deepregression: A Flexible Neural Network Framework for Semi-Structured Deep Distributional Regression
ArXiv(2023)
摘要
In this paper we describe the implementation of semi-structured deep distributional re-gression, a flexible framework to learn conditional distributions based on the combination of additive regression models and deep networks. Our implementation encompasses (1) a modular neural network building system based on the deep learning library TensorFlow for the fusion of various statistical and deep learning approaches, (2) an orthogonaliza-tion cell to allow for an interpretable combination of different subnetworks, as well as (3) pre-processing steps necessary to set up such models. The software package allows to define models in a user-friendly manner via a formula interface that is inspired by classical statistical model frameworks such as mgcv. The package's modular design and function-ality provides a unique resource for both scalable estimation of complex statistical models and the combination of approaches from deep learning and statistics. This allows for state-of-the-art predictive performance while simultaneously retaining the indispensable interpretability of classical statistical models.
更多查看译文
关键词
additive predictors,deep learning,effect decomposition,orthogonal complement,penalization,smoothing
AI 理解论文
溯源树
样例
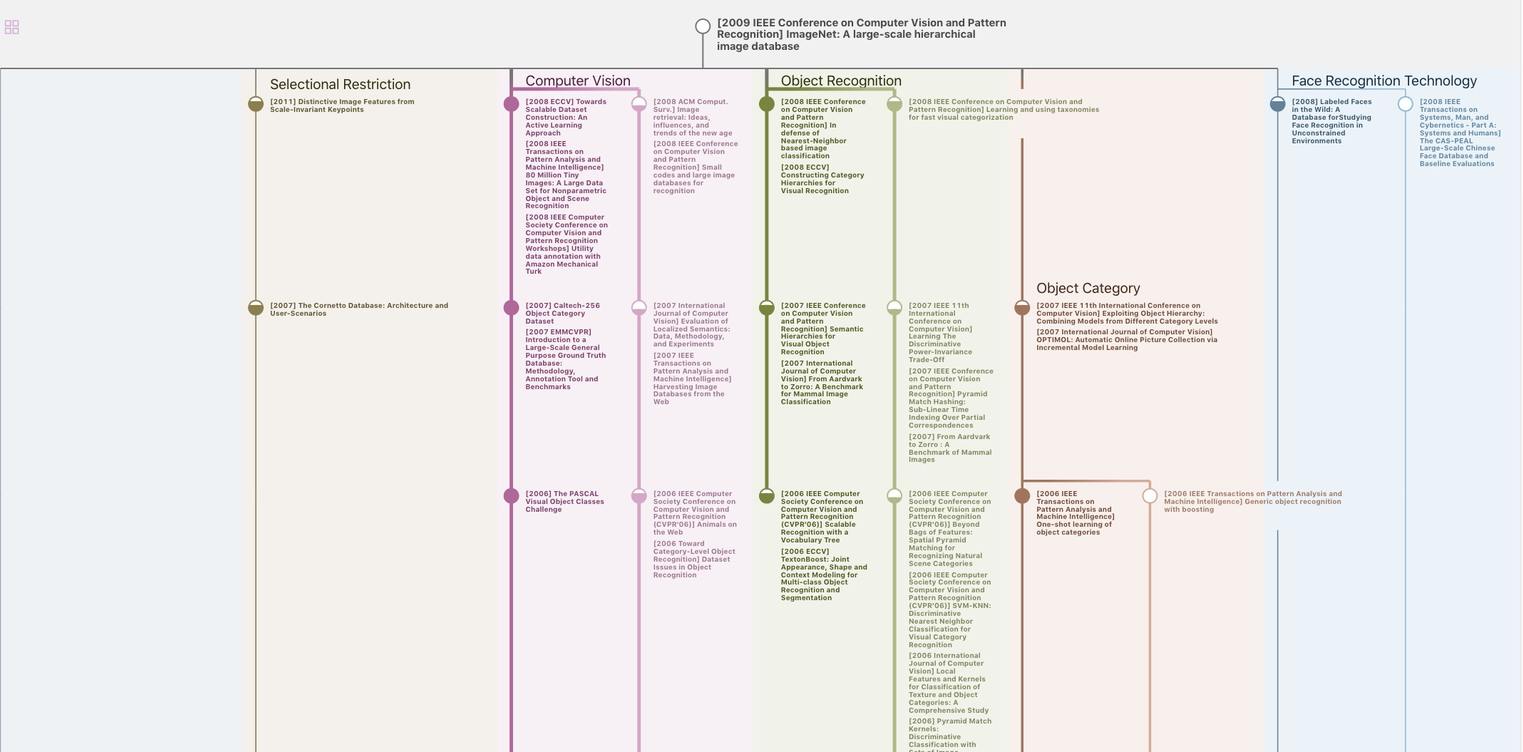
生成溯源树,研究论文发展脉络
Chat Paper
正在生成论文摘要