming Stress Field with Attention Mechanism and Perceptual Features
CoRR(2021)
摘要
In stress field analysis, the finite element analysis is a crucial approach, in which the mesh-density has a significant impact on the results. High mesh density usually contributes authentic to simulation results but costs more computing resources, leading to curtailing efficiency during the design process. To eliminate this drawback, we propose a new data-driven mesh-density boost model named SuperMeshingNet that strengthens the advantages of finite element analysis (FEA) with low mesh-density as inputs to the deep learning model, which consisting of Res-UNet architecture, to acquire high-density stress field instantaneously, shortening computing time and cost automatically. Moreover, the attention mechanism and the perceptual features are utilized, enhancing the performance of SuperMeshingNet. Compared to the baseline that applied the linear interpolation method, SuperMeshingNet achieves a prominent reduction in the mean squared error (MSE) and mean absolute error (MAE) on test data, which contains prior unseen cases. Based on the data set of metal forming, the comparable experiments are proceeded to demonstrate the high quality and superior precision of the reconstructed results generated by our model. The well-trained model can successfully show more excellent performance than the baseline and other methods on the multiple scaled mesh-density, including $2\times$, $4\times$, and $8\times$. With the refined result owning broaden scaling of mesh density and high precision, the FEA process can be accelerated with seldom cost on computation resources. We publicly share our work with full detail of implementation at https://github.com/zhenguonie/2021_SuperMeshing_2D_Metal_Forming
更多查看译文
关键词
metal forming stress field,new deep learning architecture,deep learning,supermeshing
AI 理解论文
溯源树
样例
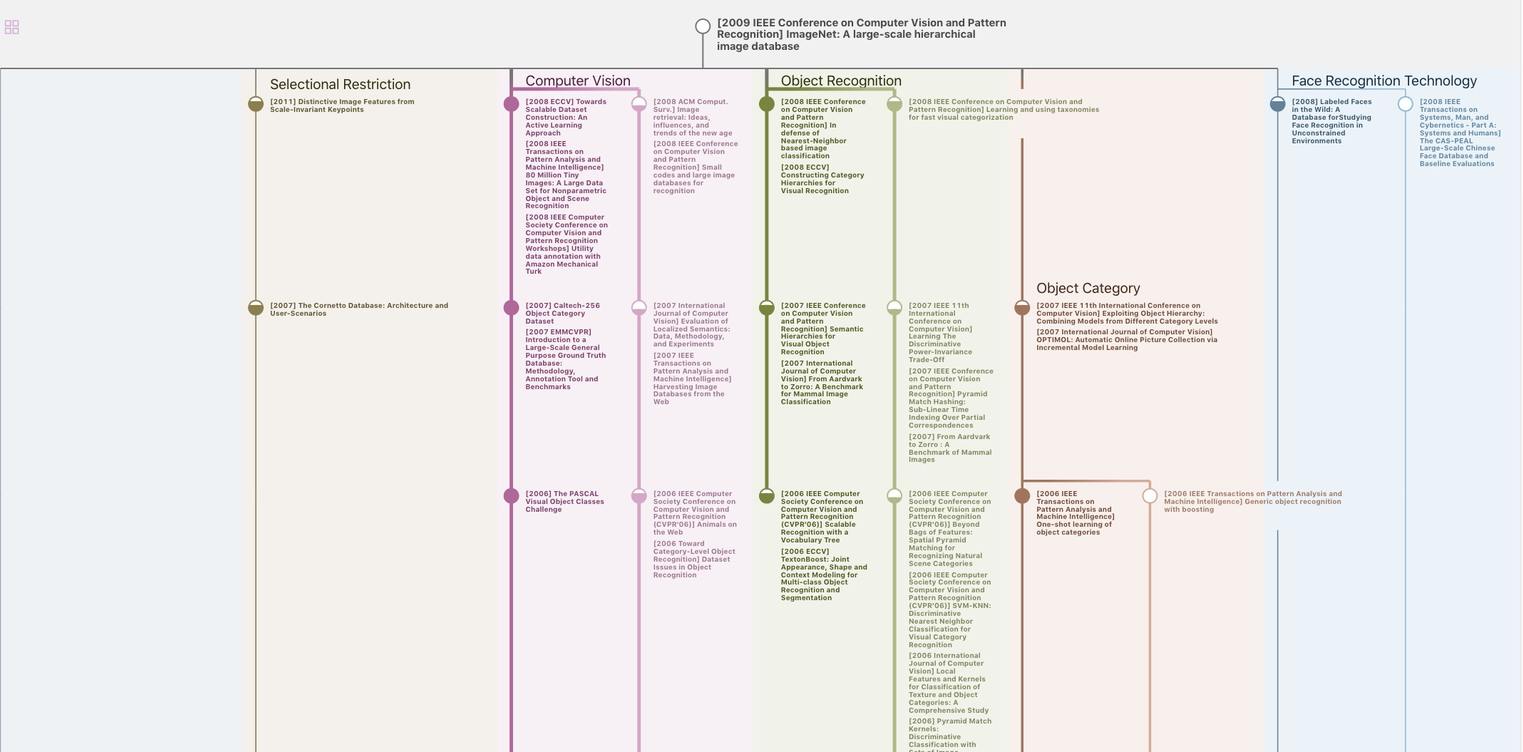
生成溯源树,研究论文发展脉络
Chat Paper
正在生成论文摘要