Data silos are undermining drug development and failing rare disease patients
ORPHANET JOURNAL OF RARE DISEASES(2021)
摘要
Data silos are proliferating while research and development activity explode following genetic and immunological advances for many clinically described disorders with previously unknown etiologies. The latter event has inspired optimism in the patient, clinical, and research communities that disease-specific treatments are on the way. However, we fear the tendency of various stakeholders to balkanize databases in proprietary formats, driven by current economic and academic incentives, will inevitably fragment the expanding knowledge base and undermine current and future research efforts to develop much-needed treatments. The proliferation of proprietary databases, compounded by a paucity of meaningful outcome measures and/or good natural history data, slows our ability to generate scalable solutions to benefit chronically underserved patient populations in ways that would translate to more common diseases. The current research and development landscape sets too many projects up for unnecessary failure, particularly in the rare disease sphere, and does a grave disservice to highly vulnerable patients. This system also encourages the collection of redundant data in uncoordinated parallel studies and registries to ultimately delay or deny potential treatments for ostensibly tractable diseases; it also promotes the waste of precious time, energy, and resources. Groups at the National Institutes of Health and Food and Drug Administration have started programs to address these issues. However, we and many others feel there should be significantly more discussion of how to coordinate and scale registry efforts. Such discourse aims to reduce needless complexity and duplication of efforts, as well as promote a pre-competitive knowledge ecosystem for rare disease drug development that cultivates and accelerates innovation.
更多查看译文
关键词
Rare diseases, Drug development, Knowledge bases, Registries, Research design, Data management
AI 理解论文
溯源树
样例
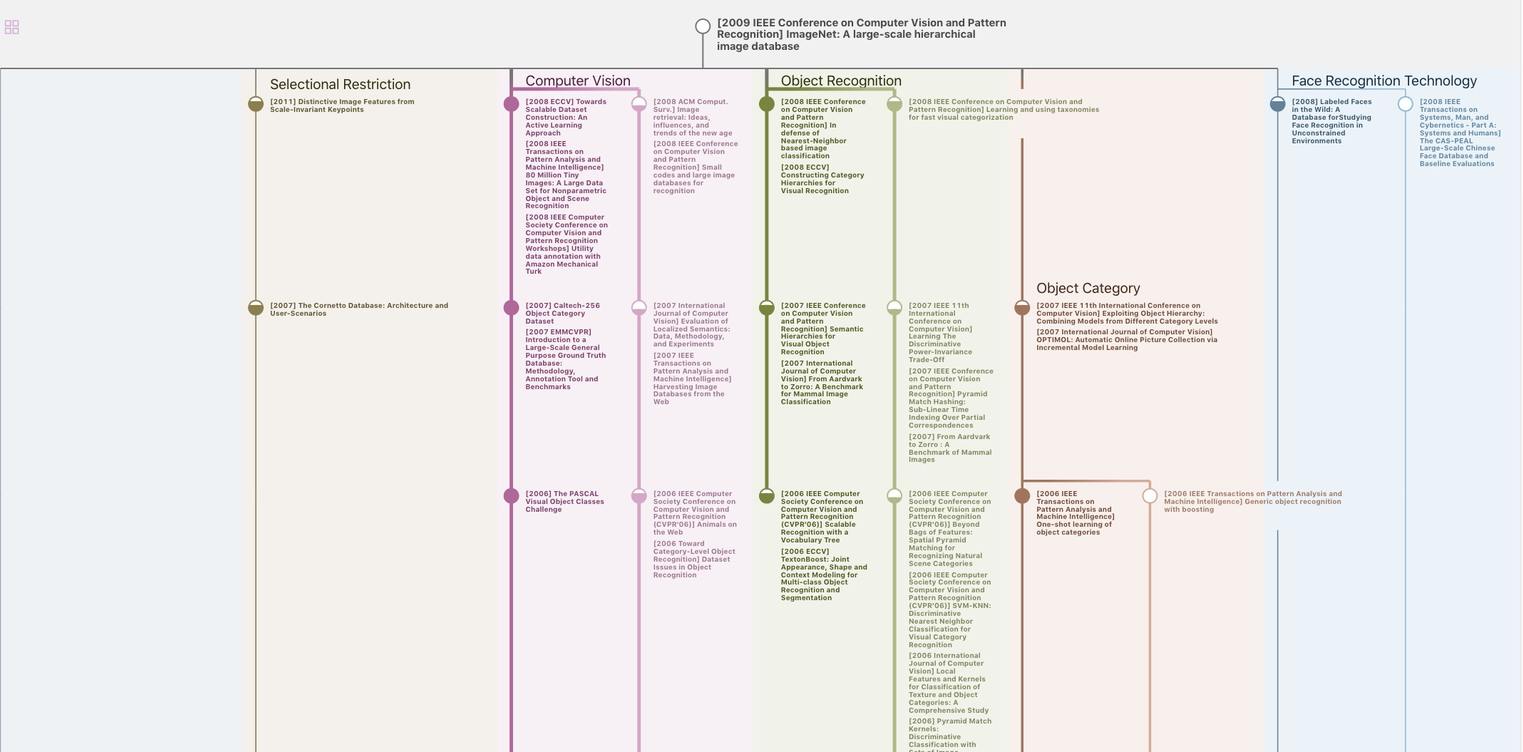
生成溯源树,研究论文发展脉络
Chat Paper
正在生成论文摘要