Structure-aware Interactive Graph Neural Networks for the Prediction of Protein-Ligand Binding Affinity
KDD '21: PROCEEDINGS OF THE 27TH ACM SIGKDD CONFERENCE ON KNOWLEDGE DISCOVERY & DATA MINING(2021)
摘要
Drug discovery often relies on the successful prediction of protein-ligand binding affinity. Recent advances have shown great promise in applying graph neural networks (GNNs) for better affinity prediction by learning the representations of protein-ligand complexes. However, existing solutions usually treat protein-ligand complexes as topological graph data, thus the biomolecular structural information is not fully utilized. The essential long-range interactions among atoms are also neglected in GNN models. To this end, we propose a structure-aware interactive graph neural network (SIGN) which consists of two components: polar-inspired graph attention layers (PGAL) and pairwise interactive pooling (PiPool). Specifically, PGAL iteratively performs the node-edge aggregation process to update embeddings of nodes and edges while preserving the distance and angle information among atoms. Then, PiPool is adopted to gather interactive edges with a subsequent reconstruction loss to reflect the global interactions. Exhaustive experimental study on two benchmarks verifies the superiority of SIGN.
更多查看译文
关键词
Binding Affinity Prediction,Graph Neural Network,Drug Discovery
AI 理解论文
溯源树
样例
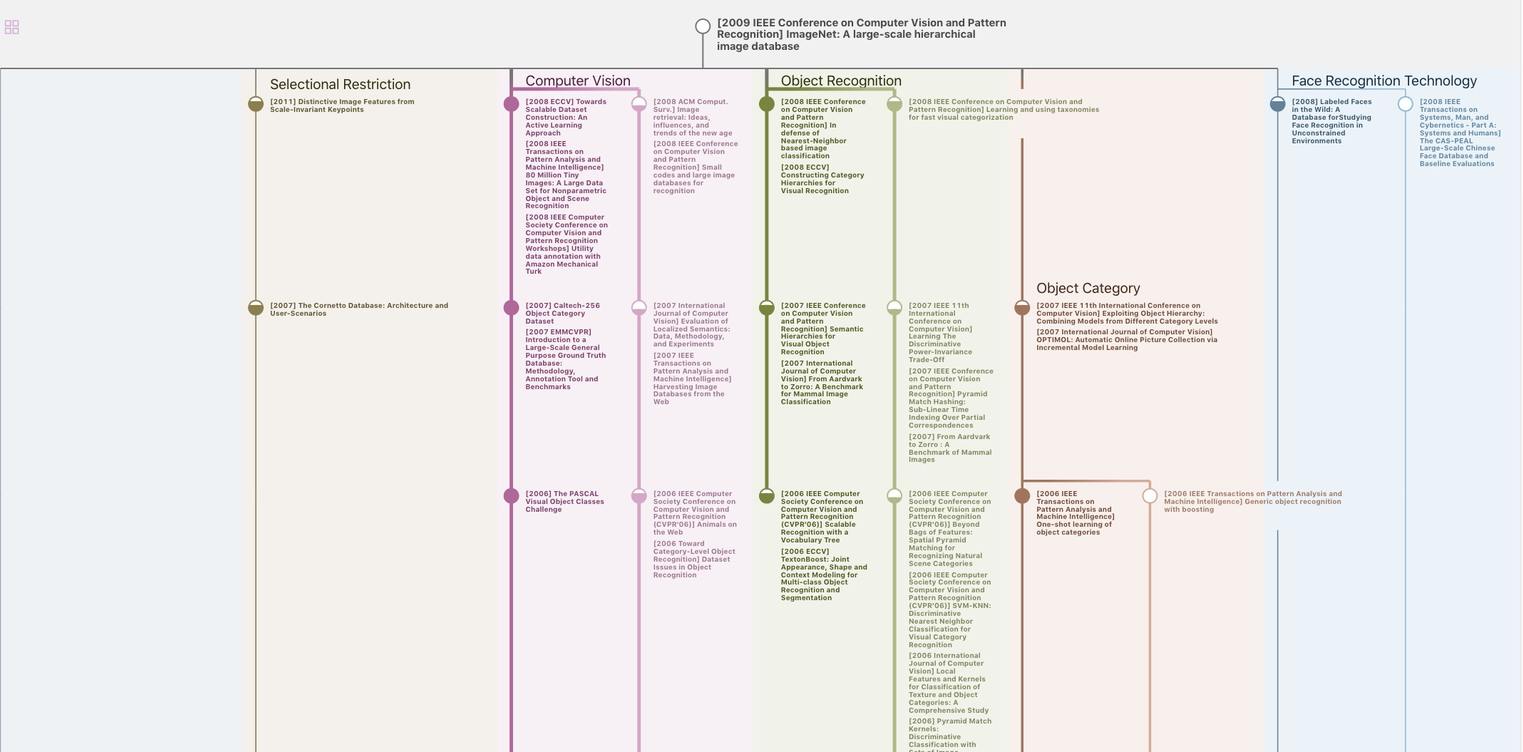
生成溯源树,研究论文发展脉络
Chat Paper
正在生成论文摘要