Computer-Aided Segmentation and Machine Learning of Integrated Clinical and Diffusion-Weighted Imaging Parameters for Predicting Lymph Node Metastasis in Endometrial Cancer
CANCERS(2021)
Abstract
Simple Summary Computer-aided segmentation and machine learning added values of clinical parameters and diffusion-weighted imaging radiomics for predicting nodal metastasis in endometrial cancer, with a diagnostic performance superior to criteria based on lymph node size or apparent diffusion coefficient. Precise risk stratification in lymphadenectomy is important for patients with endometrial cancer (EC), to balance the therapeutic benefit against the operation-related morbidity and mortality. We aimed to investigate added values of computer-aided segmentation and machine learning based on clinical parameters and diffusion-weighted imaging radiomics for predicting lymph node (LN) metastasis in EC. This prospective observational study included 236 women with EC (mean age +/- standard deviation, 51.2 +/- 11.6 years) who underwent magnetic resonance (MR) imaging before surgery during July 2010-July 2018, randomly split into training (n = 165) and test sets (n = 71). A decision-tree model was constructed based on mean apparent diffusion coefficient (ADC) value of the tumor (cutoff, 1.1 x 10(-3) mm(2)/s), skewness of the relative ADC value (cutoff, 1.2), short-axis diameter of LN (cutoff, 1.7 mm) and skewness ADC value of the LN (cutoff, 7.2 x 10(-2)), as well as tumor grade (1 vs. 2 and 3), and clinical tumor size (cutoff, 20 mm). The sensitivity and specificity of the model were 94% and 80% for the training set and 86%, 78% for the independent testing set, respectively. The areas under the receiver operating characteristics curve (AUCs) of the decision-tree was 0.85-significantly higher than the mean ADC model (AUC = 0.54) and LN short-axis diameter criteria (AUC = 0.62) (both p < 0.0001). We concluded that a combination of clinical and MR radiomics generates a prediction model for LN metastasis in EC, with diagnostic performance surpassing the conventional ADC and size criteria.
MoreTranslated text
Key words
computer-aided,diffusion-weighted imaging,endometrial cancer,lymph node,metastasis,machine learning,magnetic resonance,radiomics
AI Read Science
Must-Reading Tree
Example
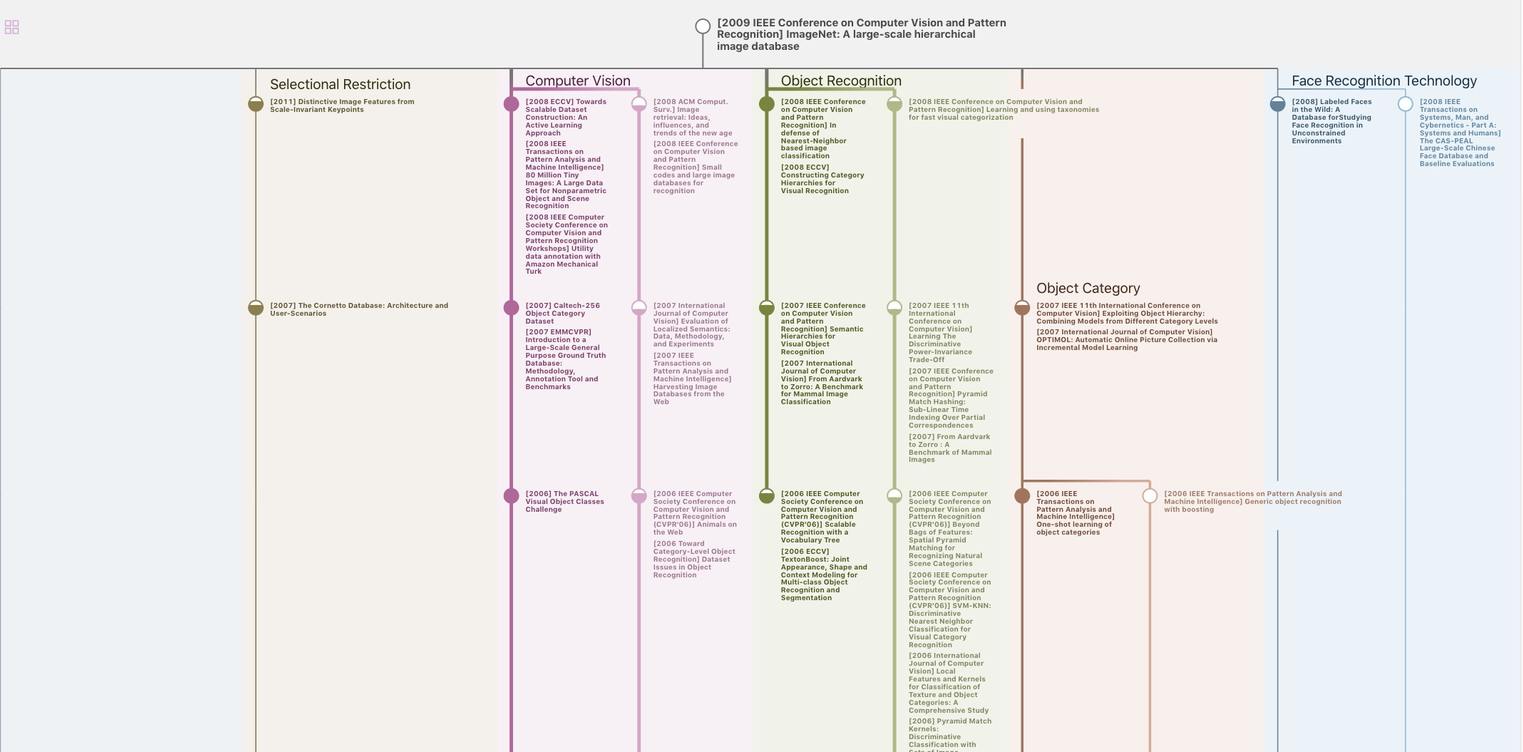
Generate MRT to find the research sequence of this paper
Chat Paper
Summary is being generated by the instructions you defined