On Representation Learning for Scientific News Articles Using Heterogeneous Knowledge Graphs
International World Wide Web Conference(2021)
摘要
ABSTRACT In the era of misinformation and information inflation, the credibility assessment of the produced news is of the essence. However, fact-checking can be challenging considering the limited references presented in the news. This challenge can be transcended by utilizing the knowledge graph that is related to the news articles. In this work, we present a methodology for creating scientific news article representations by modeling the directed graph between the scientific news articles and the cited scientific publications. The network used for the experiments is comprised of the scientific news articles, their topic, the cited research literature, and their corresponding authors. We implement and present three different approaches: 1) a baseline Relational Graph Convolutional Network (R-GCN), 2) a Heterogeneous Graph Neural Network (HetGNN) and 3) a Heterogeneous Graph Transformer (HGT). We test these models in the downstream task of link prediction on the: a) news article - paper links and b) news article - article topic links. The results show promising applications of graph neural network approaches in the domains of knowledge tracing and scientific news credibility assessment.
更多查看译文
关键词
heterogeneous knowledge graphs, graph neural networks, misinformation, graph embeddings
AI 理解论文
溯源树
样例
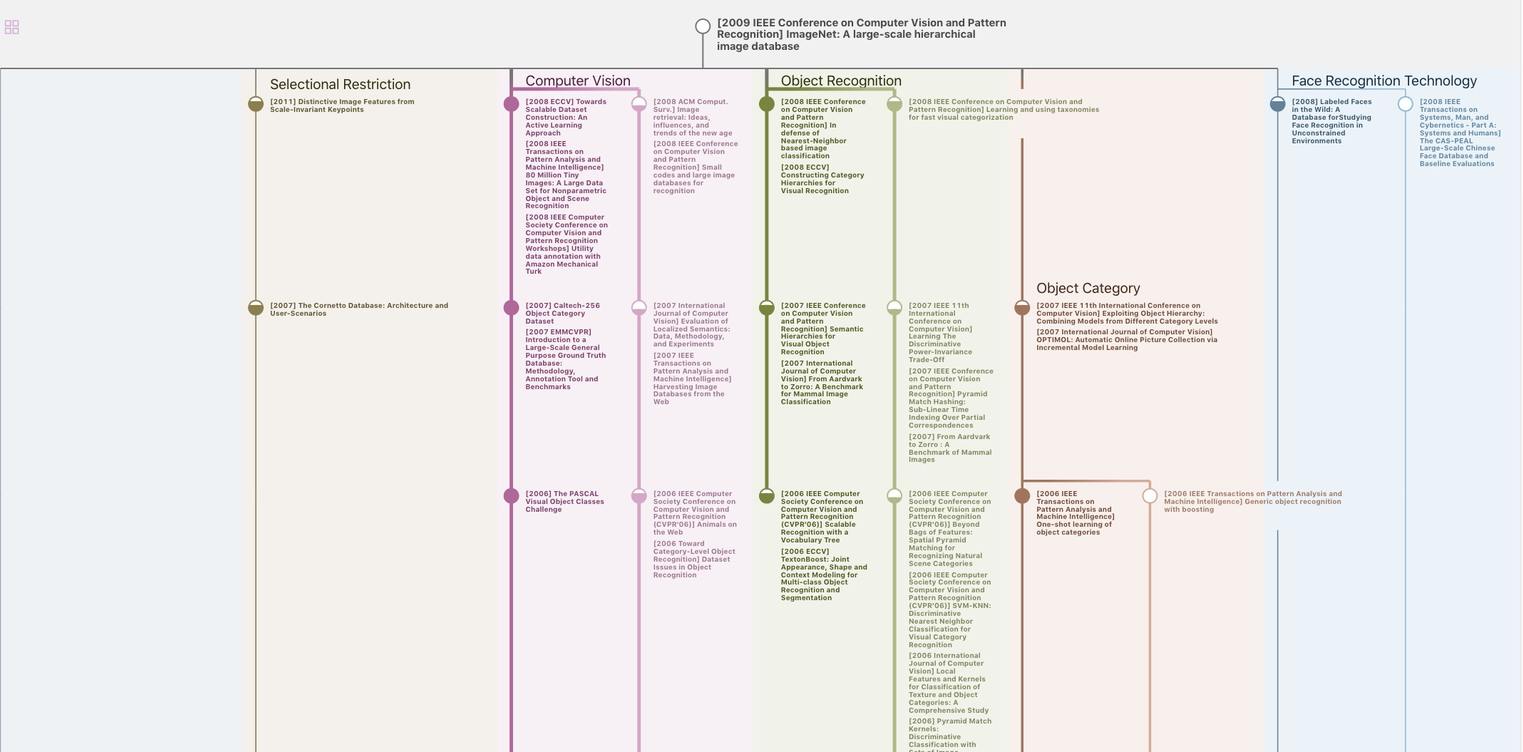
生成溯源树,研究论文发展脉络
Chat Paper
正在生成论文摘要