Variational Transformer Networks for Layout Generation
2021 IEEE/CVF CONFERENCE ON COMPUTER VISION AND PATTERN RECOGNITION, CVPR 2021(2021)
摘要
Generative models able to synthesize layouts of different kinds (e.g. documents, user interfaces or furniture arrangements) are a useful tool to aid design processes and as a first step in the generation of synthetic data, among other tasks. We exploit the properties of self-attention layers to capture high level relationships between elements in a layout, and use these as the building blocks of the well-known Variational Autoencoder (VAE) formulation. Our proposed Variational Transformer Network (VTN) is capable of learning margins, alignments and other global design rules without explicit supervision. Layouts sampled from our model have a high degree of resemblance to the training data, while demonstrating appealing diversity. In an extensive evaluation on publicly available benchmarks for different layout types VTNs achieve state-of-the-art diversity and perceptual quality. Additionally, we show the capabilities of this method as part of a document layout detection pipeline.
更多查看译文
关键词
training data,document layout detection pipeline,layout generation,generative models,user interfaces,furniture arrangements,synthetic data,self-attention layers,high level relationships,building blocks,global design rules,variational transformer network,variational autoencoder formulation,variational transformer networks,layout types VTN
AI 理解论文
溯源树
样例
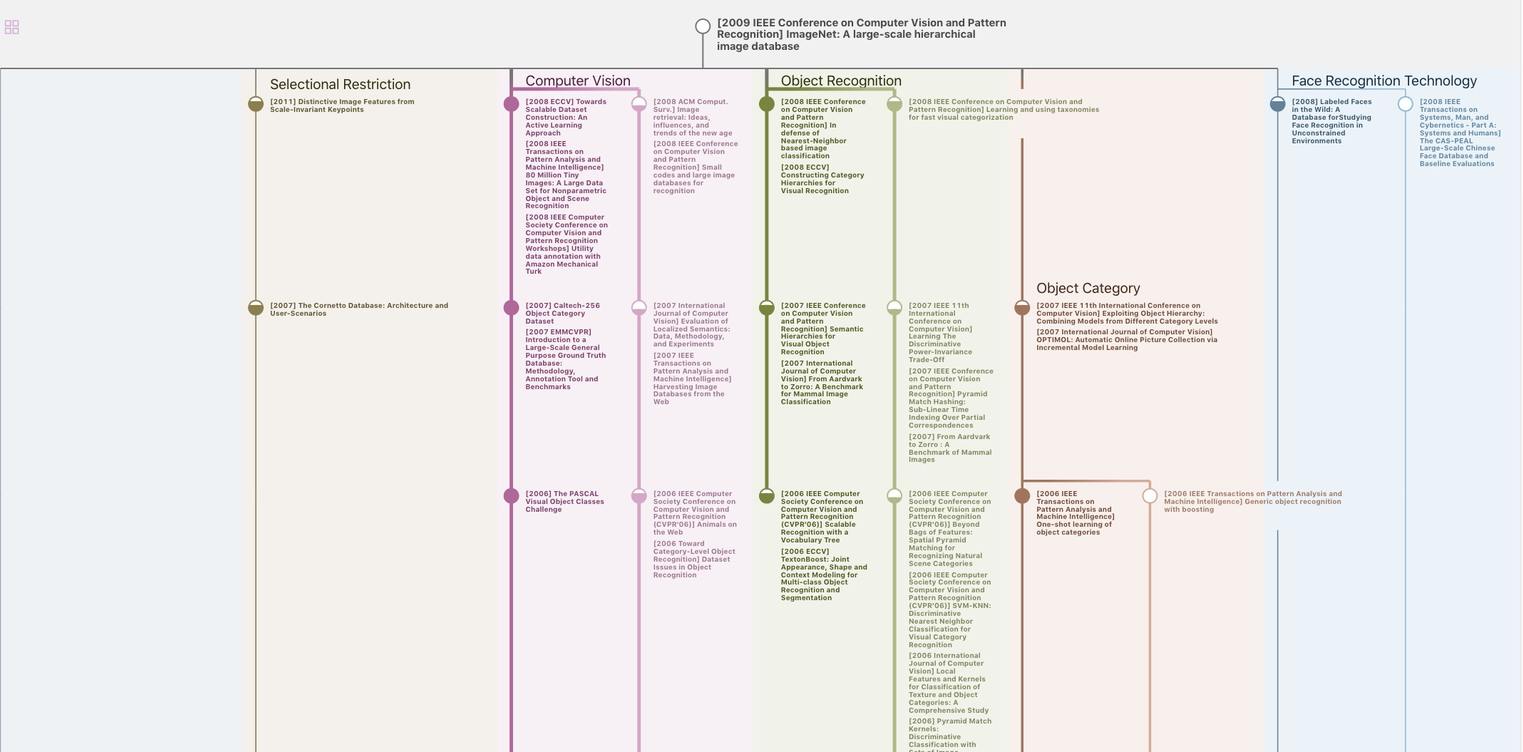
生成溯源树,研究论文发展脉络
Chat Paper
正在生成论文摘要