Boltzmann Tuning of Generative Models
arxiv(2021)
摘要
The paper focuses on the a posteriori tuning of a generative model in order to favor the generation of good instances in the sense of some external differentiable criterion. The proposed approach, called Boltzmann Tuning of Generative Models (BTGM), applies to a wide range of applications. It covers conditional generative modelling as a particular case, and offers an affordable alternative to rejection sampling. The contribution of the paper is twofold. Firstly, the objective is formalized and tackled as a well-posed optimization problem; a practical methodology is proposed to choose among the candidate criteria representing the same goal, the one best suited to efficiently learn a tuned generative model. Secondly, the merits of the approach are demonstrated on a real-world application, in the context of robust design for energy policies, showing the ability of BTGM to sample the extreme regions of the considered criteria.
更多查看译文
关键词
generative models
AI 理解论文
溯源树
样例
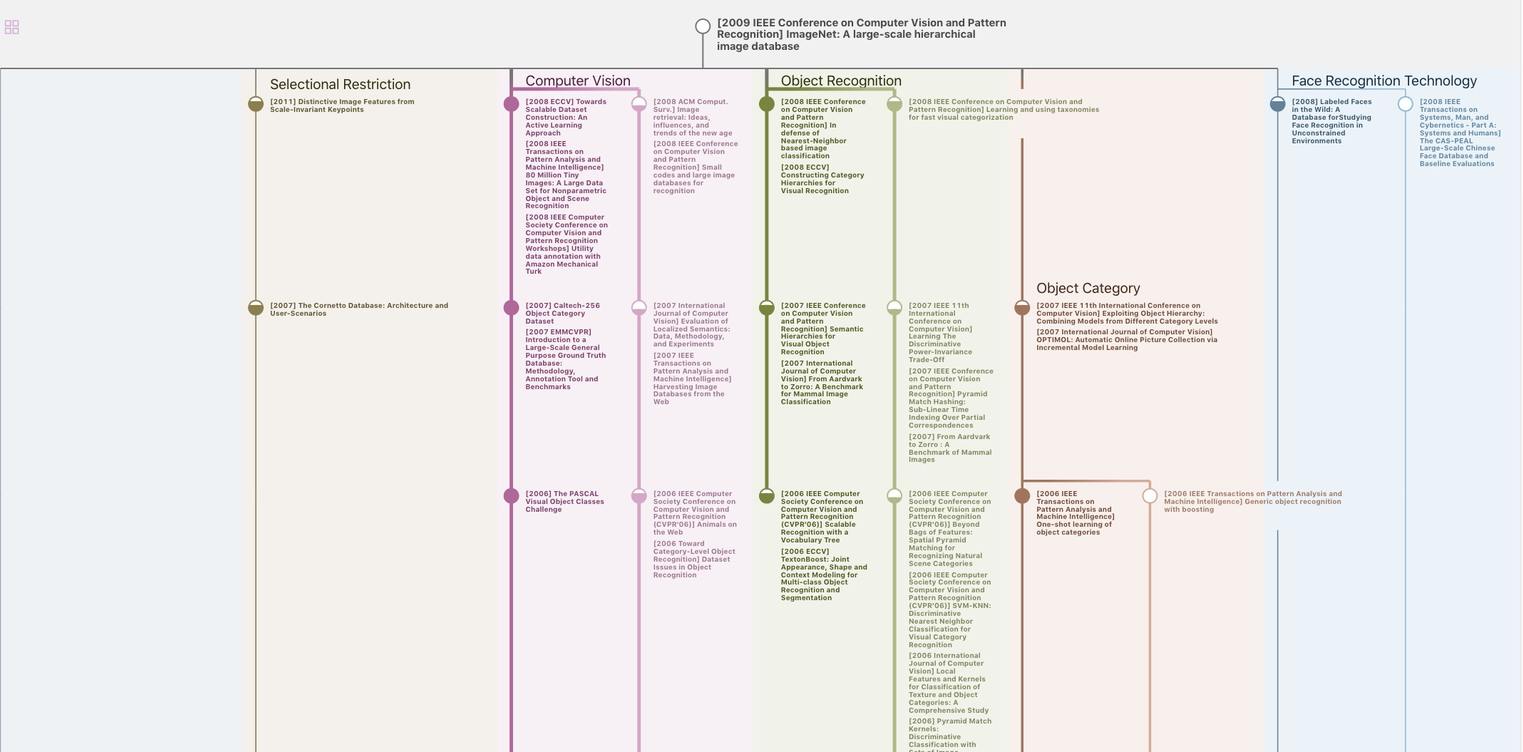
生成溯源树,研究论文发展脉络
Chat Paper
正在生成论文摘要