Pycurt: An Automated Data Curation Workflow For Radiotherapy Big Data Analysis Using Pythons' Nypipe
INTERNATIONAL JOURNAL OF RADIATION ONCOLOGY BIOLOGY PHYSICS(2020)
摘要
Personalized medicine in Radiotherapy (RT) aims to increase tumor control probability and decrease normal tissue toxicity. Recently, Radiomics is widely used to infer tumor/tissue characteristics and link outcome to applied dose and underlying biology. The building of robust models requires high amounts of data, which are often only available from multiple centers/sites. Missing standardization of data (inconsistent naming schemes, differences in acquisition parameters) makes manual curation necessary, which can be extremely time-consuming. Here, we propose a fully automated general framework that can sort all relevant RT data with minimal human intervention - pyCuRT. Our method builds upon NyPipe, a Python package used to create complex analysis workflows. Any DICOM directory containing different radiological data e.g., batch exported from PACS systems without specific structure requirements, can be used as input for pyCuRT. It checks the integrity of files and sorts them based on DICOM attributes. For RT data, using information from the RT Plan, pyCuRT links together the DICOM-RT objects, i.e., the RT planning CT, Structure Set (SS), and Dose Distribution (DD). Furthermore, the structure within the SS showing the highest overlap with the DD can be automatically identified, allowing the extraction of inconsistently named structures. The final output has a subject/session/scan structure. Parallelization is implemented to speed up computation on multi-core machines. Successful curation has been achieved in retrospectively collected data cohorts of 3 different cancer entities (brain n = 621, rectal n = 127, and pancreatic n = 13 for a total of ∼50000 scans), from several various institutions across Germany. In the rectal cohort, e.g., from more than 2000 present images, 774 CT scans with 224 unique series descriptions were identified. From them, pyCuRT extracted the RT planning CTs, and linked them to the corresponding RT-DD and RT-SS automatically. Furthermore, all structures with any combination of GTV or PTV in the name were correctly extracted from the SSs and saved as NIFTI (the 35 RT-SS not containing those structures were identified correctly). The total processing time was around 2 hours and a half, which corresponds to less than 3 seconds per scan. We propose a new, fully automated method to curate radiotherapy data coming from different institutions and vendors. Its robustness and usability were demonstrated in three cohorts of tumor entities treated with RT. Future work will expand the workflow to automatically classify MR sequences.
更多查看译文
关键词
radiotherapy big data analysis,automated data curation workflow,big data,pythons
AI 理解论文
溯源树
样例
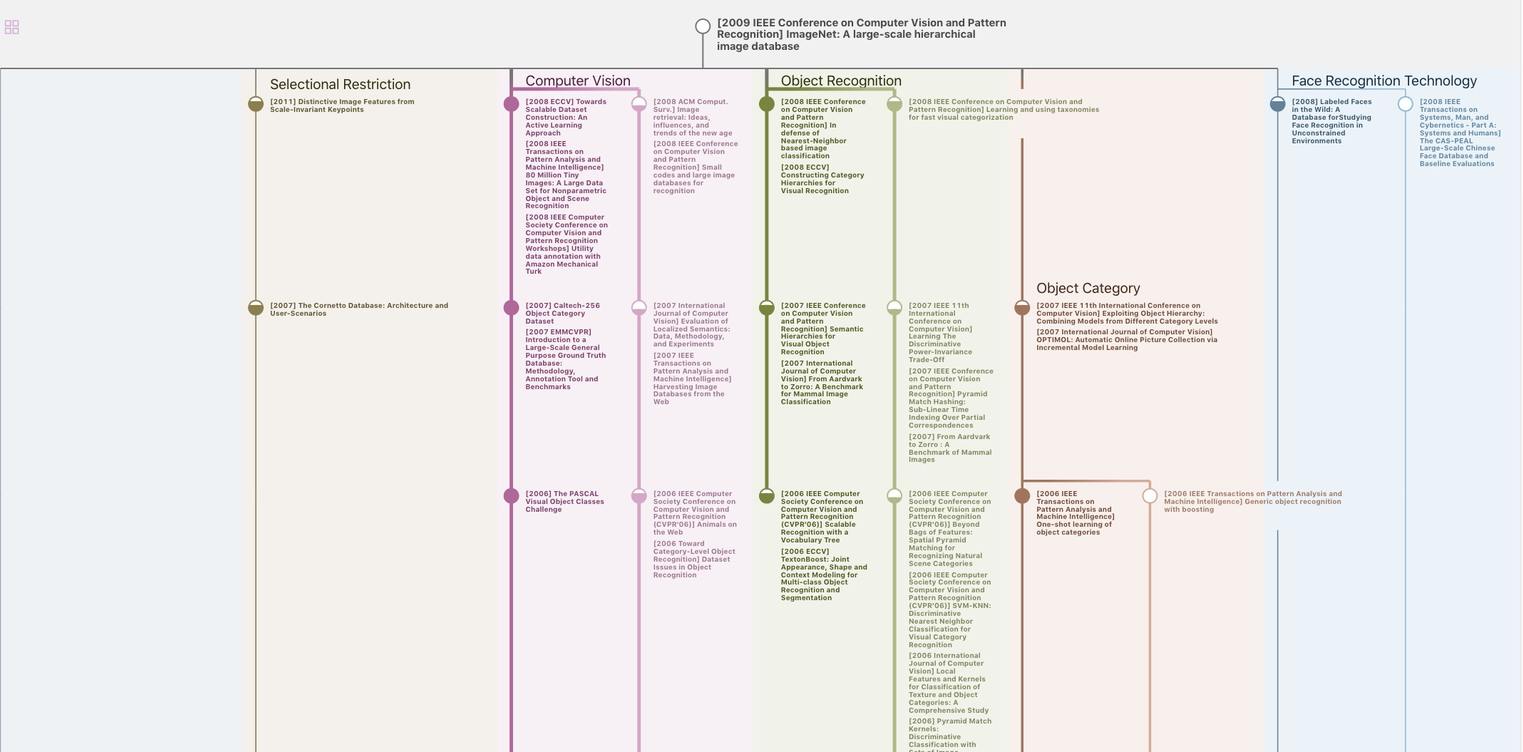
生成溯源树,研究论文发展脉络
Chat Paper
正在生成论文摘要