Clustering via Meta-path Embedding for Heterogeneous Information Networks
2020 IEEE International Conference on Knowledge Graph (ICKG)(2020)
摘要
A low-dimensional embedding of multiple nodes is very convenient for clustering, which is one of the most fundamental tasks for heterogeneous information networks (HINs). On the other hand, the random walk-based network embedding is proved to be equivalent to the method of matrix factorization whose computational cost is very expensive. Moreover, mapping different types of nodes into one metric space may result in incompatibility. To cope with the two challenges above, a meta-path embedding based clustering method (called MPEClus) is proposed in this paper. Firstly, the original network is transformed into several subnetworks with independent semantics specified by meta-paths to solve the incompatibility problem. Secondly, an approximate commute embedding method, bypassing eigen-decomposition to reduce computational cost, is leveraged to the representation learning of the nodes in each subnetwork. At last, a unified probabilistic generation model is designed to aggregate the vectorized representations learned in different metric spaces for clustering. Experiment results show that MPEClus is effective in HIN clustering and outperforms the state-of-the-art baselines on two real-world datasets.
更多查看译文
关键词
heterogeneous information networks,network embedding,graph clustering,representation learning
AI 理解论文
溯源树
样例
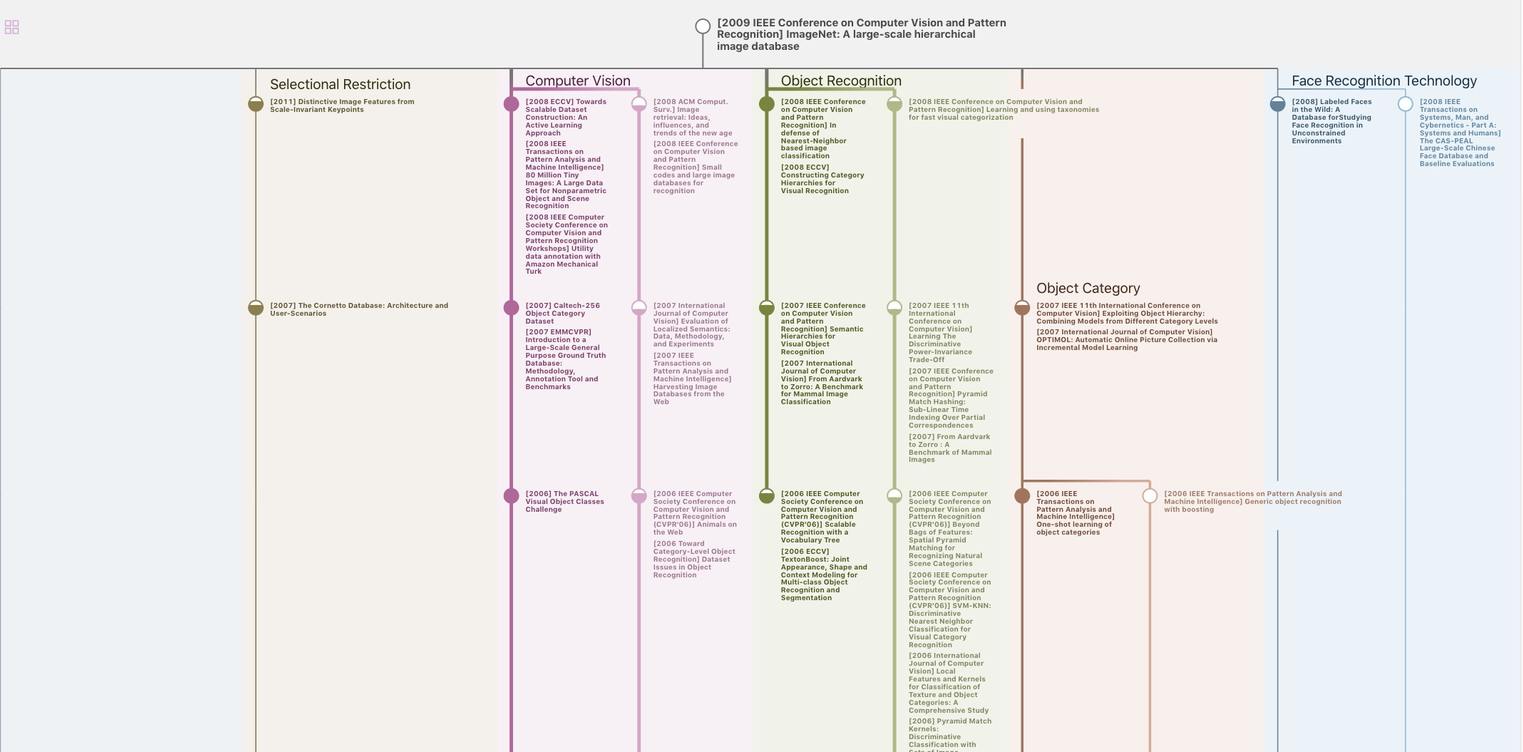
生成溯源树,研究论文发展脉络
Chat Paper
正在生成论文摘要