Towards Modular Modeling and Analytic for Multi-Modal Transportation Networks
2020 IEEE INTERNATIONAL CONFERENCE ON BIG DATA (BIG DATA)(2020)
摘要
The growing urban population and the changes in travel behavior with the help of IoT has created a market for new generations of transportation systems. Multi-Modal Mobility (MMM) is one of the major trends that is driving this transformation toward seamless and reliable transportation. However, due to the disconnected infrastructure of different modes of transportation, there is a need to develop advanced analytical tools to optimize the operational and service functions of different modes. Our goal is to build methods for integration and interaction of different modes. We propose a segmentation method to have a holistic view of the MMM network, but yet independent operation of different modalities. To this end, we encode the area into a uniform set of hexagonal-shaped cells and then extract features based on various modes of transportation. Then, we leverage cell features and the segmented network structure for analysis. As a case study, we specifically focus on demand-based analysis as it is an essential task for transportation systems. The demand pattern knowledge can improve the transportation system in both micro and macro level decision making. In this paper, we propose a graph analytic framework for the segmented MMM ecosystem. We utilize graph neural networks (GNNs) to perform demand-based clustering on the segmented area. Our focus is to cluster an area into categories based on the MMM demand patterns using the ridership data. We use Boston Bay open dataset with multiple modes of public transportation for analysis.
更多查看译文
关键词
Multi Modal Mobility, Public Transportation, Ride-hailing, Internet of Things, Demand Forecast, Graph Neural Networks, Clustering, Graph Embedding, Graph Analytic
AI 理解论文
溯源树
样例
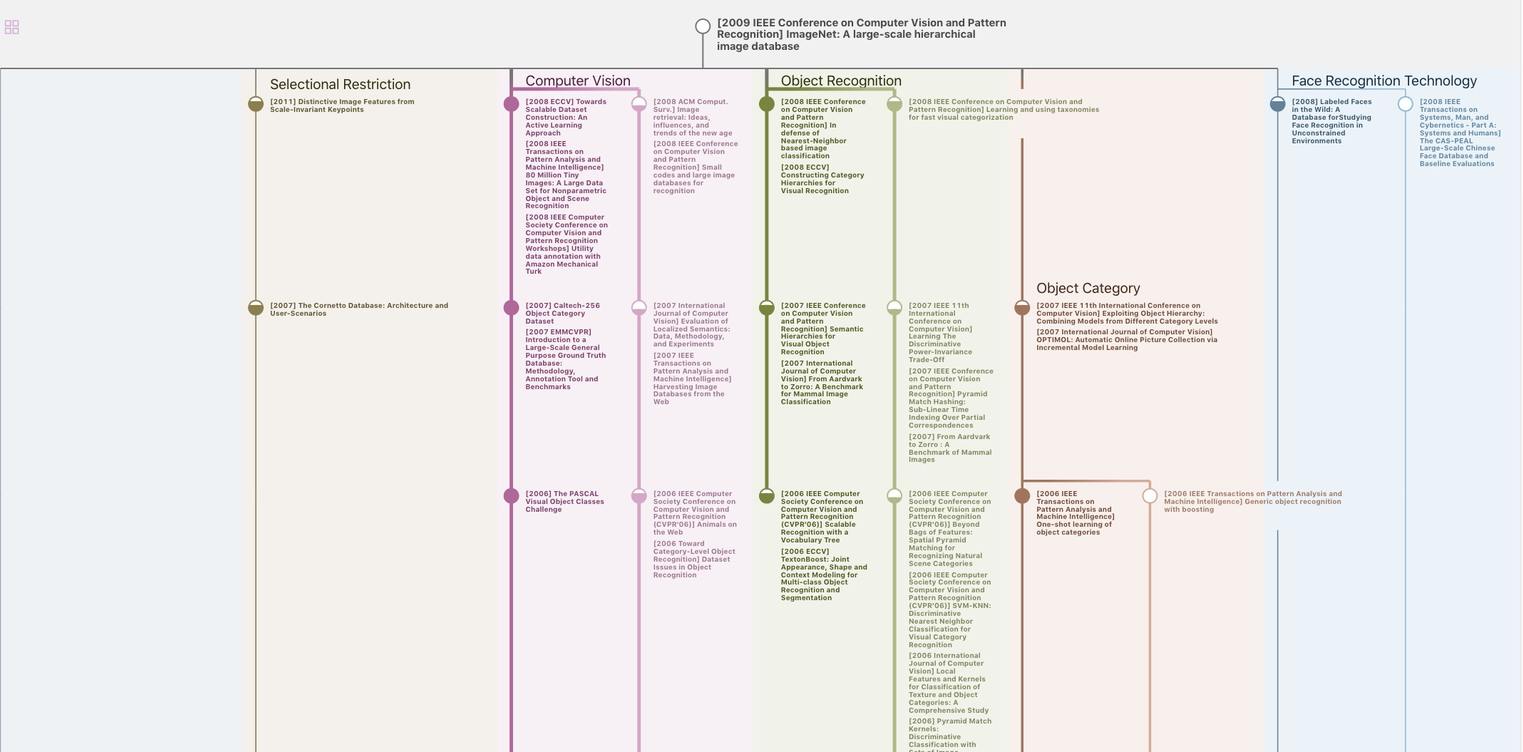
生成溯源树,研究论文发展脉络
Chat Paper
正在生成论文摘要