Pathological Image Segmentation with Noisy Labels
arxiv(2021)
摘要
Segmentation of pathological images is essential for accurate disease diagnosis. The quality of manual labels plays a critical role in segmentation accuracy; yet, in practice, the labels between pathologists could be inconsistent, thus confusing the training process. In this work, we propose a novel label re-weighting framework to account for the reliability of different experts' labels on each pixel according to its surrounding features. We further devise a new attention heatmap, which takes roughness as prior knowledge to guide the model to focus on important regions. Our approach is evaluated on the public Gleason 2019 datasets. The results show that our approach effectively improves the model's robustness against noisy labels and outperforms state-of-the-art approaches.
更多查看译文
关键词
pathological image segmentation,labels
AI 理解论文
溯源树
样例
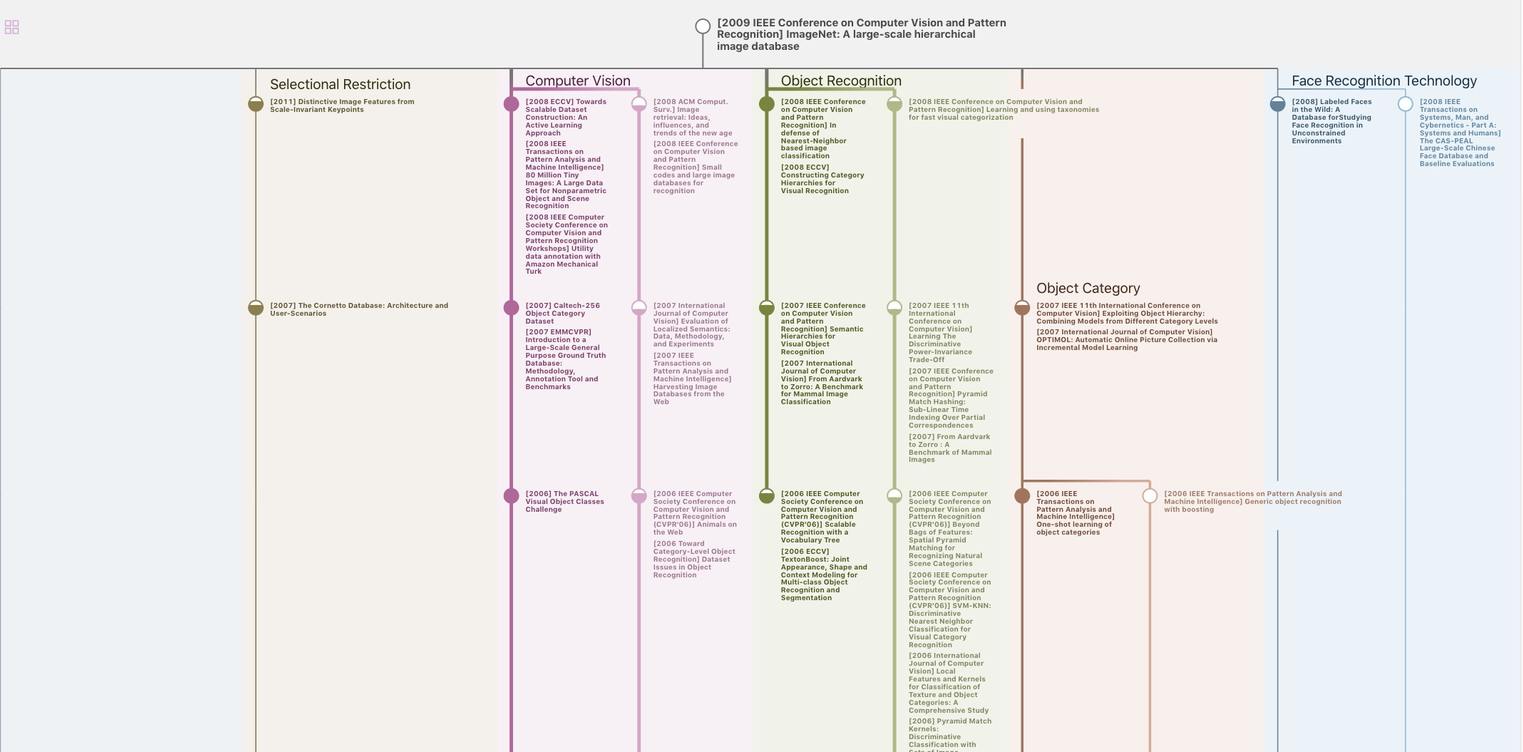
生成溯源树,研究论文发展脉络
Chat Paper
正在生成论文摘要