A Novel Automatic Morphologic Analysis of Eyelids Based on Deep Learning Methods
CURRENT EYE RESEARCH(2021)
摘要
Purpose: To propose a deep-learning-based approach to automatically and objectively evaluate morphologic eyelid features using two-dimensional(2D) digital photographs and to assess the agreement between automatic and manual measurements. Materials and Methods: The 2D photographs of 1378 normal Asian participants (2756 eyes) were included for training, validating and testing the cornea and eyelid segmentation network. Margin reflex distance 1 (MRD1) and margin reflex distance 2 (MRD2) of 406 eyes from 203 participants were manually evaluated by 3 ophthalmologists and the photographs of 406 eyes were measured automatically for 8 morphologic eyelid features. The Spearman's correlation coefficient, intra-class correlation coefficient (ICC) and Bland-Altman analyses were used to determine the agreement between manual and automatic MRDs. Results: The dice coefficient was 0.922 and 0.974 for eyelid and cornea segmentation, respectively. A strong correlation was shown between manually and automatically measured MRD1 (r = 0.993, ICC = 0.996) and MRD2 (r = 0.950, ICC = 0.974). Bland-Altman analyses also showed excellent reliability with bias being 0.04 mmbetween automated and manual MRD1 measurements and 0.06 mm for MRD2. Automatically measured 8 features (MRD1, MRD2, palpebral fissure, medial area, lateral area, cornea area, upper and lower eyelid lengths) were found to be increased with age and peaked around the age range of 21 to 30 years. Conclusions: The proposed novel integrative analysis scheme was comparable with human performance. The approach with excellent reliability and reproductivity showed great potential for automated diagnosis and remote monitoring of eyelid-related diseases.
更多查看译文
关键词
Eyelid, deep learning, morphologic features, digital images, automatic analysis
AI 理解论文
溯源树
样例
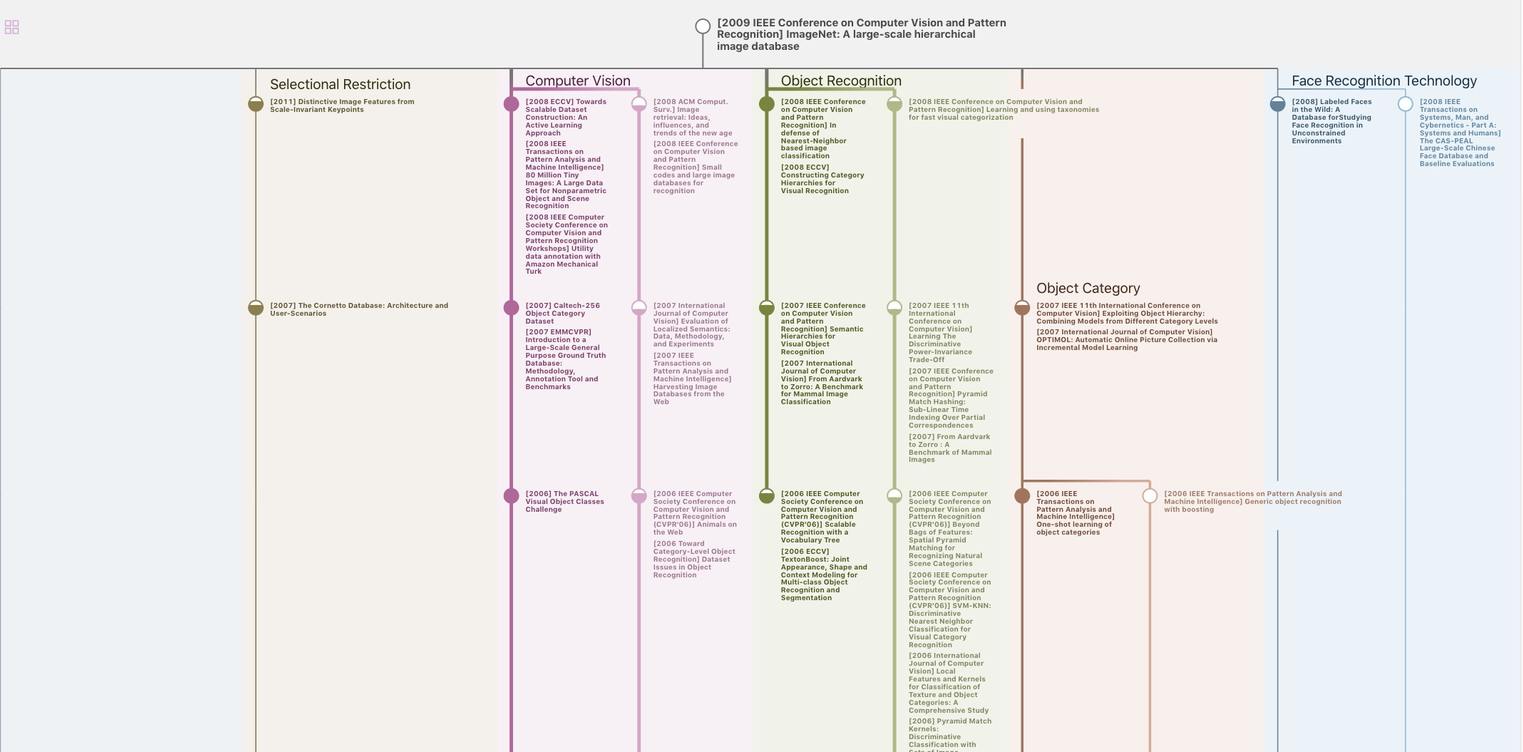
生成溯源树,研究论文发展脉络
Chat Paper
正在生成论文摘要