ORBIT - A Real-World Few-Shot Dataset for Teachable Object Recognition.
ICCV(2021)
摘要
Object recognition has made great advances in the last decade, but predominately still relies on many high-quality training examples per object category. In contrast, learning new objects from only a few examples could enable many impactful applications from robotics to user personalization. Most few-shot learning research, however, has been driven by benchmark datasets that lack the high variation that these applications will face when deployed in the real-world. To close this gap, we present the ORBIT dataset and benchmark, grounded in a real-world application of teachable object recognizers for people who are blind/low vision. The dataset contains 3,822 videos of 486 objects recorded by people who are blind/low-vision on their mobile phones, and the benchmark reflects a realistic, highly challenging recognition problem, providing a rich playground to drive research in robustness to few-shot, high-variation conditions. We set the first state-of-the-art on the benchmark and show that there is massive scope for further innovation, holding the potential to impact a broad range of real-world vision applications including tools for the blind/low-vision community. The dataset and benchmark code can be accessed at https://github.com/microsoft/ORBIT-Dataset.
更多查看译文
关键词
Datasets and evaluation,Transfer/Low-shot/Semi/Unsupervised Learning,Vision applications and systems
AI 理解论文
溯源树
样例
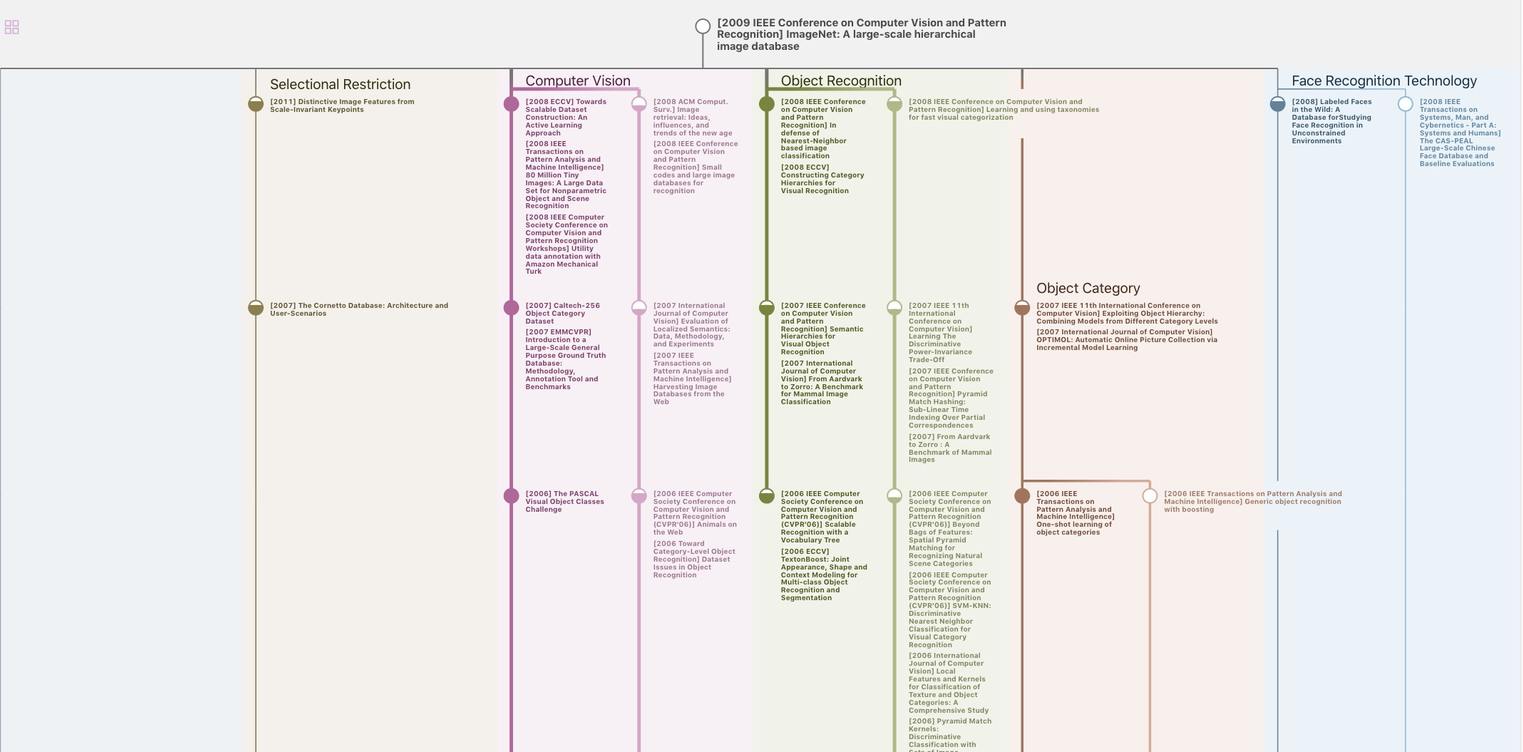
生成溯源树,研究论文发展脉络
Chat Paper
正在生成论文摘要