Reducing Reporting Burden Of Healthcare Data Using Robust Principal Component Analysis
2020 IEEE INTERNATIONAL CONFERENCE ON BIG DATA (BIG DATA)(2020)
摘要
The US government imposes reporting requirements on healthcare providers as health metrics are important for assessing and improving the US healthcare system. However, proposed health metrics requirements can be unnecessarily burdensome if the preliminary analysis does not consider possible redundancies between the various metrics. Accordingly, if some subset of the proposed metrics could be demonstrated to contain nearly all the information of the full set of metrics, then the reporting burden could be substantially lightened with minimum impact. However, such an analysis is complicated by anomalies in the collected metrics. Accordingly, we propose a machine learning approach which simultaneously identifies redundancies in collected healthcare metrics and identifies anomalies in those collected metrics.
更多查看译文
关键词
robust principal component analysis, anomaly detection, health analytics, dimension reduction
AI 理解论文
溯源树
样例
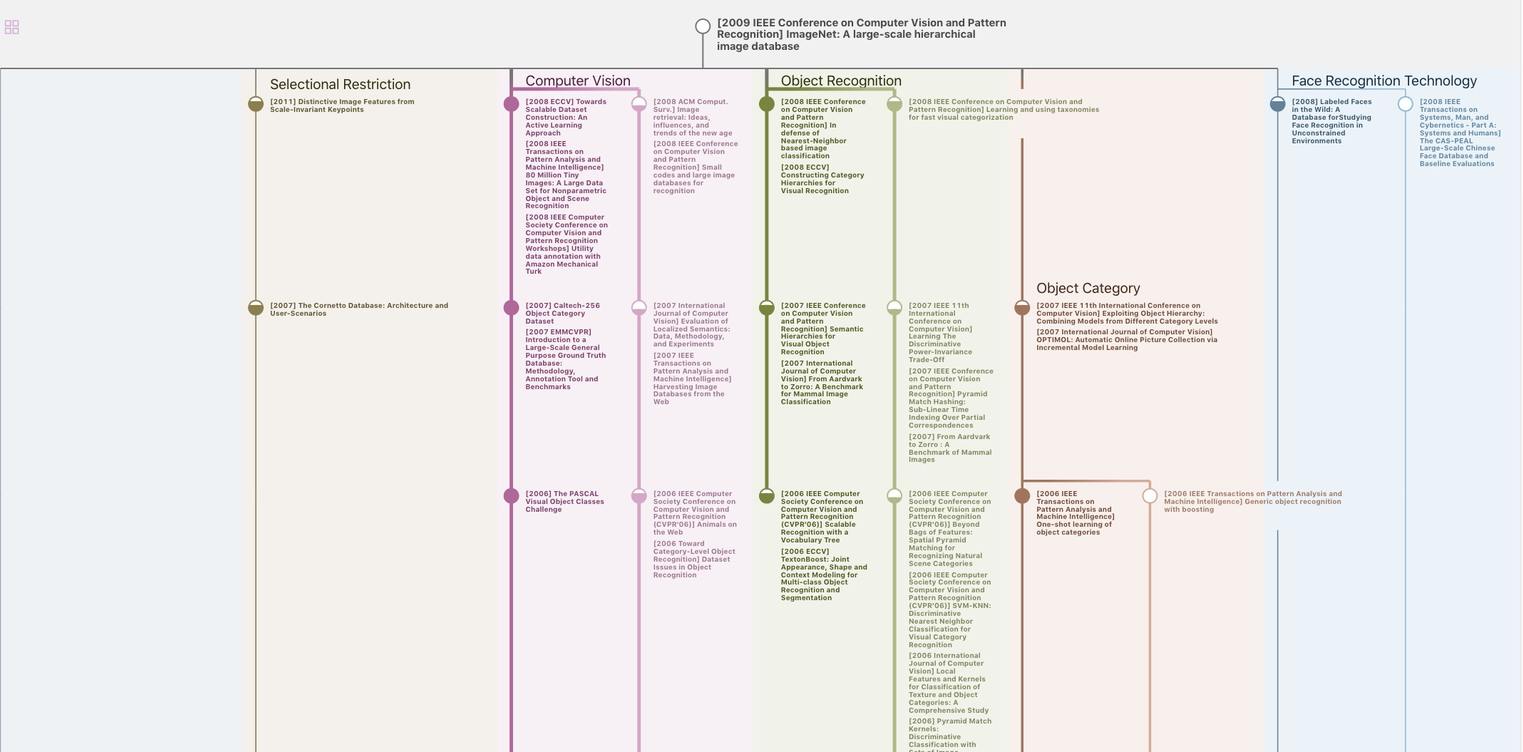
生成溯源树,研究论文发展脉络
Chat Paper
正在生成论文摘要