Knowledge-driven Answer Generation for Conversational Search
arxiv(2021)
摘要
The conversational search paradigm introduces a step change over the traditional search paradigm by allowing users to interact with search agents in a multi-turn and natural fashion. The conversation flows naturally and is usually centered around a target field of knowledge. In this work, we propose a knowledge-driven answer generation approach for open-domain conversational search, where a conversation-wide entities' knowledge graph is used to bias search-answer generation. First, a conversation-specific knowledge graph is extracted from the top passages retrieved with a Transformer-based re-ranker. The entities knowledge-graph is then used to bias a search-answer generator Transformer towards information rich and concise answers. This conversation specific bias is computed by identifying the most relevant passages according to the most salient entities of that particular conversation. Experiments show that the proposed approach successfully exploits entities knowledge along the conversation, and outperforms a set of baselines on the search-answer generation task.
更多查看译文
关键词
answer generation,conversational search,knowledge-driven
AI 理解论文
溯源树
样例
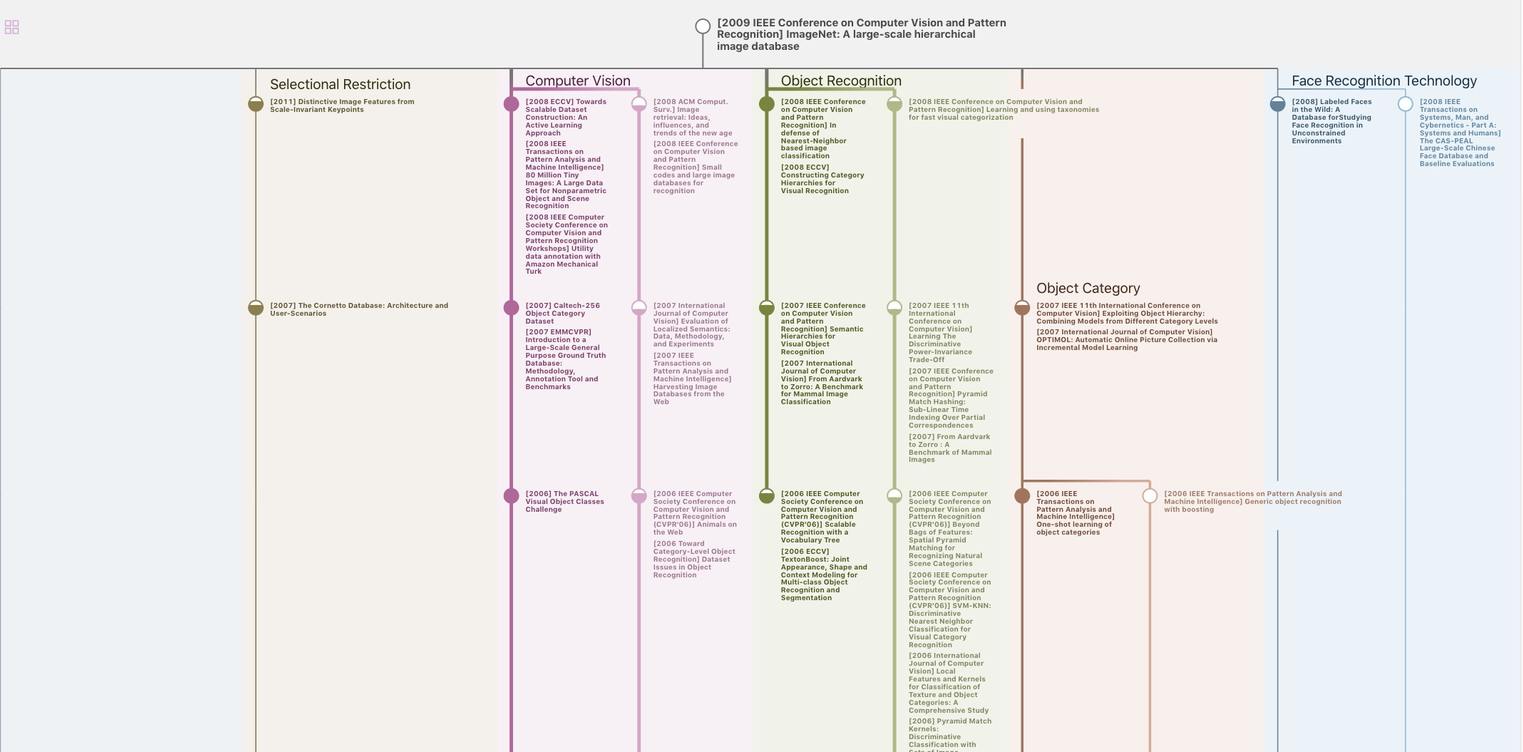
生成溯源树,研究论文发展脉络
Chat Paper
正在生成论文摘要