Integrate Candidate Answer Extraction With Re-Ranking For Chinese Machine Reading Comprehension
ENTROPY(2021)
摘要
Machine Reading Comprehension (MRC) research concerns how to endow machines with the ability to understand given passages and answer questions, which is a challenging problem in the field of natural language processing. To solve the Chinese MRC task efficiently, this paper proposes an Improved Extraction-based Reading Comprehension method with Answer Re-ranking (IERC-AR), consisting of a candidate answer extraction module and a re-ranking module. The candidate answer extraction module uses an improved pre-training language model, RoBERTa-WWM, to generate precise word representations, which can solve the problem of polysemy and is good for capturing Chinese word-level features. The re-ranking module re-evaluates candidate answers based on a self-attention mechanism, which can improve the accuracy of predicting answers. Traditional machine-reading methods generally integrate different modules into a pipeline system, which leads to re-encoding problems and inconsistent data distribution between the training and testing phases; therefore, this paper proposes an end-to-end model architecture for IERC-AR to reasonably integrate the candidate answer extraction and re-ranking modules. The experimental results on the Les MMRC dataset show that IERC-AR outperforms state-of-the-art MRC approaches.
更多查看译文
关键词
answer re-ranking, extraction-based machine reading comprehension, pre-training language model, self-attention
AI 理解论文
溯源树
样例
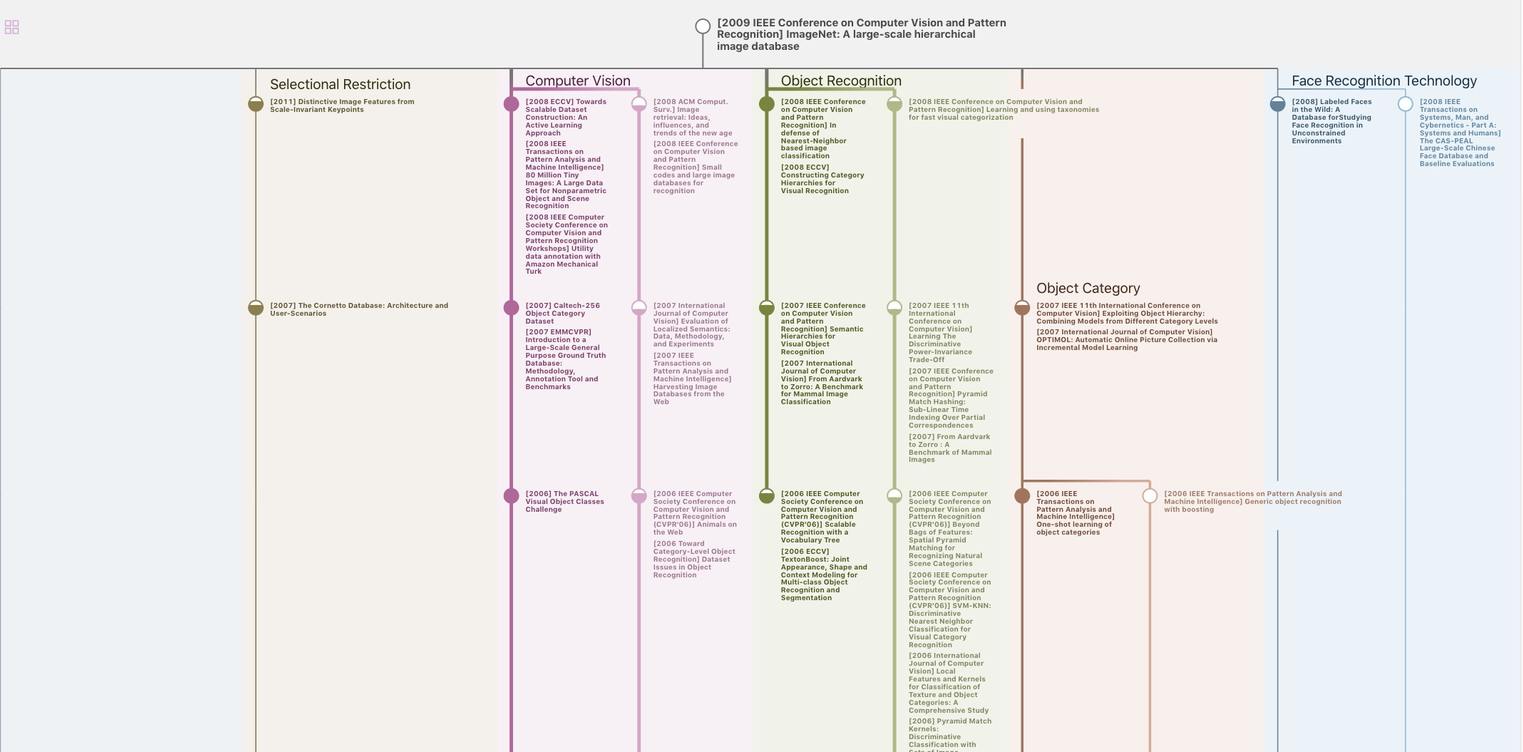
生成溯源树,研究论文发展脉络
Chat Paper
正在生成论文摘要