An Efficient 2D Method for Training Super-Large Deep Learning Models
CoRR(2021)
摘要
Huge neural network models have shown unprecedented performance in real-world applications. However, due to memory constraints, model parallelism must be utilized to host large models that would otherwise not fit into the memory of a single device. Previous methods like Megatron partition the parameters of the entire model among multiple devices, while each device has to accommodate the redundant activations in forward and backward pass. In this work, we propose Optimus, a highly efficient and scalable 2D-partition paradigm of model parallelism that would facilitate the training of infinitely large language models. In Optimus, activations are partitioned and distributed among devices, further reducing redundancy. In terms of isoefficiency, Optimus significantly outperforms Megatron. On 64 GPUs of TACC Frontera, Optimus achieves 1.48X speedup for training, 1.78X speedup for inference, and 8X increase in maximum batch size over Megatron. Optimus surpasses Megatron in scaling efficiency by a great margin. The code is available at https://github.com/xuqifan897/Optimus.
更多查看译文
关键词
matrix-matrix multiplication, distributed training, neural networks, natural language processing
AI 理解论文
溯源树
样例
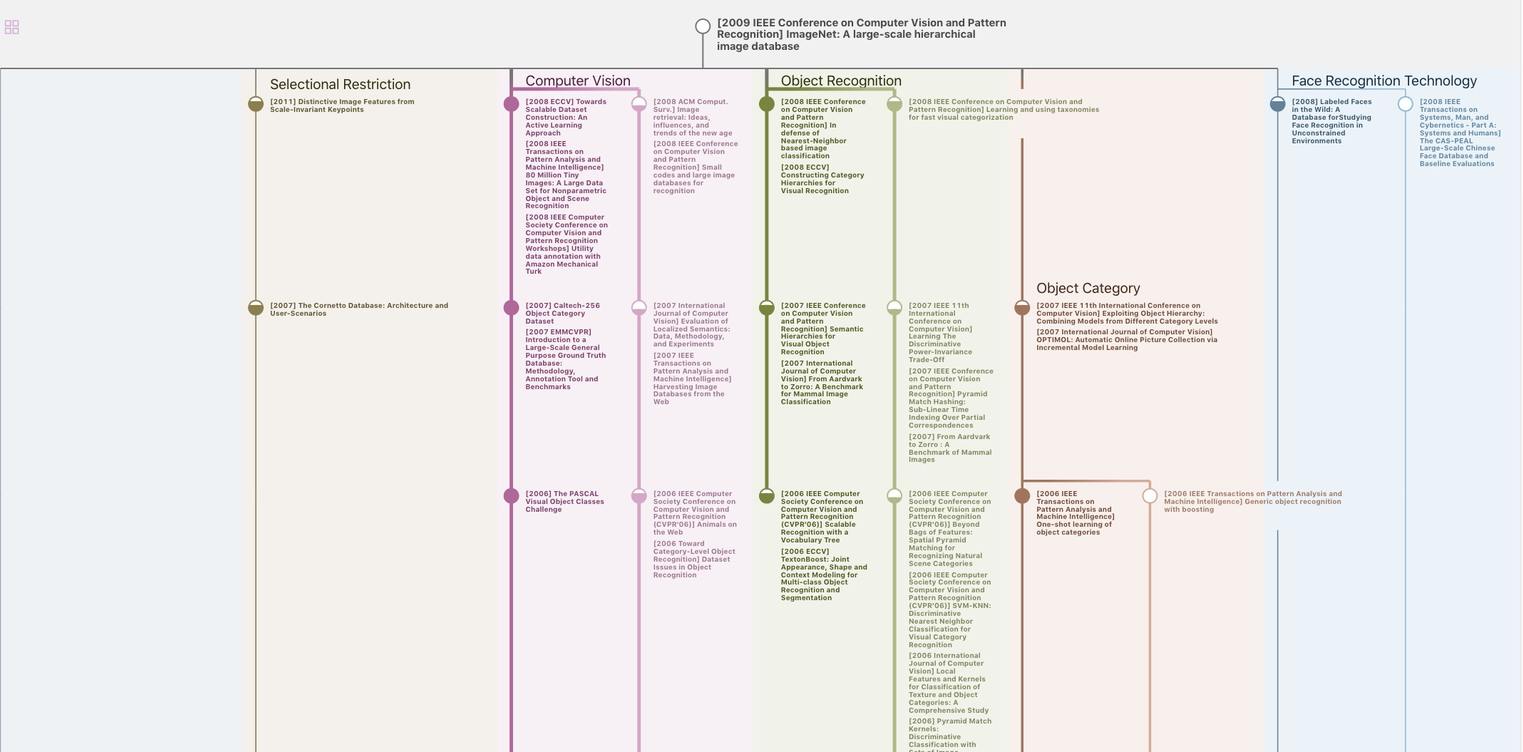
生成溯源树,研究论文发展脉络
Chat Paper
正在生成论文摘要