Dense Dilated UNet: Deep Learning for 3D Photoacoustic Tomography Image Reconstruction
arxiv(2021)
摘要
In photoacoustic tomography (PAT), the acoustic pressure waves produced by optical excitation are measured by an array of detectors and used to reconstruct an image. Sparse spatial sampling and limited-view detection are two common challenges faced in PAT. Reconstructing from incomplete data using standard methods results in severe streaking artifacts and blurring. We propose a modified convolutional neural network (CNN) architecture termed Dense Dilation UNet (DD-UNet) for correcting artifacts in 3D PAT. The DD-Net leverages the benefits of dense connectivity and dilated convolutions to improve CNN performance. We compare the proposed CNN in terms of image quality as measured by the multiscale structural similarity index metric to the Fully Dense UNet (FD-UNet). Results demonstrate that the DD-Net consistently outperforms the FD-UNet and is able to more reliably reconstruct smaller image features.
更多查看译文
关键词
tomography,dense dilated unet,deep learning
AI 理解论文
溯源树
样例
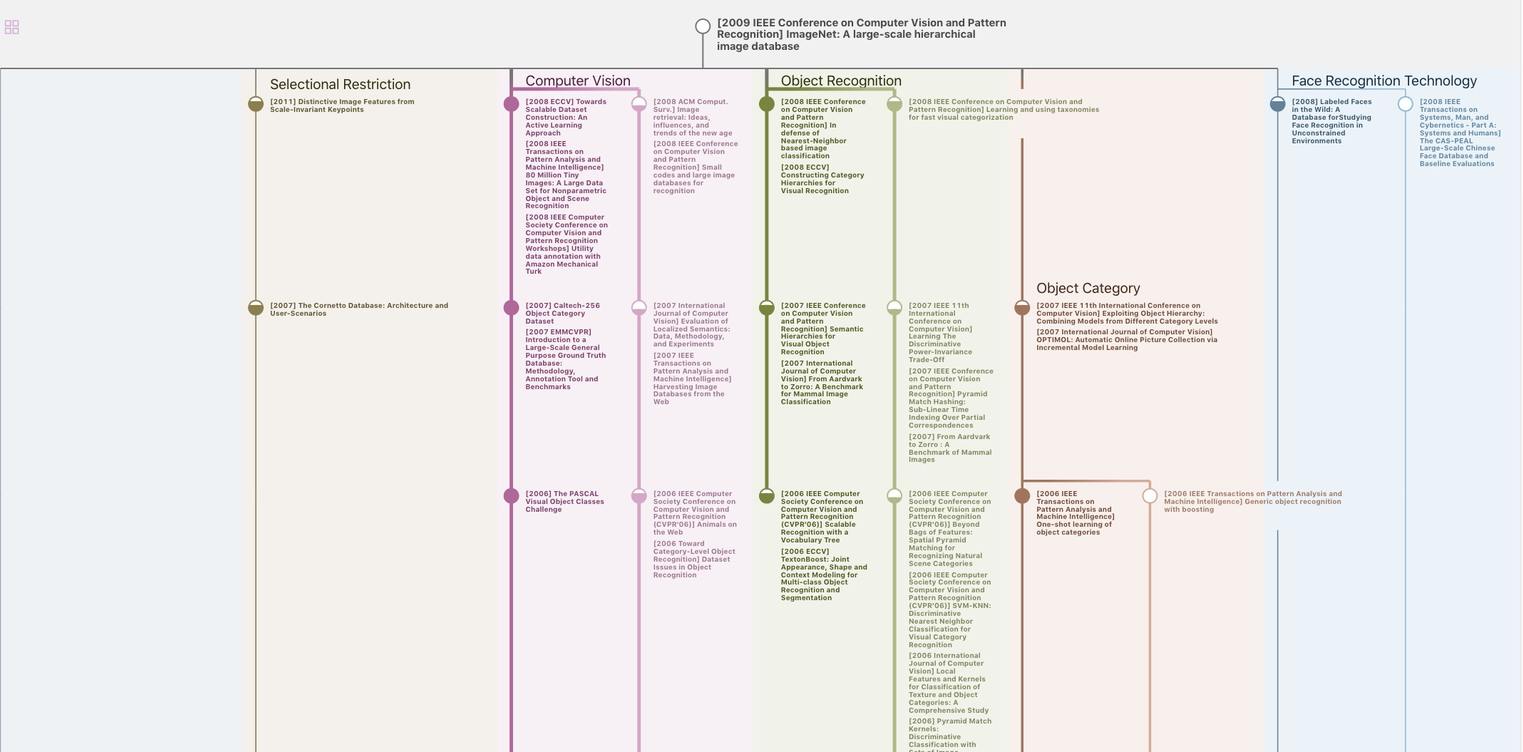
生成溯源树,研究论文发展脉络
Chat Paper
正在生成论文摘要