Causal Modeling With Stochastic Confounders
24TH INTERNATIONAL CONFERENCE ON ARTIFICIAL INTELLIGENCE AND STATISTICS (AISTATS)(2021)
摘要
This work extends causal inference in temporal models with stochastic confounders. We propose a new approach to variational estimation of causal inference based on a representer theorem with a random input space. We estimate causal effects involving latent confounders that may be interdependent and time-varying from sequential, repeated measurements in an observational study. Our approach extends current work that assumes independent, non-temporal latent confounders with potentially biased estimators. We introduce a simple yet elegant algorithm without parametric specification on model components. Our method avoids the need for expensive and careful parameterization in deploying complex models, such as deep neural networks in existing approaches, for causal inference and analysis. We demonstrate the effectiveness of our approach on various benchmark temporal datasets.
更多查看译文
关键词
stochastic confounders,modeling
AI 理解论文
溯源树
样例
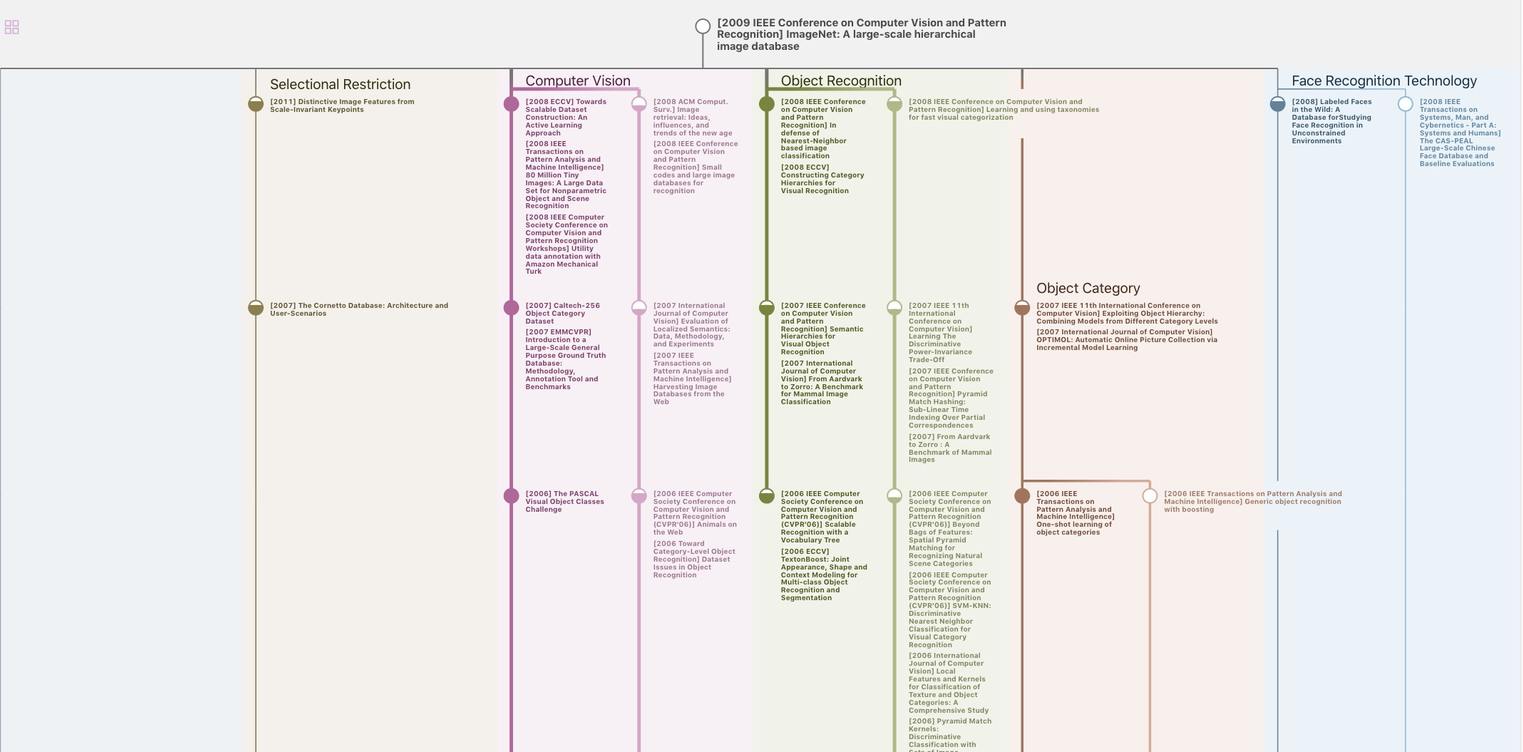
生成溯源树,研究论文发展脉络
Chat Paper
正在生成论文摘要