Two to Trust - AutoML for Safe Modelling and Interpretable Deep Learning for Robustness.
TAILOR(2020)
摘要
With great power comes great responsibility . The success of machine learning, especially deep learning, in research and practice has attracted a great deal of interest, which in turn necessitates increased trust. Sources of mistrust include matters of model genesis (“Is this really the appropriate model?”) and interpretability (“Why did the model come to this conclusion?”, “Is the model safe from being easily fooled by adversaries?”). In this paper, two partners for the trustworthiness tango are presented: recent advances and ideas, as well as practical applications in industry in (a) Automated machine learning (AutoML), a powerful tool to optimize deep neural network architectures and fine-tune hyperparameters, which promises to build models in a safer and more comprehensive way; (b) Interpretability of neural network outputs, which addresses the vital question regarding the reasoning behind model predictions and provides insights to improve robustness against adversarial attacks.
更多查看译文
关键词
safe modelling,interpretable deep learning,robustness,automl
AI 理解论文
溯源树
样例
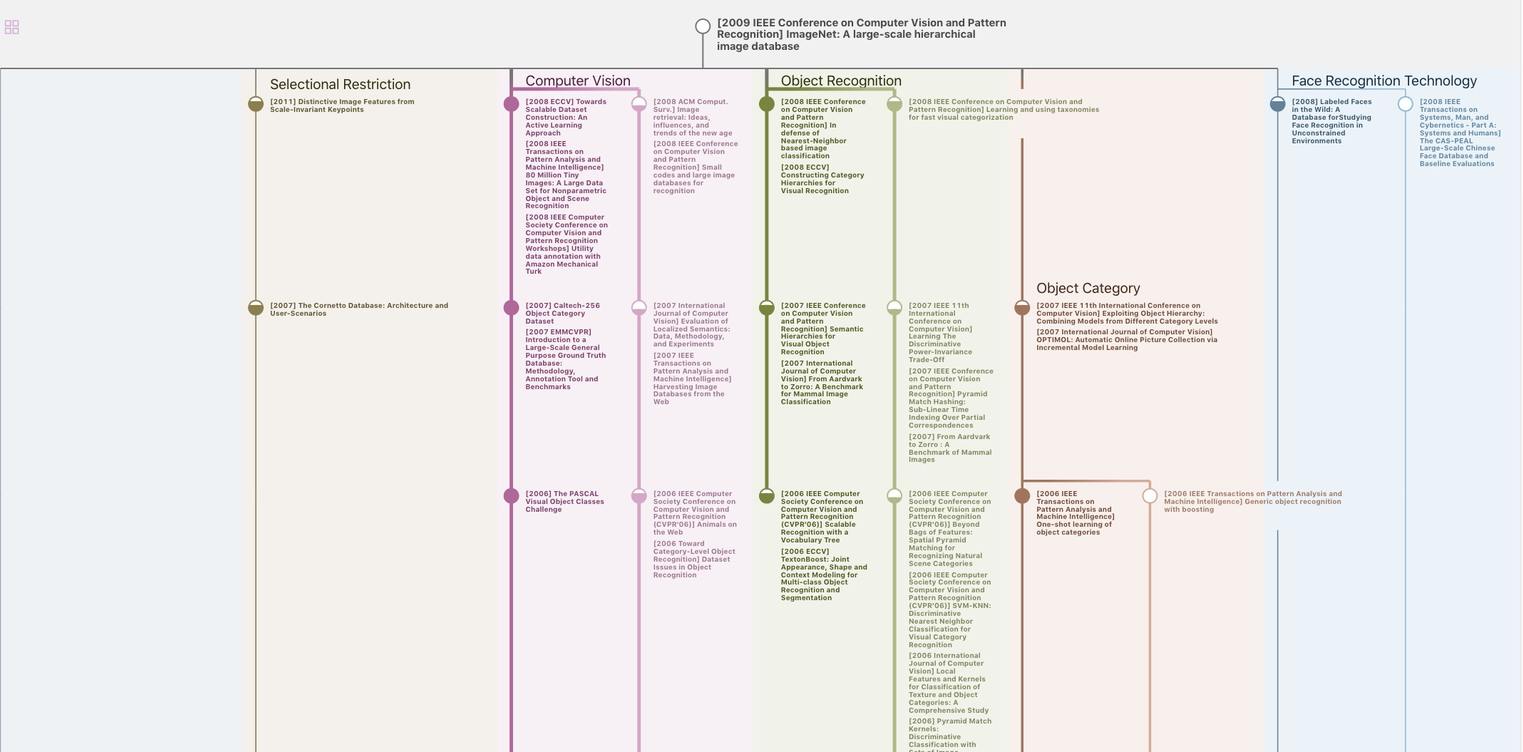
生成溯源树,研究论文发展脉络
Chat Paper
正在生成论文摘要