Fine-grained image classification model based on improved SqueezeNet
2021 IEEE 5th Advanced Information Technology, Electronic and Automation Control Conference (IAEAC)(2021)
Abstract
In order to solve the problem that some fine-grained algorithms have a large amount of calculation and low operating efficiency, This paper firstly proposes the use of SqueezeNet for fine-grained image recognition tasks, because SqueezeNet, as one of the representatives of lightweight CNN, has fewer parameters and faster running time. The experimental verification of SqueezeNet on three fine-grained datasets shows that SqueezeNet can also finely recognize images, but the classification accuracy rate is low, this paper further improves SqueezeNet: two different attention mechanism modules are embedded in the SqueezeNet in different ways, then use bilinear fusion to fuse the attention feature maps, and then the new attention feature map is bilinearly fused with the last layer of the network, a new tensor is obtained and sent to the linear layer for classification finally. Through experimental comparison and visual analysis, the improved SqueezeNet has a significant improvement in accuracy compared to the original network and other algorithms.
MoreTranslated text
Key words
Visualization,Image recognition,Tensors,Memory management,Task analysis,Kernel,Image classification
AI Read Science
Must-Reading Tree
Example
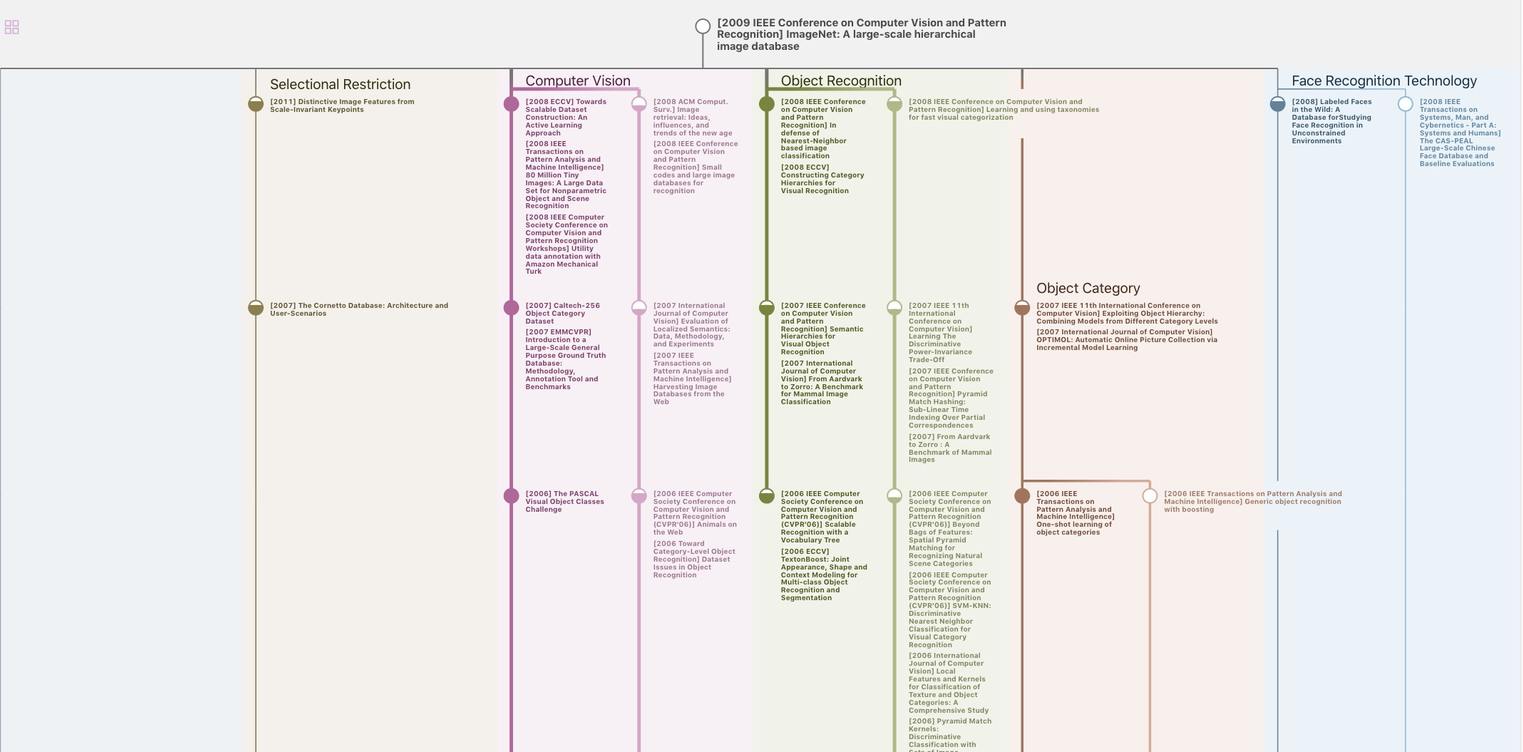
Generate MRT to find the research sequence of this paper
Chat Paper
Summary is being generated by the instructions you defined