Behavioral Discrimination And Time-Series Phenotyping Of Birdsong Performance
PLOS COMPUTATIONAL BIOLOGY(2021)
摘要
Variation in the acoustic structure of vocal signals is important to communicate social information. However, relatively little is known about the features that receivers extract to decipher relevant social information. Here, we took an expansive, bottom-up approach to delineate the feature space that could be important for processing social information in zebra finch song. Using operant techniques, we discovered that female zebra finches can consistently discriminate brief song phrases ("motifs") from different social contexts. We then applied machine learning algorithms to classify motifs based on thousands of time-series features and to uncover acoustic features for motif discrimination. In addition to highlighting classic acoustic features, the resulting algorithm revealed novel features for song discrimination, for example, measures of time irreversibility (i.e., the degree to which the statistical properties of the actual and time-reversed signal differ). Moreover, the algorithm accurately predicted female performance on individual motif exemplars. These data underscore and expand the promise of broad time-series phenotyping to acoustic analyses and social decision-making.Author summaryVariation in vocal performance can provide information about the social context and motivation of the signaler. For example, prosodic changes to the pitch, tempo, or loudness of speech can reveal a speaker's emotional state or motivation, even when speech content is unchanged. Similarly, in songbirds like the zebra finch, males produce songs with the same acoustic elements but with different vocal performance when courting females and when singing alone, and females strongly prefer to hear the courtship song. Here we integrated behavioral and computational approaches to reveal the acoustic features that female zebra finches might use for social discrimination. We first discovered that females excelled at distinguishing between brief phrases of courtship and non-courtship song. We next extracted thousands of time-series features from courtship and non-courtship phrases using a highly-comparative time series analysis (HCTSA) toolbox, and trained machine learning algorithms to use those features to discriminate between phrases. The machine learning algorithm identified features important for discriminating between courtship and non-courtship song phrases, some of which have not been implicated before, and reliably predicted the discrimination abilities of females. Together, these data highlight the power of expansive and bottom-up approaches to reveal acoustic features important for social discrimination.
更多查看译文
关键词
Avian Vocal Communication,Vocal Learning,Social Learning
AI 理解论文
溯源树
样例
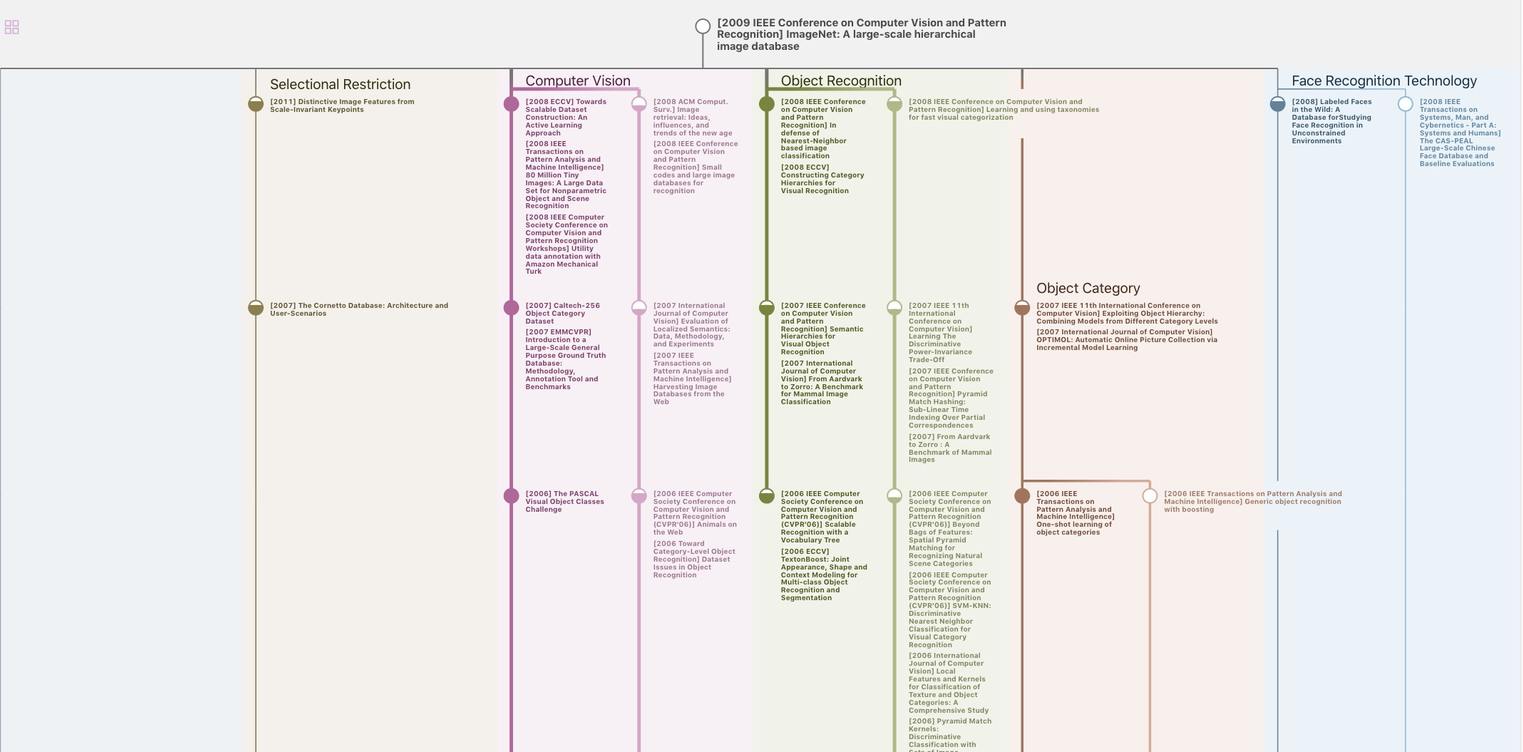
生成溯源树,研究论文发展脉络
Chat Paper
正在生成论文摘要