Doctor for Machines: A Failure Pattern Analysis Solution for Industry 4.0
2020 IEEE INTERNATIONAL CONFERENCE ON BIG DATA (BIG DATA)(2020)
摘要
The success of IoT depends on our ability to solve challenging problems which were previously infeasible. One of the most critical challenges in IoT space is the preventive maintenance in industrial manufacturing processes to maximize equipment availability and durability. Traditionally, preventive management only follows less cost-effective strategies, say time or usage based management. With large amount sensor data harvested from IoT, we can develop much more intelligent predictive maintenance based on accurate machinery failure prediction. In this paper, we develop a framework named DoM (Doctor for Machines) to produce the best predictive model for several oil and gas industry engagements. Our framework is built in the form of pipeline that allows us to generate multiple models simultaneously with parallel computing. We configure the modeling process by assigned different machine learning tasks as such sampling, feature extraction, modeling, and post-processing into the pipeline. The pipeline forms a machine learning graph workflow. With the automation of execution all the tasks in the workflow, the client can easily choose a best predictive model fitting their failure tolerance. We have successfully applied DoM to six data sets, and identified valuables insights on the best practices of creating predictive model. Our clients have successfully achieved financial benefits by applying DoM to develop predictive maintenance schemes to their expensive equipment.
更多查看译文
关键词
IoT,preventive maintenance,industrial manufacturing processes,durability,cost-effective strategies,usage based management,sensor data,intelligent predictive maintenance,DoM,oil-gas industry,pipeline forms,Industry 4.0,machinery failure pattern analysis solution,parallel computing,machine learning graph workflow,feature extraction
AI 理解论文
溯源树
样例
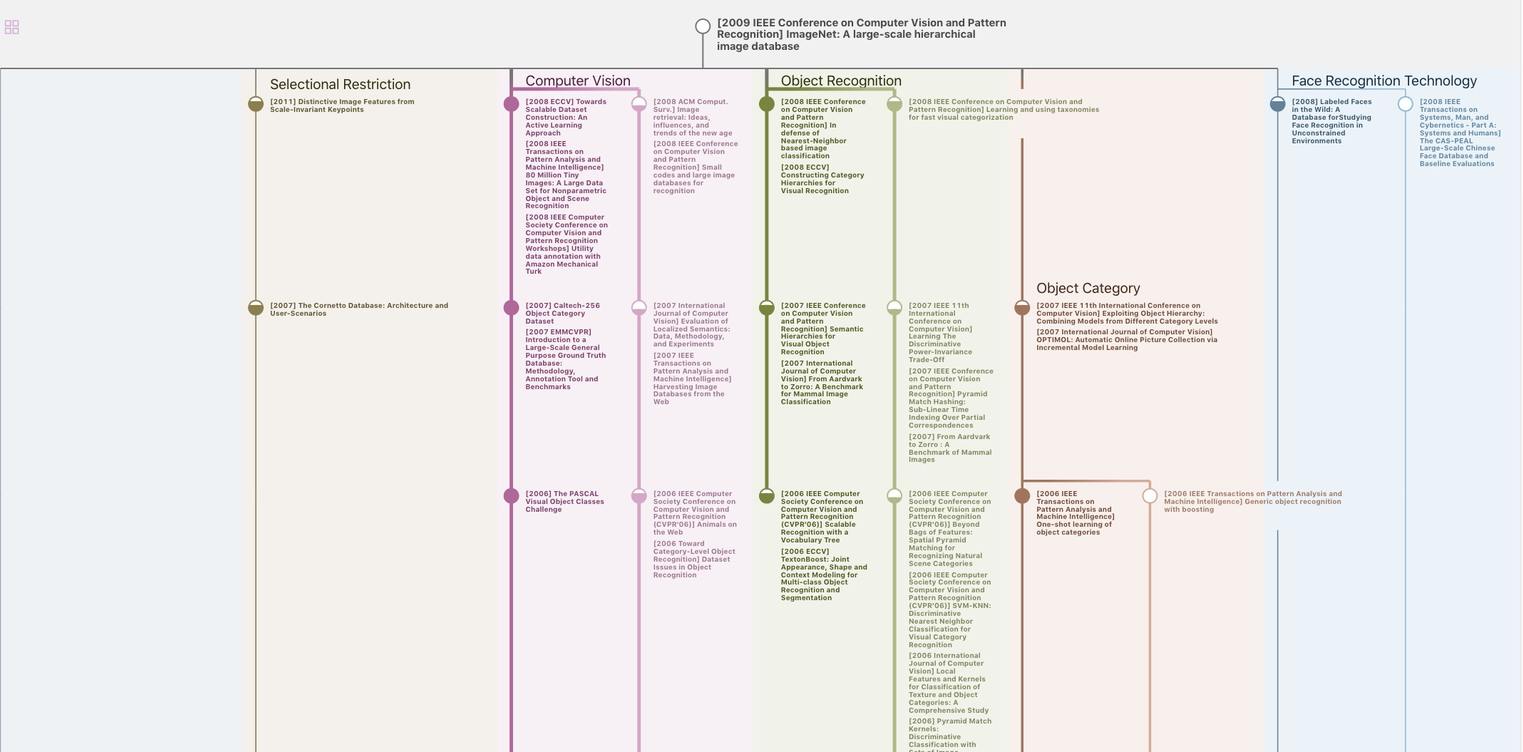
生成溯源树,研究论文发展脉络
Chat Paper
正在生成论文摘要