An Approach for Assessing Quality of Labeled Data for a Machine Learning Task in Malaria Detection
The Compass(2020)
摘要
While microscopy diagnosis through supervised learning for image analysis notably contributes to malaria detection, it has limitations. Among its principle challenges is the manual and tiresome process of data annotation for the classification task. The manual annotation of data is prone to inaccuracy defects due to bias, subjectivity and unclear images resulting into many false positives. This is normally due to personal independent judgements that vary from individual microscopists hence summatively affecting the accuracy of the model. In this paper, we seek to investigate the possibility of classifying the negative far examples and the positive near examples from the positives in thick blood smear images for malaria detection. Assessing the classification performance could potentially inform us of the quality of training dataset and guide n selecting the best training dataset for a malaria parasite detection task. We employ the Mean Squared Error (MSE) to distinguish between positive and negative images. We later investigate the performance of the VGG-16 classification model based on how close or far negative examples are from positives. Experimental results showed that negative examples far from the positives produce better results than those near and that the proposed method could potentially be used to reduce false positives and bias in the training data.
更多查看译文
AI 理解论文
溯源树
样例
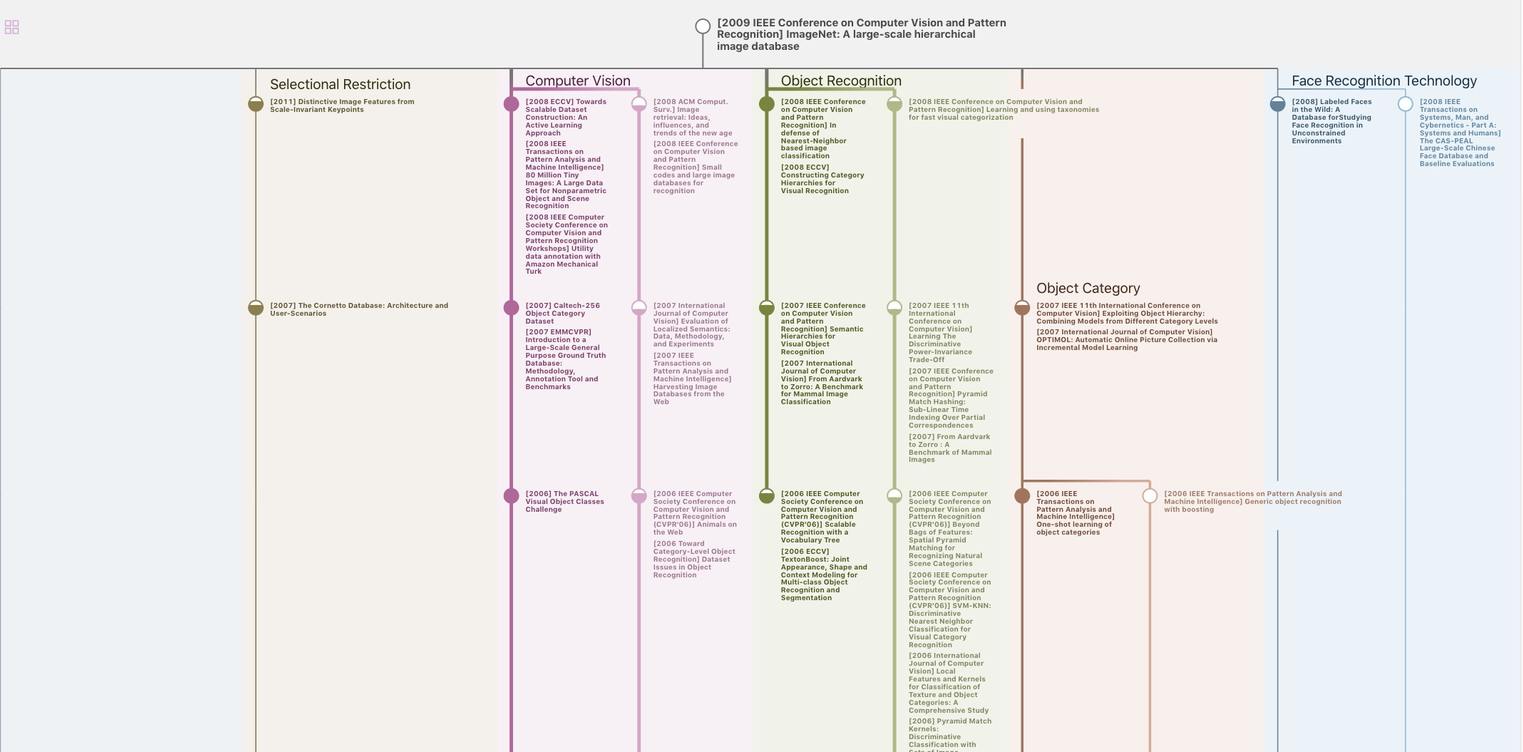
生成溯源树,研究论文发展脉络
Chat Paper
正在生成论文摘要