Quantum algorithms for SVD-based data representation and analysis
Quantum Machine Intelligence(2022)
摘要
This paper narrows the gap between previous literature on quantum linear algebra and practical data analysis on a quantum computer, formalizing quantum procedures that speed-up the solution of eigenproblems for data representations in machine learning. The power and practical use of these subroutines is shown through new quantum algorithms, sublinear in the input matrix’s size, for principal component analysis, correspondence analysis, and latent semantic analysis. We provide a theoretical analysis of the run-time and prove tight bounds on the randomized algorithms’ error. We run experiments on multiple datasets, simulating PCA’s dimensionality reduction for image classification with the novel routines. The results show that the run-time parameters that do not depend on the input’s size are reasonable and that the error on the computed model is small, allowing for competitive classification performances.
更多查看译文
关键词
Quantum computing, machine learning, Data analysis, Data representations, Singular value decomposition, Principal component analysis, Correspondence analysis, Latent semantic analysis
AI 理解论文
溯源树
样例
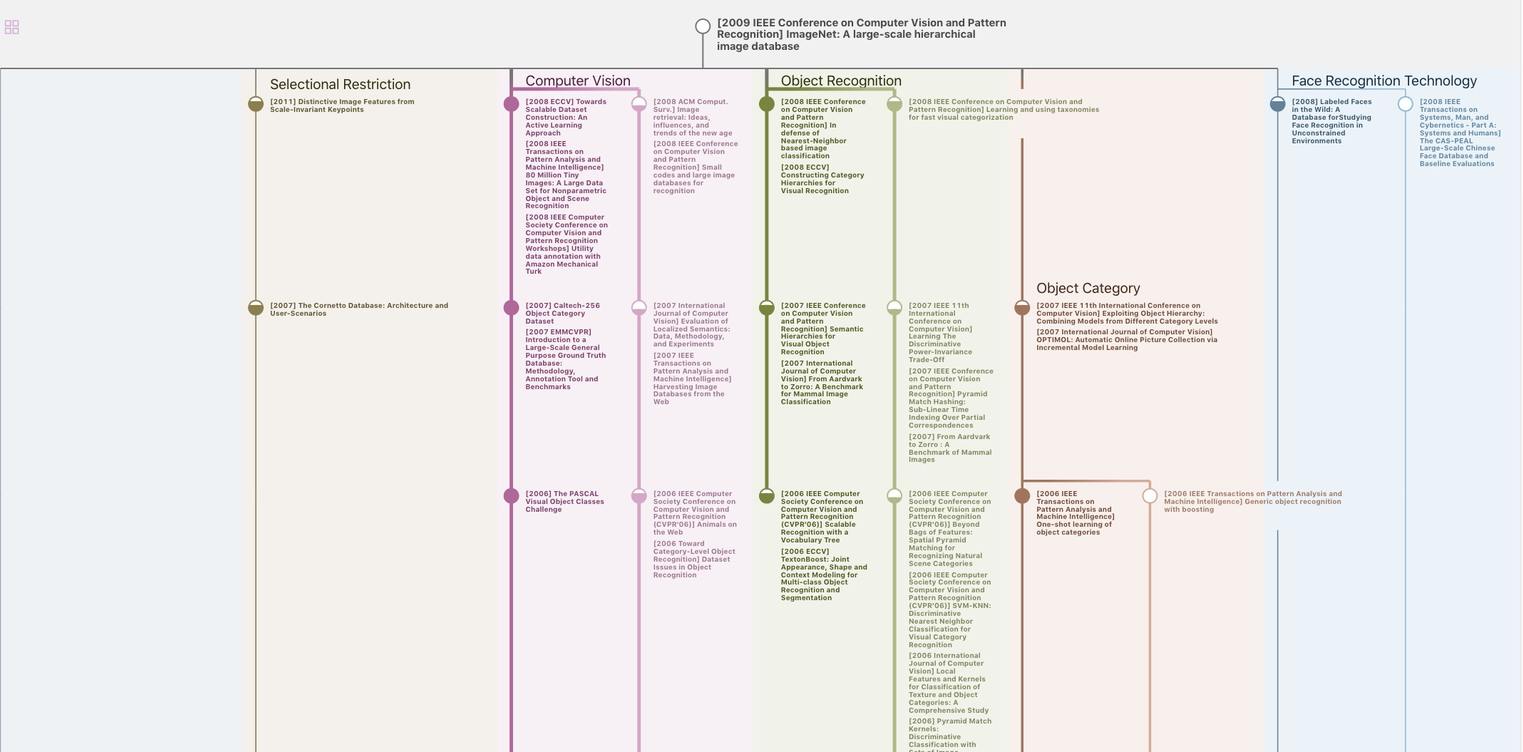
生成溯源树,研究论文发展脉络
Chat Paper
正在生成论文摘要