Evaluation of 'Deep Learning System' (DLS) for Assistive Diagnosis of Brain Tumors Based on MR Image
SSRN Electronic Journal(2019)
Abstract
Background: To improve the effectiveness of brain tumor screening, decrease misdiagnosis, omitted diagnosis, and to increase the work efficiency of neuroradiologists, a Deep Learning System (DLS), which could assist doctors in achieving rapid, accurate, brain tumor lesion interpretation, provide more detailed lesion information, and improve the accuracy of the diagnoses of intracranial brain tumors.
Methods: In particular, we developed a deep learning system to detect, diagnose, and localize brain tumors in MRI images, based on convolutional neural network (CNN), able of achieving a level of diagnostic accuracy comparable to the average of accuracy levels of senior neuroradiologists. More specifically, in two test sets, we evaluate DLS ability to diagnose brain tumors (Test One) and to help doctors to improve their diagnostic accuracy (Test Two).
Findings: In Test One, the DLS had a higher top-1 accuracy compared to the average of all of the evaluators who are young doctors within 10 years' clinical experience (67.0% vs. 62.2%), and correspondingly a significantly better top-2 accuracy (79.7% vs. 69.1%) as well. In Test Set Two, the accuracy of evaluators without DLS was 63.5%, and more importantly, the accuracy of evaluators with DLS assistance was 76.1%, totally the accuracy in Top-2 has a 12.6% increase. More in detail, the DLS could correct the evaluator more than 75.8% wrong diagnosis.
Interpretation: This significant improvement in diagnostic accuracy proved that DLS could be a useful auxiliary tool for neuroradiologists to improve their prediction accuracy. Further research is necessary to assess the feasibility of applying DLS in a clinical setting.
Funding Statement: The study is Supported by National Key R&D Program of China, (#2018YFC1312903, #2017YFC1310901), funded by China National Clinical Research Centre.
Declaration of Interests: All authors declare no competing interests.
Ethics Approval Statement: The present study was approved by the Ethics committee of the Beijing Tiantan hospital that was affiliated to the Capital Medical University of the People's Republic of China (reference number QX2018-009-01). The study was conducted following the Declaration of Helsinki.
MoreTranslated text
Key words
Medical diagnosis,Medical physics,Declaration of Helsinki,Deep learning,Test set,Brain tumor,Medicine,Artificial intelligence,Competing interests,Diagnostic accuracy,Mr images
AI Read Science
Must-Reading Tree
Example
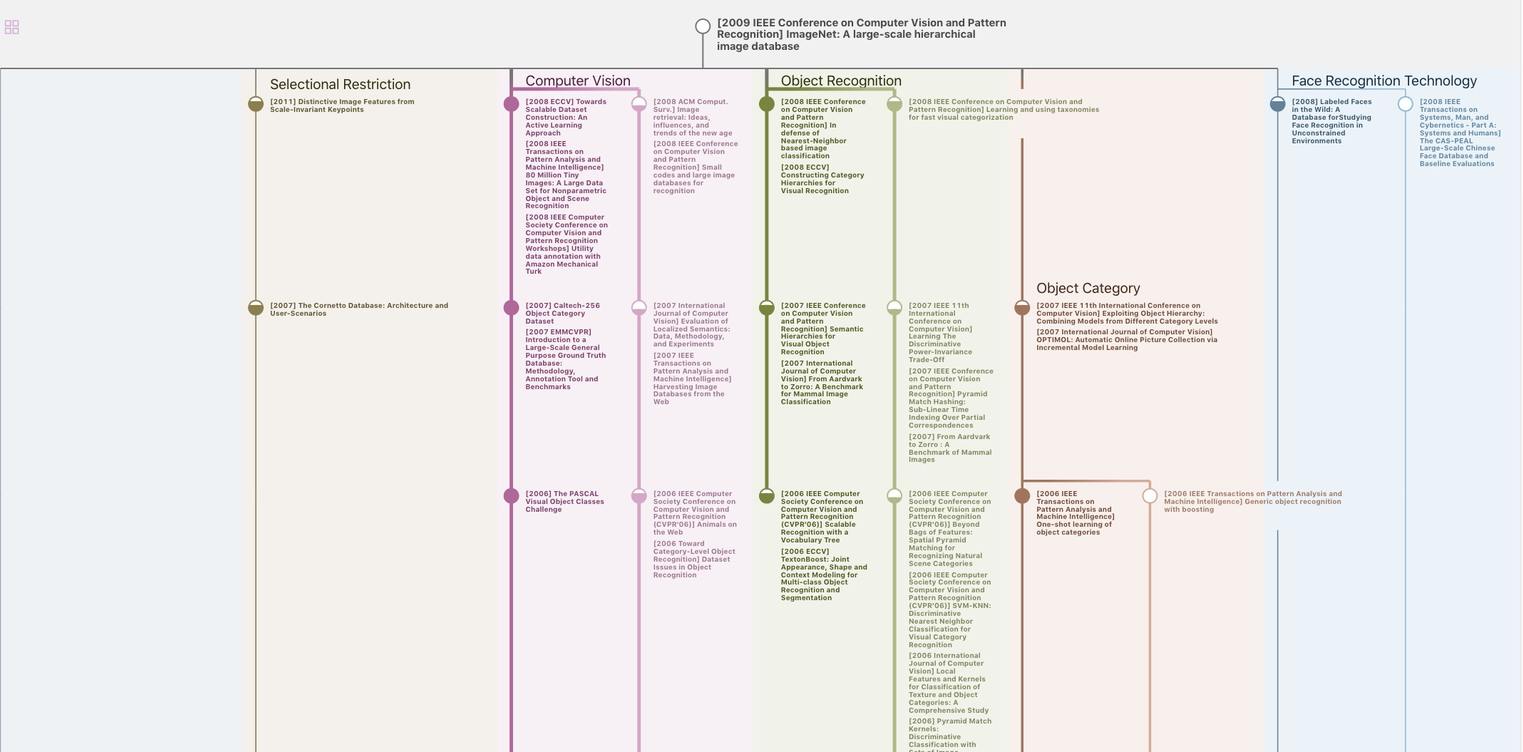
Generate MRT to find the research sequence of this paper
Chat Paper
Summary is being generated by the instructions you defined