Fill-in-the-blank as a Challenging Video Understanding Evaluation Framework
CoRR(2021)
Abstract
Work to date on language-informed video understanding has primarily addressed two tasks: (1) video question answering using multiple-choice questions, where models perform relatively well because they exploit the fact that candidate answers are readily available; and (2) video captioning, which relies on an open-ended evaluation framework that is often inaccurate because system answers may be perceived as incorrect if they differ in form from the ground truth. In this paper, we propose fill-in-the-blanks as a video understanding evaluation framework that addresses these previous evaluation drawbacks, and more closely reflects real-life settings where no multiple choices are given. The task tests a system understanding of a video by requiring the model to predict a masked noun phrase in the caption of the video, given the video and the surrounding text. We introduce a novel dataset consisting of 28,000 videos and fill-in-the-blank tests. We show that both a multimodal model and a strong language model have a large gap with human performance, thus suggesting that the task is more challenging than current video understanding benchmarks.
MoreTranslated text
Key words
evaluation,video,fiber,understanding,fill-in-the-blanks
AI Read Science
Must-Reading Tree
Example
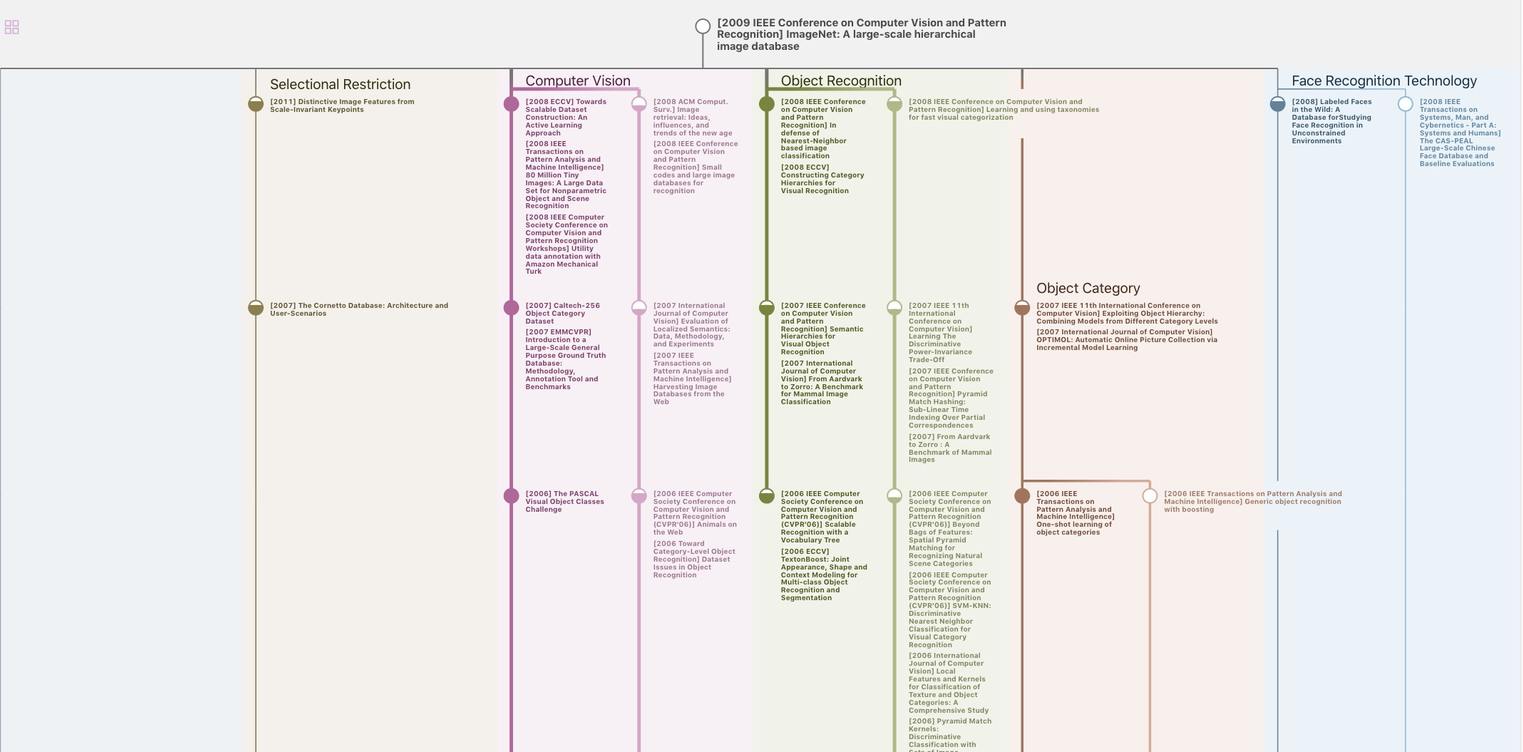
Generate MRT to find the research sequence of this paper
Chat Paper
Summary is being generated by the instructions you defined