Nanosecond Machine Learning Event Classification With Boosted Decision Trees In Fpga For High Energy Physics
JOURNAL OF INSTRUMENTATION(2021)
摘要
We present a novel implementation of classification using the machine learning/artificial intelligence method called boosted decision trees (BDT) on field programmable gate arrays (FPGA). The firmware implementation of binary classification requiring 100 training trees with a maximum depth of 4 using four input variables gives a latency value of about 10 ns, independent of the clock speed from 100 to 320 MHz in our setup. The low timing values are achieved by restructuring the BDT layout and reconfiguring its parameters. The FPGA resource utilization is also kept low at a range from 0.01% to 0.2% in our setup. A software package called FWXNACHINA achieves this implementation. Our intended user is an expert in custom electronics-based trigger systems in high energy physics experiments or anyone that needs decisions at the lowest latency values for real-time event classification. Two problems from high energy physics are considered, in the separation of electrons vs. photons and in the selection of vector boson fusion-produced Higgs bosons vs. the rejection of the multijet processes.
更多查看译文
关键词
Digital electronic circuits, Trigger algorithms, Trigger concepts and systems (hardware and software), Data reduction methods
AI 理解论文
溯源树
样例
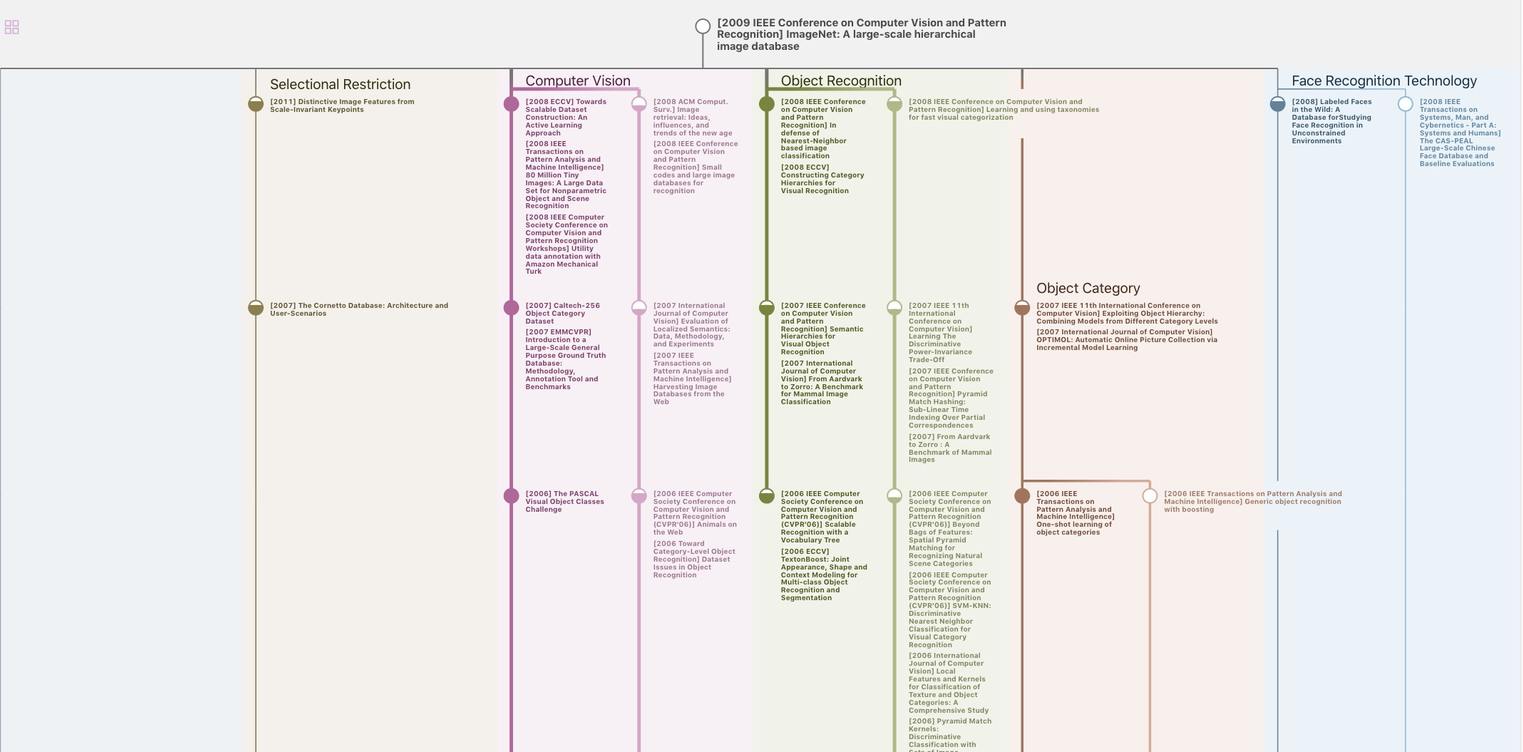
生成溯源树,研究论文发展脉络
Chat Paper
正在生成论文摘要