Improving the Expressive Power of Graph Neural Network with Tinhofer Algorithm
arXiv (Cornell University)(2021)
Abstract
In recent years, Graph Neural Network (GNN) has bloomly progressed for its power in processing graph-based data. Most GNNs follow a message passing scheme, and their expressive power is mathematically limited by the discriminative ability of the Weisfeiler-Lehman (WL) test. Following Tinhofer's research on compact graphs, we propose a variation of the message passing scheme, called the Weisfeiler-Lehman-Tinhofer GNN (WLT-GNN), that theoretically breaks through the limitation of the WL test. In addition, we conduct comparative experiments and ablation studies on several well-known datasets. The results show that the proposed methods have comparable performances and better expressive power on these datasets.
MoreTranslated text
Key words
graph neural network,tinhofer algorithm,neural network
AI Read Science
Must-Reading Tree
Example
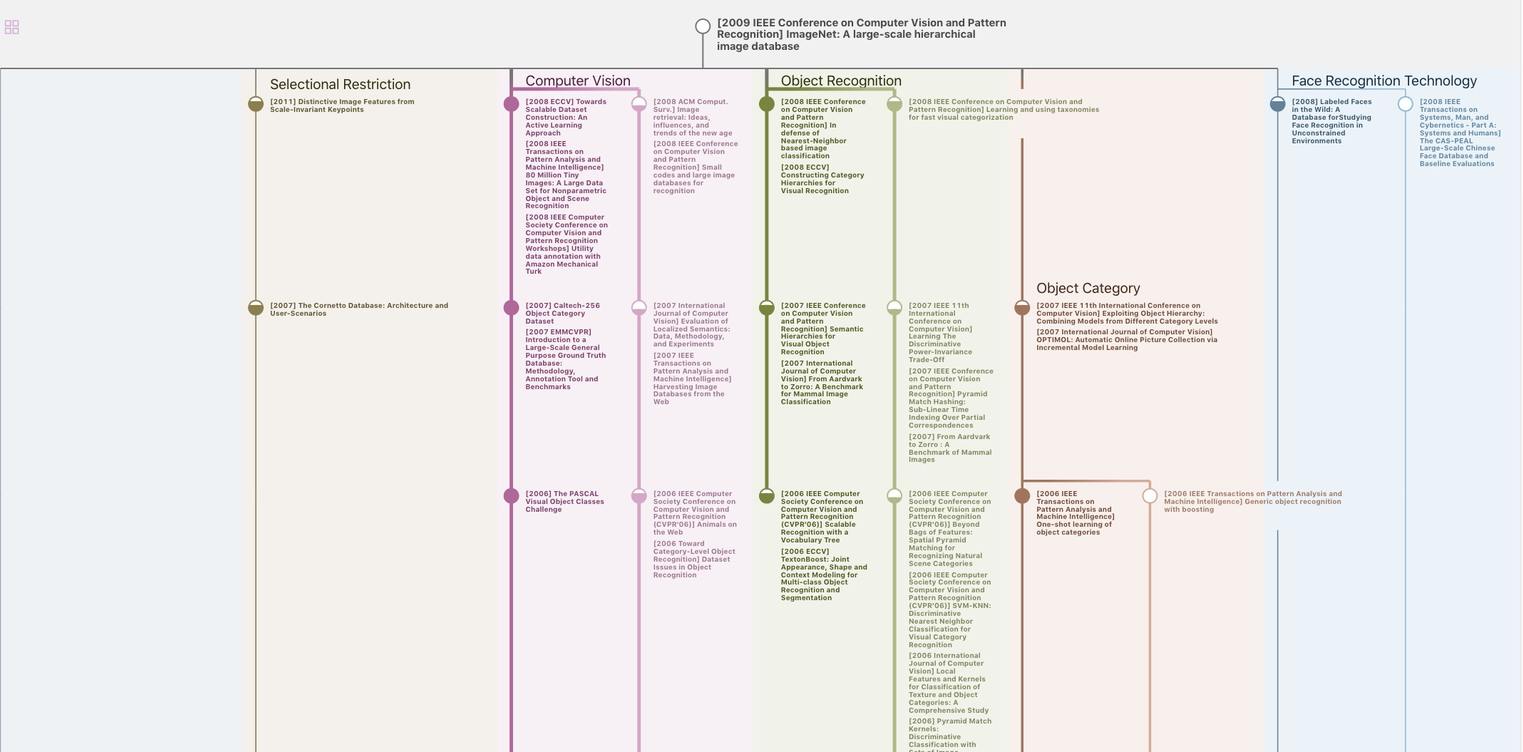
Generate MRT to find the research sequence of this paper
Chat Paper
Summary is being generated by the instructions you defined