Statistical Reasoning Of Zero-Inflated Right-Skewed User-Generated Big Data A/B Testing
2020 IEEE INTERNATIONAL CONFERENCE ON BIG DATA (BIG DATA)(2020)
摘要
A/B testing serves as an ultimate standard for decision making in the technology industry. Compared with extensive studies on machine learning algorithms, system design and user research of relevance related products, like recommender systems, search ranking, etc., there are very few works on discussion of related A/B testing methods. In particular, some very important online KPIs, like user expenditure for online e-commerce and user active minutes for social media or video streaming platforms, typically involve zero-inflated and right-skewed data as a significant number of users may not even engage while a few of them engage heavily. Therefore, a deep understanding of zero-inflated right-skewed data testing methods is crucial. In this paper, we did an extensive and detailed survey on this topic, while we extended several statistical estimators and hypothesis testing methods for zero-inflated right-skewed data as well. We compared these statistical methods both theoretically and empirically in a large sample setting to align with the huge amount of data we collect in industrial A/B testing. Our theoretical analysis and simulation results on both synthetic and Twitter real data challenged the superiority of several methods which claimed with small samples or specific underlying distributions. Moreover, we analyzed two common pitfalls of testing zero-inflated right-skewed data in practice, which helps better decision making with A/B testing on data with such structures.
更多查看译文
关键词
A/B Testing, Statistical Inference, Asymptotic Theory
AI 理解论文
溯源树
样例
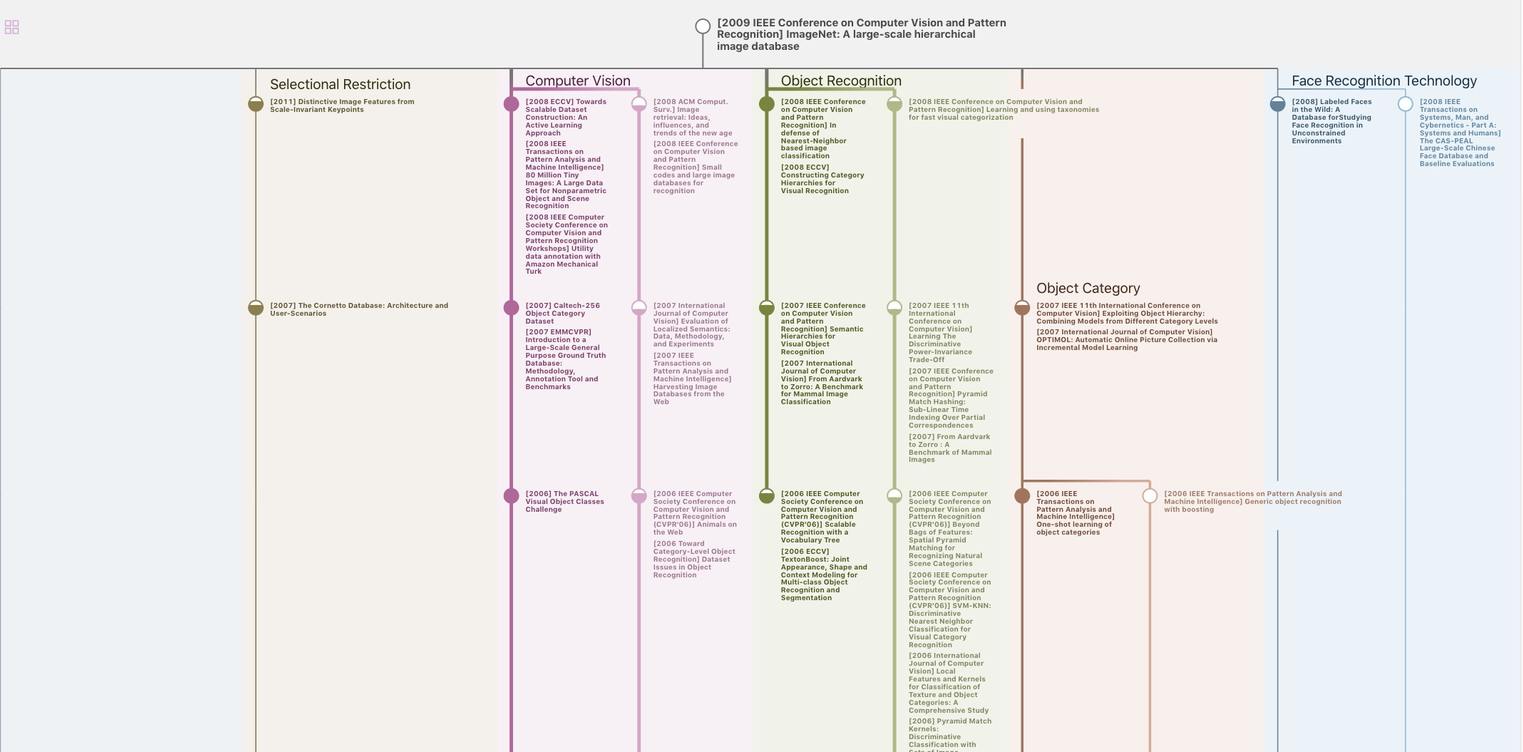
生成溯源树,研究论文发展脉络
Chat Paper
正在生成论文摘要