Learning Shared Subgraphs in Ising Model Pairs
24TH INTERNATIONAL CONFERENCE ON ARTIFICIAL INTELLIGENCE AND STATISTICS (AISTATS)(2021)
摘要
Probabilistic graphical models (PGMs) are effective for capturing the statistical dependencies in stochastic databases. In many domains (e.g., working with multimodal data), one faces multiple information layers that can be modeled by structurally similar PGMs. While learning the structures of PGMs in isolation is well-investigated, the algorithmic design and performance limits of learning from multiple coupled PGMs are not well-investigated. This paper considers learning the structural similarities shared by a pair of Ising PGMs. The objective is to learn only the shared structure with no regard for the structures exclusive to either of the graphs. This is significantly different from the existing approaches that focus on learning the entire structures of the graphs. This paper proposes an algorithm for learning the shared structure, evaluates its performance empirically and analytically, and compares the performance with that of the existing approaches.
更多查看译文
关键词
shared subgraphs,ising model pairs,learning
AI 理解论文
溯源树
样例
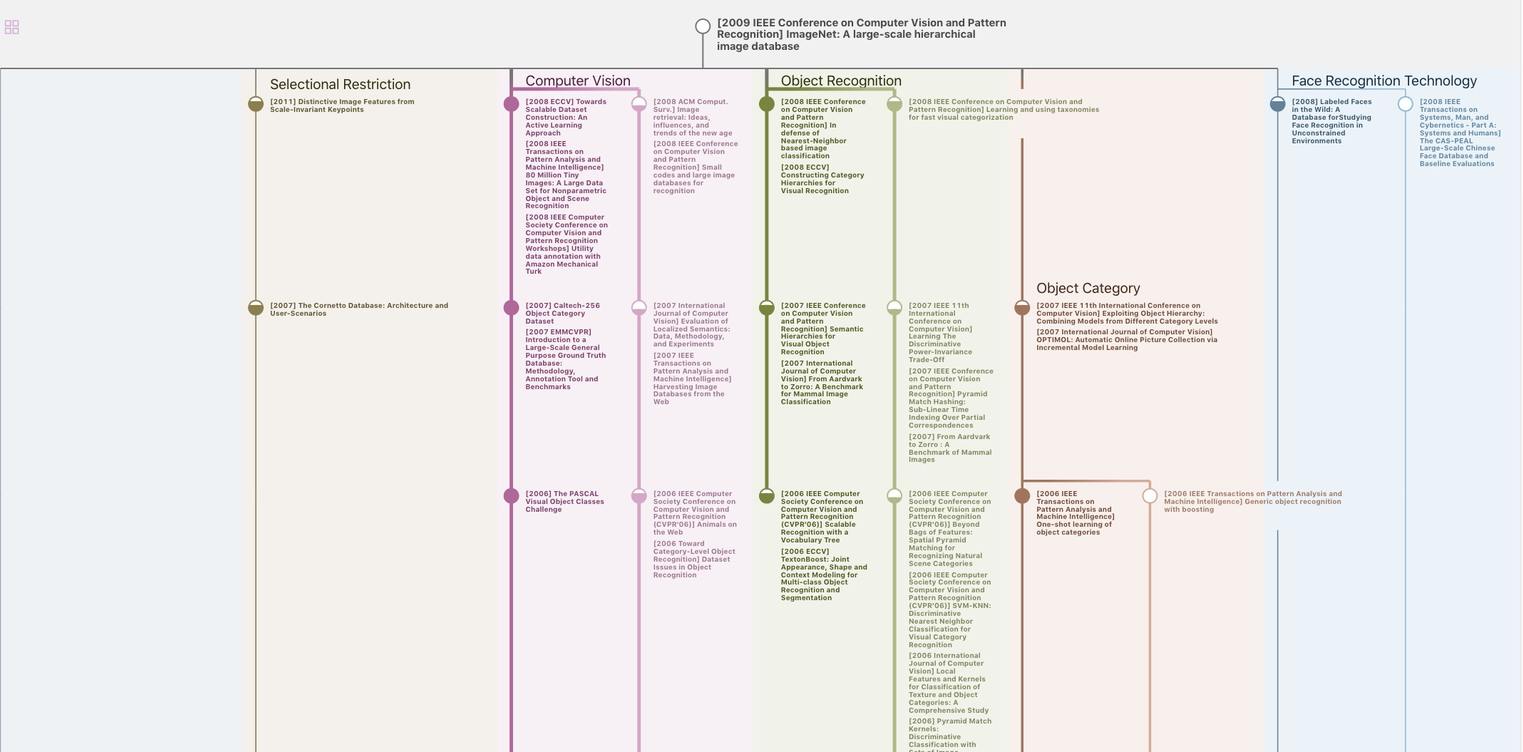
生成溯源树,研究论文发展脉络
Chat Paper
正在生成论文摘要