Node Co-occurrence based Graph Neural Networks for Knowledge Graph Link Prediction
WSDM(2022)
摘要
ABSTRACTWe introduce a novel embedding model, named NoGE, which aims to integrate co-occurrence among entities and relations into graph neural networks to improve knowledge graph completion (i.e., link prediction). Given a knowledge graph, NoGE constructs a single graph considering entities and relations as individual nodes. NoGE then computes weights for edges among nodes based on the co-occurrence of entities and relations. Next, NoGE proposes Dual Quaternion Graph Neural Networks (DualQGNN) and utilizes DualQGNN to update vector representations for entity and relation nodes. NoGE then adopts a score function to produce the triple scores. Comprehensive experimental results show that NoGE obtains state-of-the-art results on three new and difficult benchmark datasets CoDEx for knowledge graph completion.
更多查看译文
关键词
graph neural networks, knowledge graph completion, quaternion
AI 理解论文
溯源树
样例
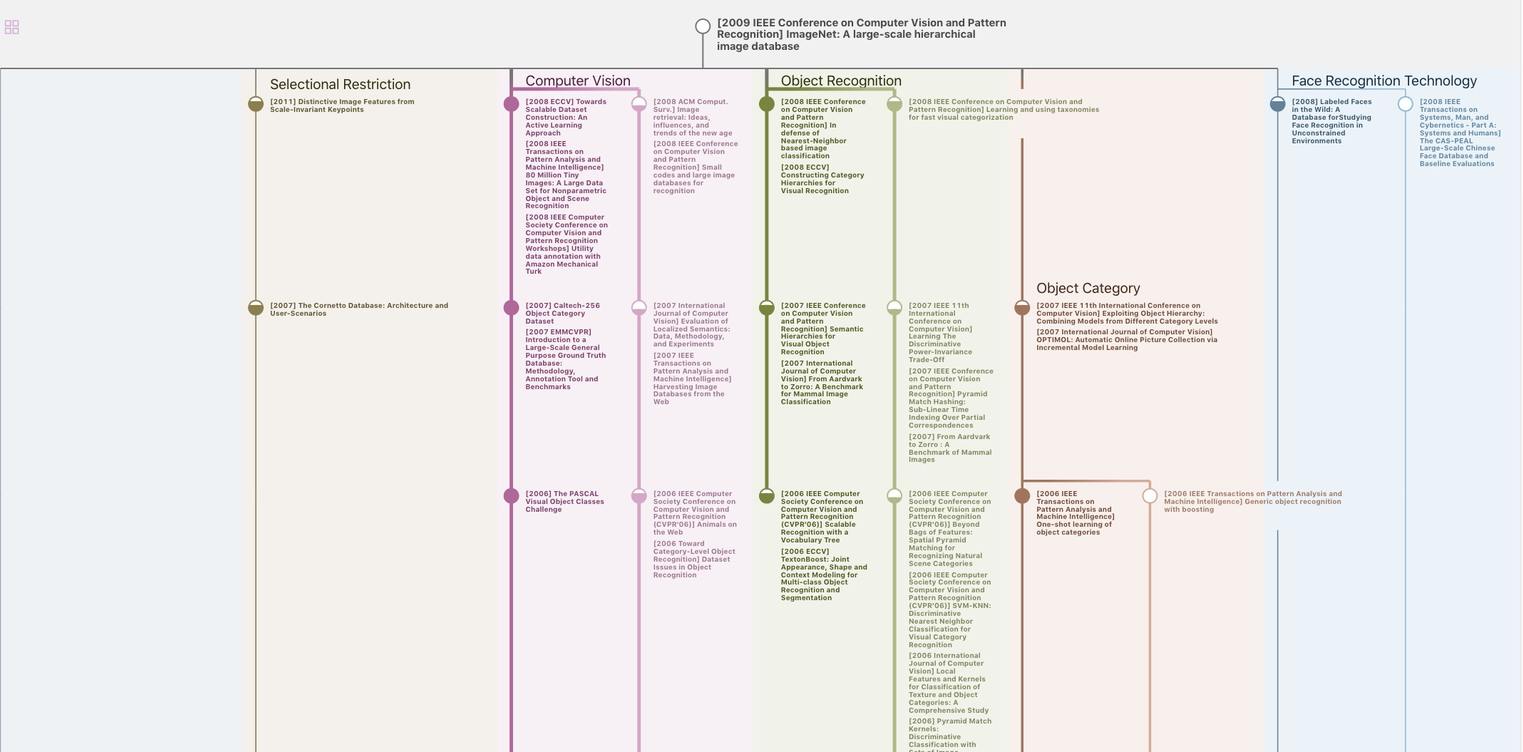
生成溯源树,研究论文发展脉络
Chat Paper
正在生成论文摘要