BERT2Code: Can Pretrained Language Models be Leveraged for Code Search?
arxiv(2021)
摘要
Millions of repetitive code snippets are submitted to code repositories every day. To search from these large codebases using simple natural language queries would allow programmers to ideate, prototype, and develop easier and faster. Although the existing methods have shown good performance in searching codes when the natural language description contains keywords from the code, they are still far behind in searching codes based on the semantic meaning of the natural language query and semantic structure of the code. In recent years, both natural language and programming language research communities have created techniques to embed them in vector spaces. In this work, we leverage the efficacy of these embedding models using a simple, lightweight 2-layer neural network in the task of semantic code search. We show that our model learns the inherent relationship between the embedding spaces and further probes into the scope of improvement by empirically analyzing the embedding methods. In this analysis, we show that the quality of the code embedding model is the bottleneck for our model's performance, and discuss future directions of study in this area.
更多查看译文
关键词
pretrained language models,bert2code search
AI 理解论文
溯源树
样例
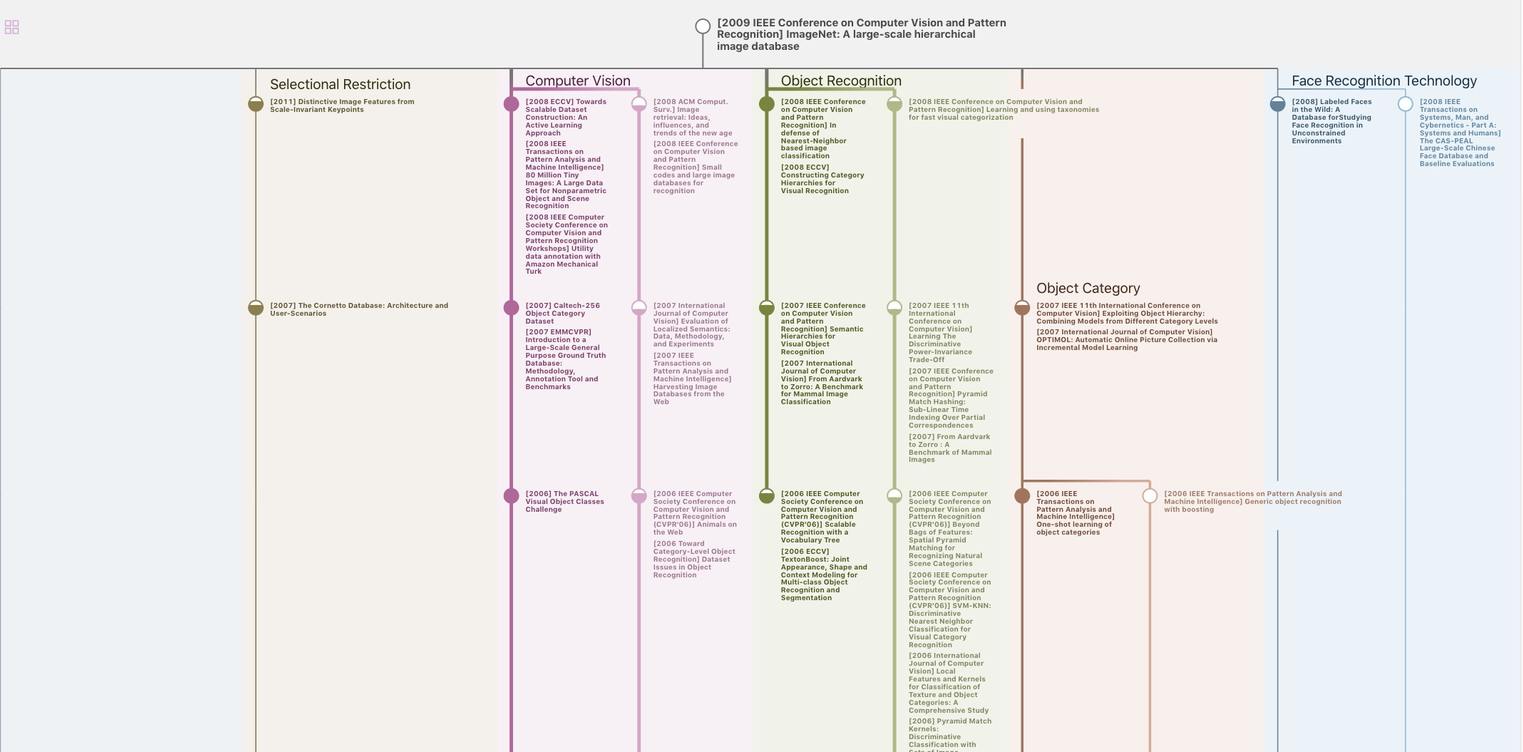
生成溯源树,研究论文发展脉络
Chat Paper
正在生成论文摘要