Multiple-manifold Generation with an Ensemble GAN and Learned Noise Prior
ADVANCES IN INTELLIGENT DATA ANALYSIS XIX, IDA 2021(2021)
摘要
Generative adversarial networks (GANs) learn to map samples from a noise distribution to a chosen data distribution. Recent work has demonstrated that GANs are consequently sensitive to, and limited by, the shape of the noise distribution. For example, for a single generator to map continuous noise (e.g. a uniform distribution) to discontinuous output (e.g. separate Gaussians), it must generate off-manifold points in the discontinuous region with nonzero probability. While existing applications generally ignore these outliers, they nevertheless represent a hindrance to accurate modeling in current frameworks. We address this problem by learning to generate from multiple networks such that the generator's output is an ensemble of distinct sub-generators. We contribute a novel formulation of multi-generator models where we learn a prior over the generators conditioned on the noise, parameterized by another neural network. Thus, this network not only learns the optimal rate to sample from each generator but also optimally shapes the noise received by each generator. The resulting Noise Prior GAN (NPGAN) achieves flexibility that surpasses both single generator models and previous multi-generator models even when the total number of parameters in the ensemble is the same as the single-generator models.
更多查看译文
关键词
ensemble gan,learned noise,multiple-manifold
AI 理解论文
溯源树
样例
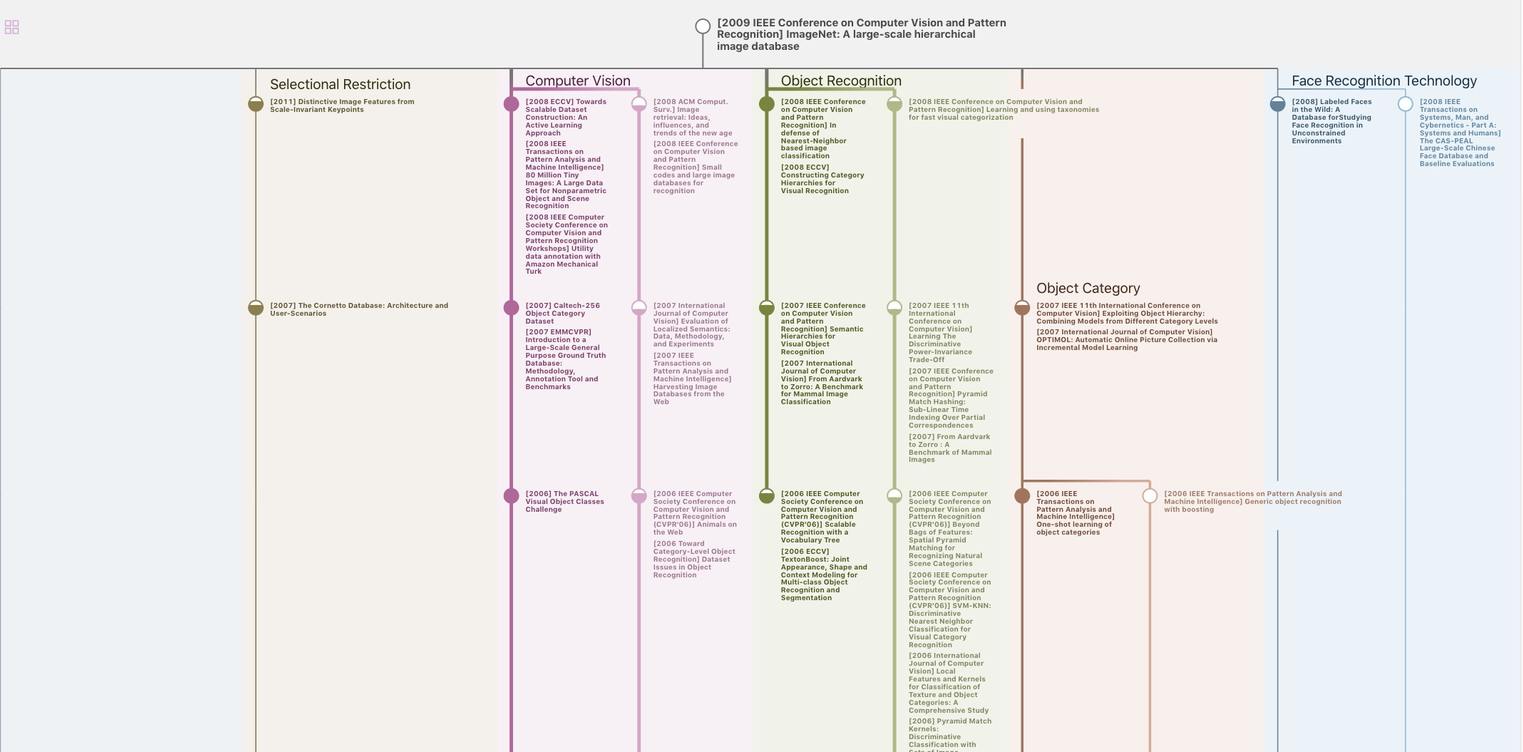
生成溯源树,研究论文发展脉络
Chat Paper
正在生成论文摘要