Emergence of Lie Symmetries in Functional Architectures Learned by CNNs
FRONTIERS IN COMPUTATIONAL NEUROSCIENCE(2021)
摘要
In this paper we study the spontaneous development of symmetries in the early layers of a Convolutional Neural Network (CNN) during learning on natural images. Our architecture is built in such a way to mimic some properties of the early stages of biological visual systems. In particular, it contains a pre-filtering step l(0) defined in analogy with the Lateral Geniculate Nucleus (LGN). Moreover, the first convolutional layer is equipped with lateral connections defined as a propagation driven by a learned connectivity kernel, in analogy with the horizontal connectivity of the primary visual cortex (V1). We first show that the l(0) filter evolves during the training to reach a radially symmetric pattern well approximated by a Laplacian of Gaussian (LoG), which is a well-known model of the receptive profiles of LGN cells. In line with previous works on CNNs, the learned convolutional filters in the first layer can be approximated by Gabor functions, in agreement with well-established models for the receptive profiles of V1 simple cells. Here, we focus on the geometric properties of the learned lateral connectivity kernel of this layer, showing the emergence of orientation selectivity w.r.t. the tuning of the learned filters. We also examine the short-range connectivity and association fields induced by this connectivity kernel, and show qualitative and quantitative comparisons with known group-based models of V1 horizontal connections. These geometric properties arise spontaneously during the training of the CNN architecture, analogously to the emergence of symmetries in visual systems thanks to brain plasticity driven by external stimuli.
更多查看译文
关键词
lie symmetries, CNN-convolutional neural network, primary visual cortex (V1), lateral connection, lateral geniculate, sub-Riemannian geometries
AI 理解论文
溯源树
样例
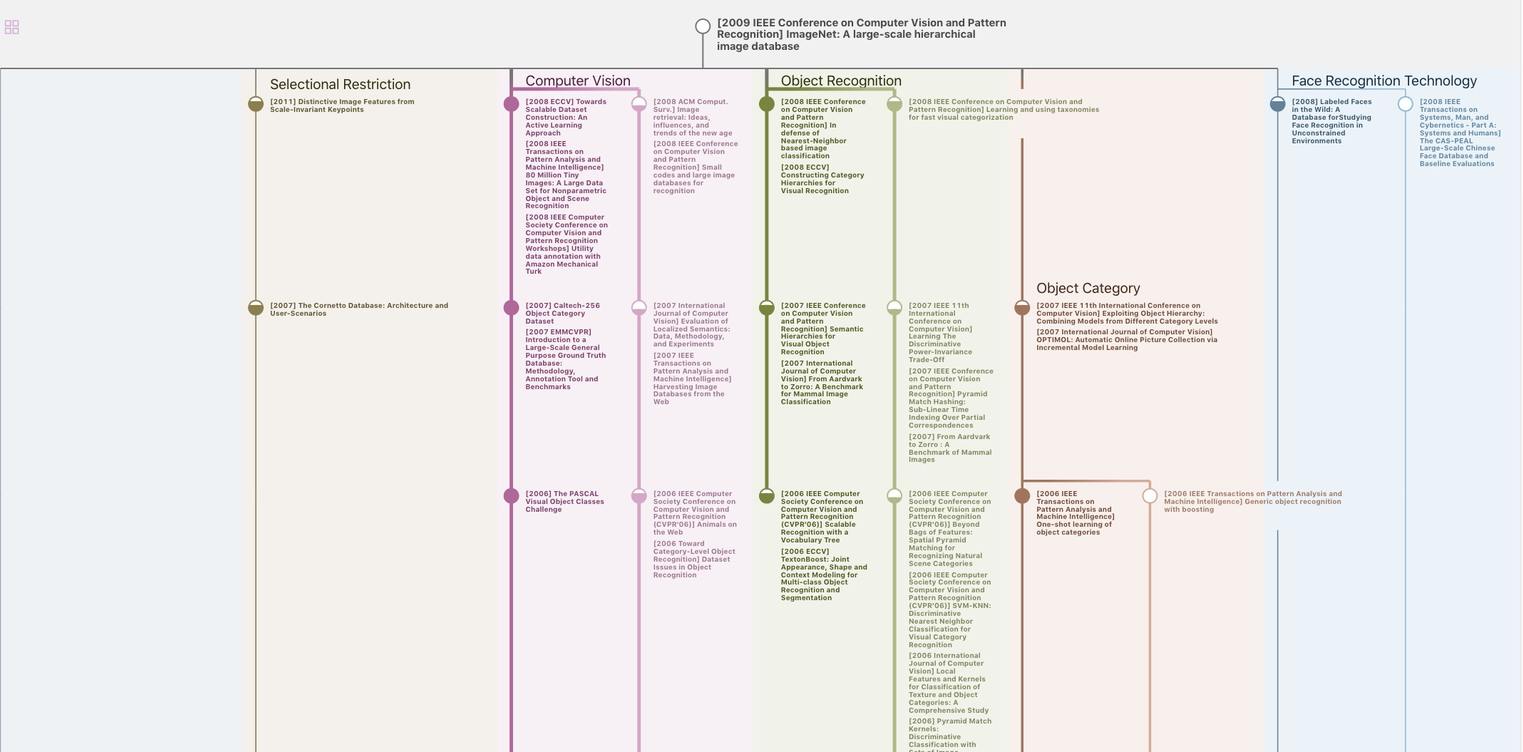
生成溯源树,研究论文发展脉络
Chat Paper
正在生成论文摘要