High-Performing Deep Learning Regression Models For Predicting Low-Pressure Co2 Adsorption Properties Of Metal-Organic Frameworks
JOURNAL OF PHYSICAL CHEMISTRY C(2020)
Abstract
Metal-organic frameworks (MOFs) have garnered interest as potential solid sorbent materials for postcombustion CO2 capture. With a seemingly infinite design space, high-throughput computational screening of MOFs has developed into an effective tool for the development of new materials. In this work, machine learning (ML) has been used to develop accurate quantitative structure-property relationship (QSPR) models to rapidly predict the CO2 working capacity and CO2/N-2 selectivity at the low-pressure conditions relevant to postcombustion carbon capture (0.15 bar CO2, 0.85 bar N-2). A database of over 340 000 MOFs constructed from hundreds of types of building units arranged in over 1000 net topologies was used to train and test the models. Neural network ML models were optimized using six geometric descriptors along with three so-called chemical descriptors, namely, the atomic property-weighted radial distribution function (AP-RDF) and some variants thereof, the bag-of-atoms, and the chemical motif density descriptors. The ML models built using geometric descriptors alone resulted in test set correlation R-2 values of only 0.71 and 0.75 for CO2 working capacity and CO2/N-2 selectivity, respectively. ML models built with a single type of chemical descriptor all outperformed the geometry-only models giving R-2 values ranging from 0.83 to 0.94 with the AP-RDF model being the most accurate. Overall, the best model was built using a combination of AP-RDF, chemical motif, and geometric descriptors (R-2 = 0.96 when predicting the CO2 working capacity and R-2 = 0.95 for the selectivity). To date, these are the most accurate ML models for predicting low-pressure gas uptake of MOFs. The combined model was able to capture 994 of the true top 1000 MOFs (from a test set of similar to 70 000) within the top 5000 MOFs as predicted by the model with CO, working capacity as the target. Thus, if the ML model were used to prescreen materials for more compute intensive GCMC simulations, then it would result in a greater than 10 times speed up while still capturing >99% of high-performing materials. These results highlight the importance of chemical descriptors in predicting low-pressure gas adsorption properties in nanoporous materials.
MoreTranslated text
AI Read Science
Must-Reading Tree
Example
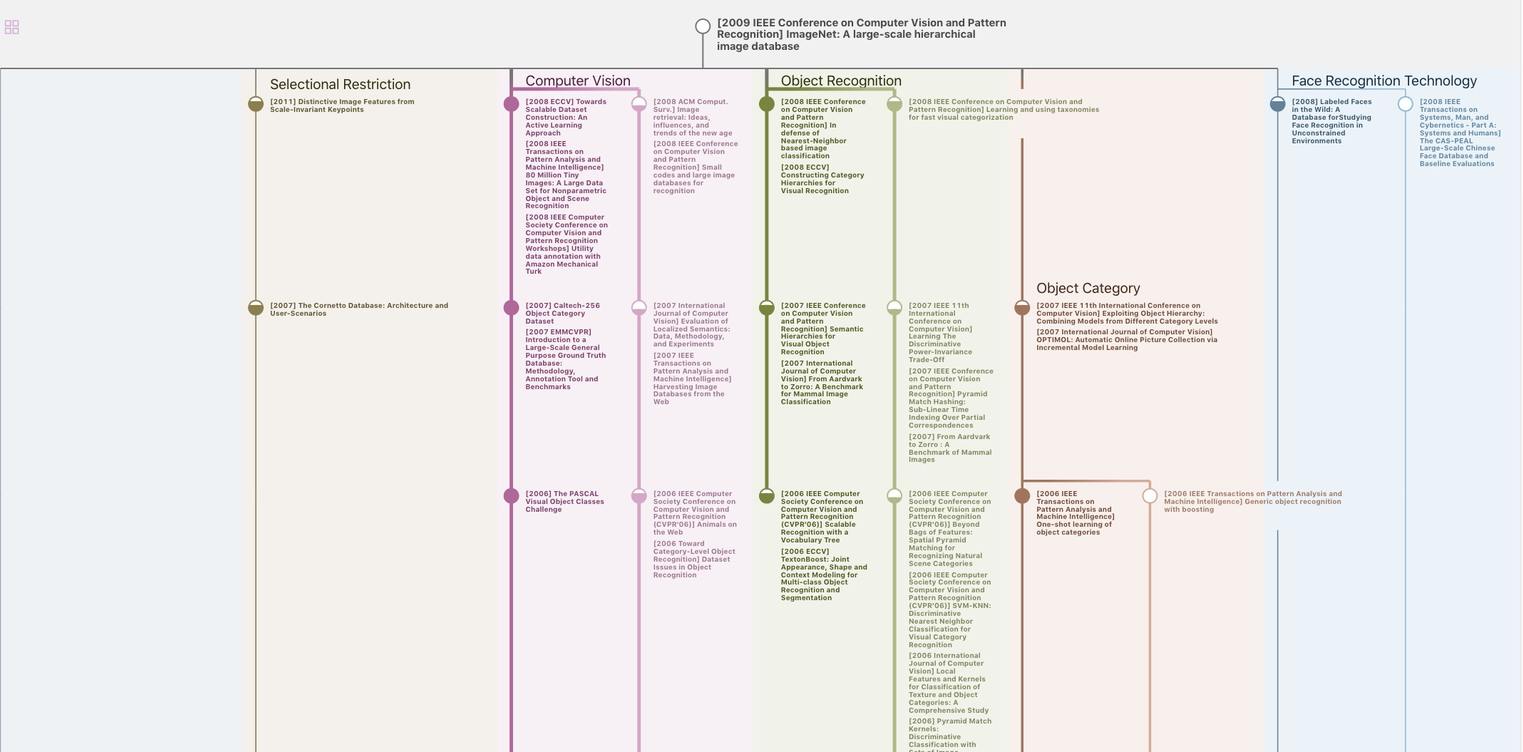
Generate MRT to find the research sequence of this paper
Chat Paper
Summary is being generated by the instructions you defined