Class-incremental Learning using a Sequence of Partial Implicitly Regularized Classifiers.
International Florida Artificial Intelligence Research Society Conference (FLAIRS)(2022)
摘要
In class-incremental learning, the objective is to learn a number of classes sequentially without having access to the whole training data. However, due to a problem known as catastrophic forgetting, neural networks suffer substantial performance drop in such settings. The problem is often approached by experience replay, a method which stores a limited number of samples to be replayed in future steps to reduce forgetting of the learned classes. When using a pretrained network as a feature extractor, we show that instead of training a single classifier incrementally, it is better to train a number of specialized classifiers which do not interfere with each other yet can cooperatively predict a single class. Our experiments on CIFAR100 dataset show that the proposed method improves the performance over SOTA by a large margin.
更多查看译文
关键词
classifiers,learning,partial implicitly
AI 理解论文
溯源树
样例
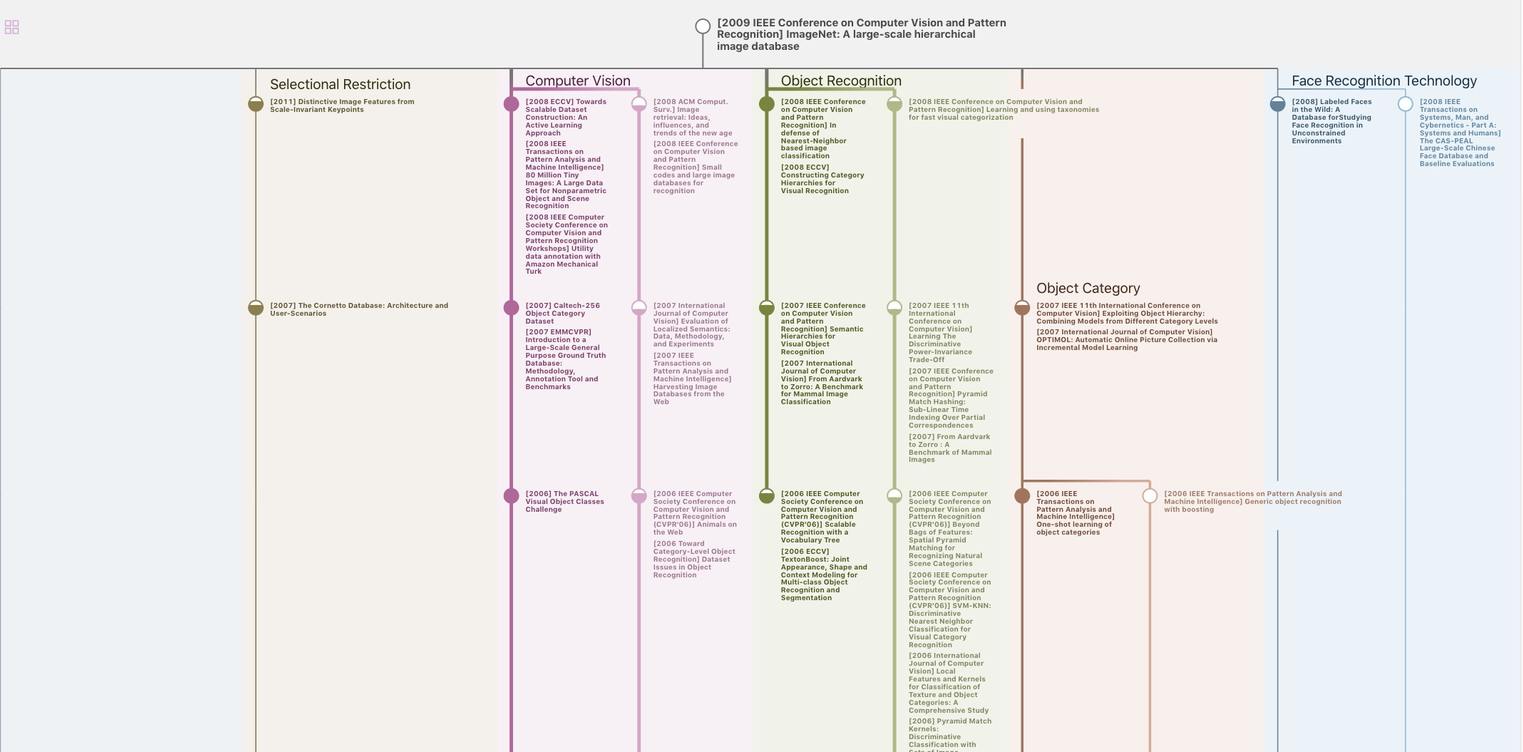
生成溯源树,研究论文发展脉络
Chat Paper
正在生成论文摘要