Rationet: Ratio Prediction Network For Object Detection
SENSORS(2021)
摘要
In object detection of remote sensing images, anchor-free detectors often suffer from false boxes and sample imbalance, due to the use of single oriented features and the key point-based boxing strategy. This paper presents a simple and effective anchor-free approach-RatioNet with less parameters and higher accuracy for sensing images, which assigns all points in ground-truth boxes as positive samples to alleviate the problem of sample imbalance. In dealing with false boxes from single oriented features, global features of objects is investigated to build a novel regression to predict boxes by predicting width and height of objects and corresponding ratios of l_ratio and t_ratio, which reflect the location of objects. Besides, we introduce ratio-center to assign different weights to pixels, which successfully preserves high-quality boxes and effectively facilitates the performance. On the MS-COCO test-dev set, the proposed RatioNet achieves 49.7% AP.
更多查看译文
关键词
object detection, bounding box regression, high-quality box detection
AI 理解论文
溯源树
样例
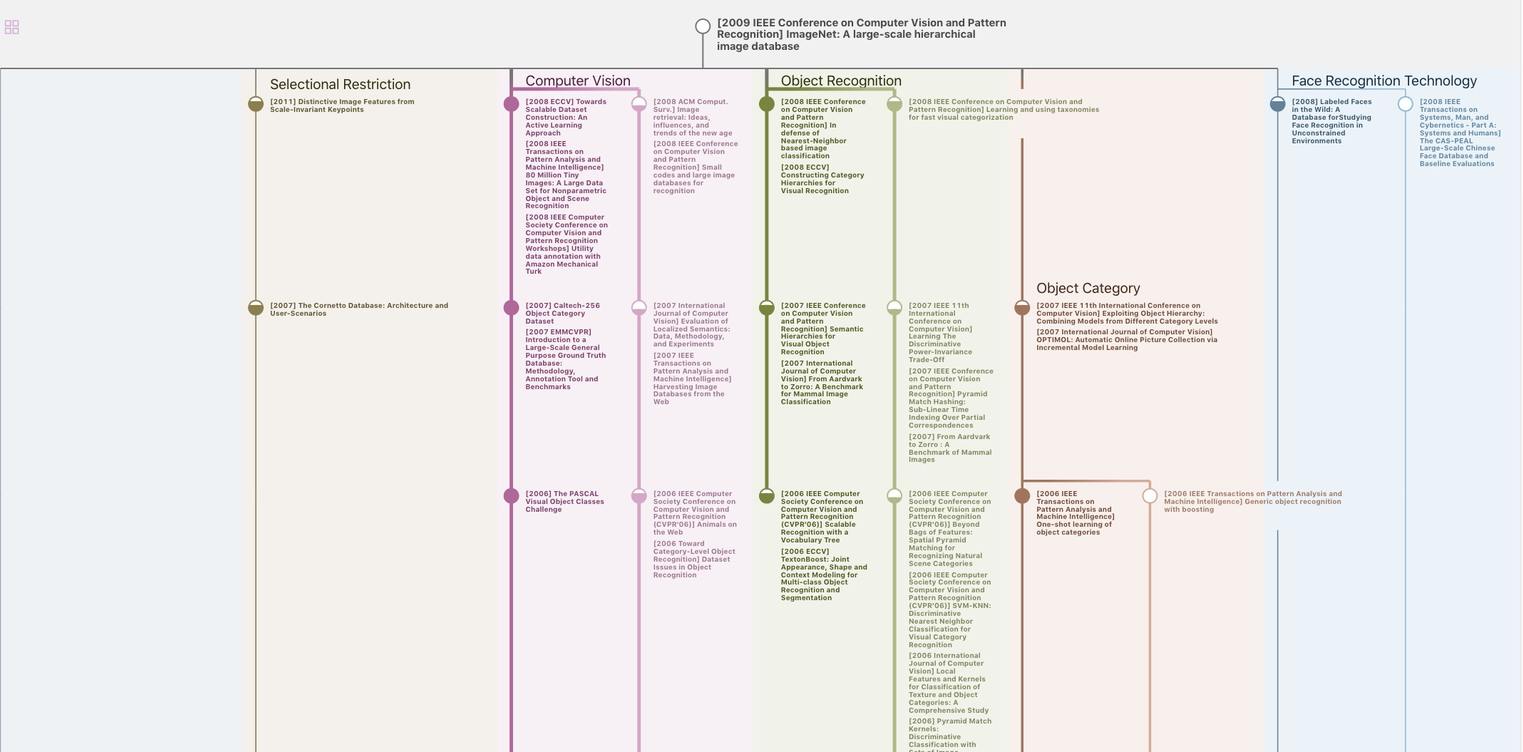
生成溯源树,研究论文发展脉络
Chat Paper
正在生成论文摘要