Flops: On Learning Important Time Series Features For Real-Valued Prediction
2020 IEEE INTERNATIONAL CONFERENCE ON BIG DATA (BIG DATA)(2020)
摘要
Time series value forecasting using machine learning models utilizing time series features has recently got good attention of Time series analytics community. This paper proposes an automated feature learning mechanisms to filter out most useful features from hundreds of available features for time series prediction problems. The paper further proposes a novel mechanism to dynamically filter features that are most suitable for the given input time series data. With such mechanisms we create pipeline consisting of most useful features for given input data and increases the performance of the prediction model. Our proposed mechanism first, groups well known features for time series analysis, generates and assigns the features importance score using multiple scoring configurations. Once scores are assigned, features are filtered using a threshold that is derived using reference feature score and Critical Difference diagram. The filtered features are subsequently analyzed based on the characteristics of the input dataset. We show using experimental results that our approach of input data based dynamic feature selection improves the overall performance of machine learning models compared to the case where dynamic feature extraction is not applied prior to modeling.
更多查看译文
关键词
time series analysis,reference feature score,filtered features,dynamic feature selection,machine learning models,dynamic feature extraction,time series features,real-valued prediction,automated feature,time series prediction problems,input time series data,prediction model,time series analytics community,time series value forecasting,scoring configurations,FLOps,critical difference diagram
AI 理解论文
溯源树
样例
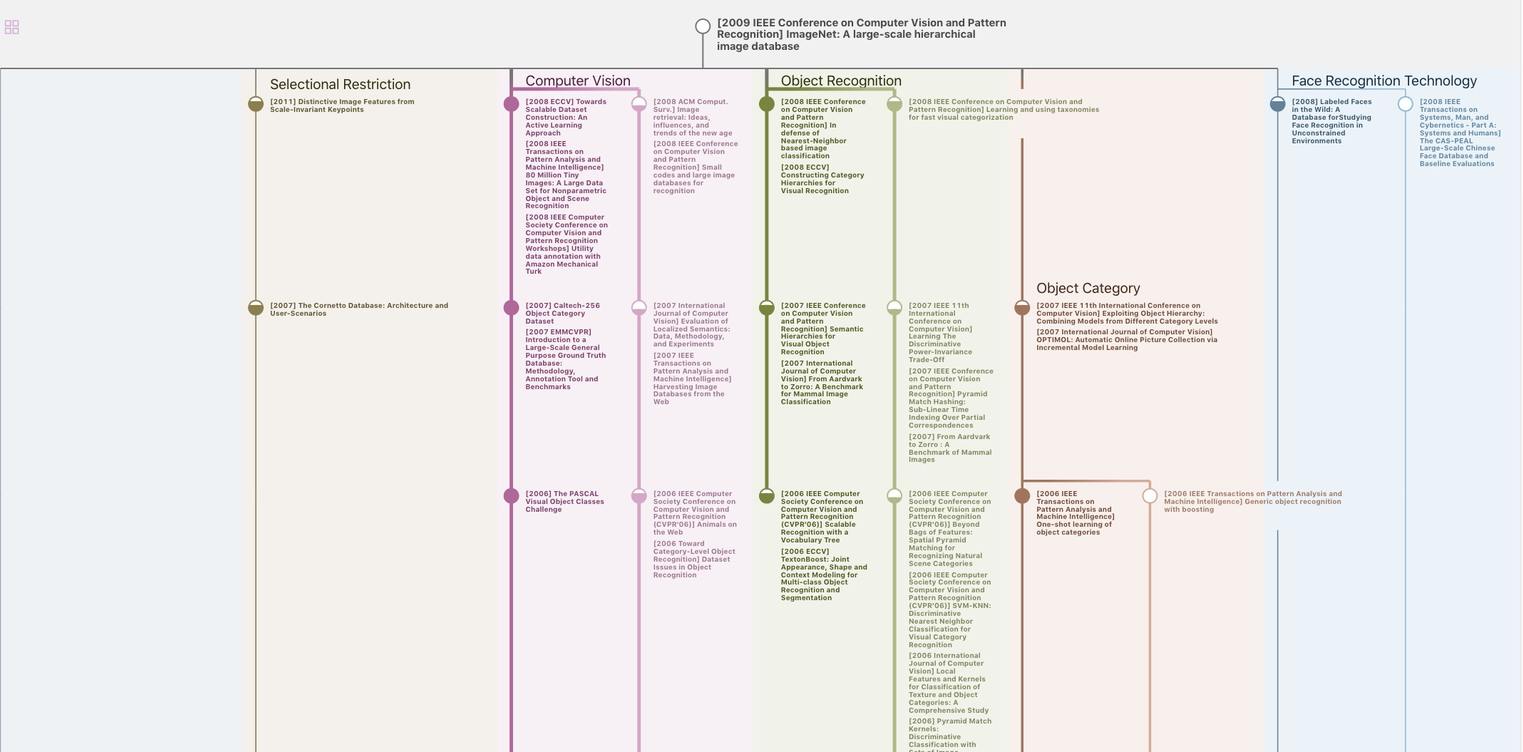
生成溯源树,研究论文发展脉络
Chat Paper
正在生成论文摘要