Comparing performance of iterative and non-iterative algorithms on various feature schemes for arrhythmia analysis.
Methods (San Diego, Calif.)(2021)
摘要
To evaluate the performance of the classic machine learning algorithms and the effectiveness of various features, the iterative algorithms (i.e., support vector machine (SVM), and least-squares SVM (LS-SVM)) and non-iterative algorithms (i.e., random forest (RF) and naive bayes (NB)) for six feature schemes were performed to classify the ECG recordings. The ECG recordings were initially filtered with a 0.1 Hz - 12 Hz band pass filter. Then 80 features, including 48 time domain, 18 frequency domain, 12 time-frequency and two principle component analysis (PCA) features, were extracted to construct six feature schemes. The RF, SVM, LS-SVM and NB were employed to discern a binary-classification task (i.e., normal and AF ECG recordings) and a tri- classification task (i.e., the normal, AF and ST change ECG recordings) for the six feature schemes. The results revealed that time domain, frequency domain features and PCA features can provide relatively reliable feature combinations to the RF and SVM. In addition, the RF yielded the highest F1-scores (0.8908 and 0.7535) for the binary-classification task and the tri-classification task than the SVM, LS-SVM and NB.
更多查看译文
AI 理解论文
溯源树
样例
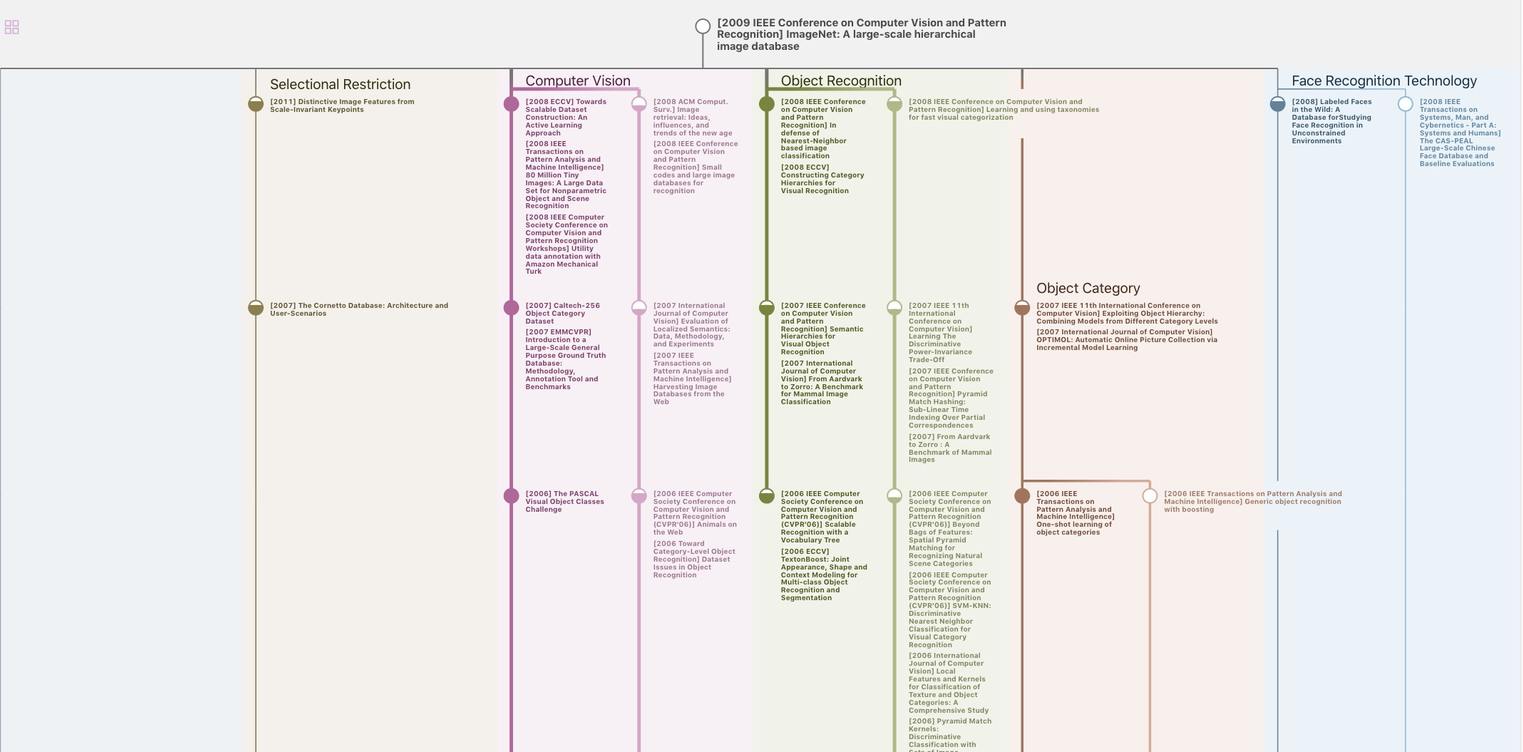
生成溯源树,研究论文发展脉络
Chat Paper
正在生成论文摘要