RAWLSNET: Altering Bayesian Networks to Encode Rawlsian Fair Equality of Opportunity
AIES '21: PROCEEDINGS OF THE 2021 AAAI/ACM CONFERENCE ON AI, ETHICS, AND SOCIETY(2021)
摘要
We present RAWLSNET, a system for altering Bayesian Network (BN) models to satisfy the Rawlsian principle of fair equality of opportunity (FEO). RAWLSNET's BN models generate aspirational data distributions: data generated to reflect an ideally fair, FEO-satisfying society. FEO states that everyone with the same talent and willingness to use it should have the same chance of achieving advantageous social positions (e.g., employment), regardless of their background circumstances (e.g., socioeconomic status). Satisfying FEO requires alterations to social structures such as school assignments. Our paper describes RAWLSNET, a method which takes as input a BN representation of an FEO application and alters the BN's parameters so as to satisfy FEO when possible, and minimize deviation from FEO otherwise. We also offer guidance for applying RAWLSNET, including on recognizing proper applications of FEO. We demonstrate the use of RAWLSNET with publicly available data sets. RAWLSNET's altered BNs offer the novel capability of generating aspirational data for FEO-relevant tasks. Aspirational data are free from biases of real-world data, and thus are useful for recognizing and detecting sources of unfairness in machine learning algorithms besides biased data.
更多查看译文
关键词
Rawlsian fair equality of opportunity, Bayesian networks, aspirational data
AI 理解论文
溯源树
样例
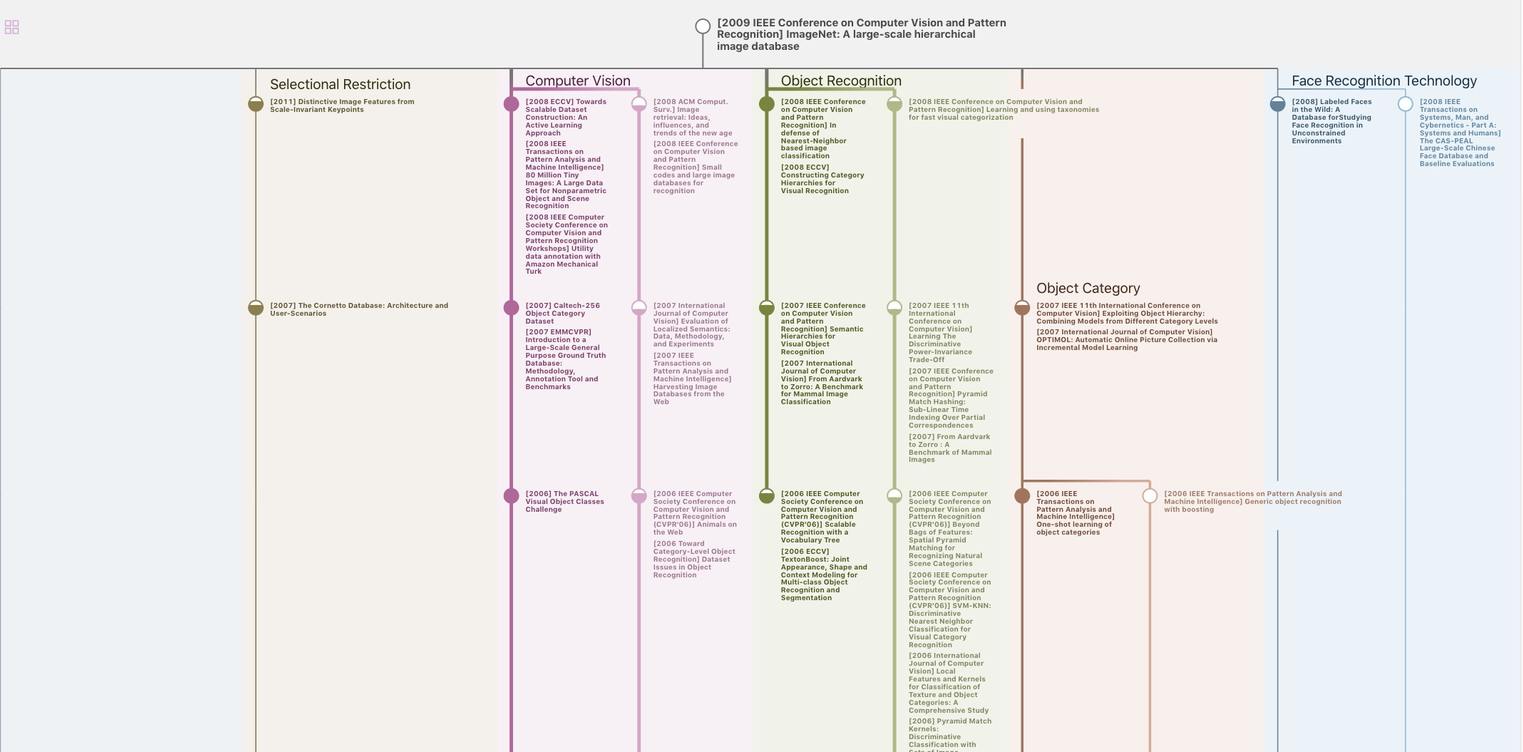
生成溯源树,研究论文发展脉络
Chat Paper
正在生成论文摘要